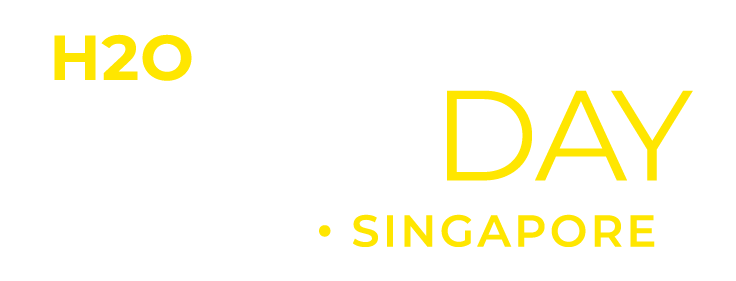
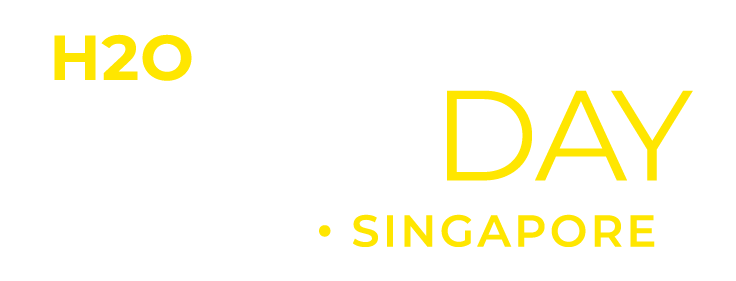
Singapore Fireside Chat 2023 | Agus Sudjianto and Sri Ambati
Speaker Bio
Sri Ambati | Founder & Chief Executive Officer
Sri Ambati is the founder and CEO of H2O.ai. A product visionary who has assembled world-class teams throughout his career, Sri founded H2O.ai in 2012 with a mission to democratize AI for anyone, anywhere, creating a movement of the world’s top data scientists, physicists, academics and technologists at more than 20,000 organizations worldwide. Sri also regularly partners with global business leaders to fund AI projects designed to solve compelling business, environmental and societal challenges. Most recently, Sri led the initiative o2forindia.org, sourcing O2 concentrators for more than 200 public health organizations in Tier 2 cities and rural communities in India during the Delta wave of the COVID-19 pandemic, helping to save thousands of lives. His strong “AI for Good” ethos for the responsible and fair use of AI to make the world a better place drives H2O.ai’s business model and corporate direction.
A sought-after speaker and thought-leader, Sri has presented at industry events including Ai4, Money2020, Red Hat Summit and more, is a frequent university guest speaker, and has been featured in publications including The Wall Street Journal, CNBC, IDG and the World Economic Forum and has been named a Datanami Person to Watch.
Before founding H2O.ai, Sri co-founded Platfora, a big data analytics company (acquired by Workday) and was director of engineering at DataStax and Azul Systems. His academic background includes sabbaticals focused on Theoretical Neuroscience at Stanford University and U.C. Berkeley, and he holds a master’s degree in Math and Computer Science from the University of Memphis.
Dr. Agus Sudjianto, Executive Vice President, Head of Corporate Model Risk, Wells Fargo
Agus Sudjianto is the Executive Vice President and Head of Model Risk at Wells Fargo, a role that includes chairing the Model Risk Committee and overseeing enterprise model risk management. His extensive career in the financial sector features roles at Lloyds Banking Group in the UK as the Modeling and Analytics Director and Chief Model Risk Officer, and at Bank of America as an executive leading Quantitative Risk. Sudjianto also has experience in the automotive industry, having been a Product Design Manager at Ford Motor Company.
An accomplished academic, he holds advanced degrees in engineering and management from Wayne State University and MIT. His expertise spans quantitative risk, credit risk modeling, machine learning, and computational statistics. Sudjianto is a prolific innovator with several U.S. patents in finance and engineering, and has made significant contributions to the field through his publications, including co-authoring "Design and Modeling for Computer Experiments." His work, particularly in interpretable machine learning models, is vital in regulated sectors like banking, and his patents cover a wide range from time series simulation to financial crime detection, highlighting his dedication to technological advancements in risk management.
Read the Full Transcript
00:18
It's a wonderful opportunity to be amongst fellow learners, both machine learners and both have some incredible content for the rest of the day. I know many of you are here to take hands -on training sessions after this on retrieval augmented to GenRTVI and GenRTVI at the LEMs.
00:43
Agus Sudjianto needs no special introduction. He's a lot of all the models in some of the largest banks in the world, almost every bank in the United States and the world follows some of the standards he's set for valuation and also perceiving the value of models is a strong following globally on kind of building designing models.
01:15
He's also a local. He's an extraordinary fruit of the seeds growing around from this region. Please welcome with warm welcome to Agus. Thank you, Sri. Thank you for the opportunities. Coming to this region is always exciting.
01:49
When I was planning to visit my team in Hong Kong and in India and then make a tour coming for a week in Indonesia to visit my mom. I was born and grew up in Indonesia. And three of us, okay, why don't we do a stu -o -jani, I will, in Singapore, okay, let's do it.
02:10
I'll make a detour to Singapore, always having fun in Singapore because it's incredible scenery and incredible foods, right? This place, if I look at foods, it's like the first thing when I arrive in Singapore, landed yesterday in the airport, I say, okay, the first thing I need to eat, I need to eat pakute, okay?
02:28
So that was my first stop. And then, okay, this is not enough. I need the next to the pakute stall in the airport was a young tofu place, okay? I need to eat that one too. And then in the evening, Sri made a reservation going to a Michelin star restaurant.
02:47
I said, Sri, we need to eat real food. So we need to find really real food, not Michelin star restaurant food, right? So we went to a Padang store, right? Padang, where we ate Padang food, right? In the Kanda Har street.
02:59
That was an incredible, incredible place. Great experience, right? I think talking about real food and real models, maybe it's a good, hard detour. What is in the day in the life of a bank, right? There are a lot of models that are being used.
03:19
Could you describe, set the landscape for what types of models? Right. A decision making by model becoming more and more important. For us in the bank, in the US, some of you may not be old enough. They experienced the crisis of 2008.
03:39
The crisis of 2008, the big recession, the great recession, the experience in 2008 when sky was falling. The blame was pointed to model. At that time was the Gaussian co -pollar. And last year and this year as well, we are in the US, it has a banking crisis.
04:06
You experience it, right? So Silicon Valley Bank went belly up. Part of it, they also get the blame on the asset liability management side of the model. So in bank, a lot of critical stuff done by model.
04:25
And AI is played a very, very critical role and a lot more important role. From credit decisioning, from fraud detection, from listening to customer when customer complain and how the complain are being handled.
04:38
Like if you an example, my complaint is so important. In the US, it has regulation. If you're not happy with your bank, you have problem, anything. You call the bank, right? Or you write in the bank.
04:49
the bank. That complaint has to be resolved by 10 days per regulation. If it's not resolved by 10 days, bank can get fined in penalty. So it's a big deal. In a company like Wells Fargo, when you have a lot of customers, you have 1 .5 million complaints per month.
05:08
1 .5 million complaints per month, every one of them have to be solved in 10 days. How would you do it? So we hire army of people. You hire army of people. These people are relatively in the low paid job and a bit boring looking reading and routing the right people.
05:27
So it's very difficult. You have very high turnover. Very high turnover, you have to train all the time. So that's when language model come to rescue. Now language model do the job. By the way, because you have to hire people train all the time, their accuracy of routing is only about 60 something percent.
05:46
If you route it wrongly, you will not solve it in 10 days. Right? Because wrong routing, you'll go somebody else. Okay? So language model has accuracy of 80 plus percent accuracy. So here it's different.
06:00
You don't have to retrain people. And this one becoming more and more coming. So the heart of language models is this incredible paper that came in 2017. Many of you remember, attention is all you need.
06:17
So now it's working remarkably well. Most of us are seeing the fruits of it. And we have some idea of why it works and some of it, which we don't know why it doesn't work. For example, for reasoning and others.
06:33
What is your intuition? Well, you know, all of you are familiar with neural networks, right? I'm assuming. Yeah? I love you family. And ReLU using ReLU activation function. A few years back, I wrote a paper on how to unwrap ReLU neural network.
06:55
What is it really? What ReLU neural network do? ReLU neural network is really local linear model. If the ReLU you need is turned on, it's linear. If it's thinks it's off, it's zero. Right? A combination of a lot of ReLU, combination of linear, still linear.
07:12
So ReLU network creating locally linear model. If you have, basically is A1X1 plus A2X2 plus A3X3 and so on and so forth. So local linear model like that. You can check that paper in H2O, implemented in H2O wave, the alaysia, right?
07:32
Very Sivam. Sivam, very Sivam. Sivam is the author of that. Put, unwrap the ReLU neural network so you know exactly all the local linear model. How many local linear model inside that? that you train.
07:47
We should also credit the agent on the team. Yeah, and the team, my team, so Siva worked with my team in Hong Kong to do this. So locally in your model, what attention, if you look at attention, this is the really important, and many of you that follow Kegel, we have several Kegel grandmasters in the traditional machine learning, in tabular data, gradient boosting machine is always win, neural network is a lacquer in this.
08:20
But why is this? In neural network is locally linear to get interaction, what we call it interaction in statistic x1 times x2. It's difficult representation in ReLU, you have to cut it into small region, and each of them will be approximated with linear model, because interaction is very difficult for neural network.
08:44
Gradient boosting machine, like 3 on the other hand, is very interaction. I split it with x1 and then I split it with x2. And then I split it with x3. So interaction is captured very fast by 3. So gradient boosting machine in tabular network all the time.
09:03
Now, here's the beauty of attention. Attention, if you remember, the basic attention, it has k, q, v, basically x, time x, time and other x. So note the interaction term is captured by attention, something that is missing.
09:22
Now you have feature representation as interaction. x1, time x2, x3, that's captured by attention because that's a structure. And this is very important in language because language is about context.
09:35
Word and word. And the context is not consecutive. In convolution, it has to be consecutive, it has to be neighborhood. Attention, you can jump. So interaction with any. This word here has context with 10 words later in the sentence.
09:52
So that's why attention works so well because it captures interaction across variables, something that is missing in the standard RELU network. It's really the feature engineering layer. Once you have the right feature, then you feed it to RELU.
10:08
That's why if you look at all the transformer, it has layer of attention to create high interaction. And then after that, you feed it to RELU network to the work. The feature of that, go to RELU network.
10:22
So that's why attention is really important in a breakthrough on this, is missing link in the traditional RELU network. Berlin. Embeddings. Yes. I want to touch on, so attention is all you need, but there's a contrary or even almost a complementary.
10:44
concept on embeddings and what some of our professors like Boyd would probably say embeddings is all you need. Embedding is a very interesting concept. If some of you are familiar with traditional etapolar data, you have variable, you transform it.
11:02
You transform it into using log transformation or quadratic transformation, that's embedding. Right? You embed it into that. Then you create a YouTube feature engineer, you create X1 times X2. So you embed it in higher dimension.
11:18
You embed it, you create features. Now, all supervised machine learning, including even including GPT, right? Because it's trained with a certain target. If you look at neural network, the final layer of neural network is linear model.
11:35
The key is the last layer. It's very good embedding. You transform your input into higher dimension, better domain, so that linear model, the last layer, works very, very well. If you look at gradient boosting machine, it built into three nodes, individual nodes of the three.
11:59
And the model is actually linear model of that terminal node. That's why if you go to Exy PUS, you can go to Exy PUS table, you can see the coefficient regression of each node. So all of this machine learning what it does is transforming from original variable into an embedding space, so that linear model works the best.
12:24
So embedding is very, very key. Now, with real language model, it's the same thing, right? All the language model, if you look at it, it's transformed into integer, and then go through all the attention layer for embedding, and then go to Raylou layer for another embedding.
12:42
And finally, it's a linear model to decision. So it's so important because embedding is the feature engineering. In the old days, we do feature engineering by hand thinking, all this thing, how to do feature engineering.
12:55
Now, with all this machinery, embedding is the feature space. It's the feature engineering, it's the key in machine learning. So that finally, linear model will work best, just like in neural network, final layer is linear model.
13:11
In gradient boosting, it's the same thing. You create it and then embed it in the zero one. The difference is in gradient boosting, the embedding is one -hot encoding, zero one, zero one, zero one, whether the data fall into that.
13:26
In neural network, the embedding is locally linear model. Amazing. Because back to the theme we started with, the interactions of... so many different cultures in Singapore actually makes it a very unique place, right?
13:43
All the different interactions. And, um, do, obviously for embeddings, in, if you're looking at Arabic or, or different languages, you need different embeddings. Um, for LLMs, language, um, makes a big difference.
14:00
What, where do you see the space moving, especially there's so many different languages to cover? Right. Yes. Um, embedding, if you think about it, embedding is the representation of the world. How you see the network model, take the embedding and then make decision of that.
14:17
Even, even what you're going to spend this afternoon in, in, in regs. It's like you have the embedding model, which translates from whatever domain into that domain, right? So it's a very, very important.
14:32
Thank you. Quality of embedding is very, very important. All this LLM, all this RAC application that you're going to build, the key is to get really, really good embedding. How to really get the good embedding is the architecture of your model, which is nowadays very standard because it's transformer.
14:54
So now the other part of that is the data. So the data is very important to get the real embedding because embedding really how you capture knowledge. And this is just like human being. Everybody learns differently.
15:10
So they capture the knowledge representation in the brain very differently. So just like large language model, we have so many of them, one will be better for another because the capture, the knowledge differently through embedding.
15:26
When you want to choose about which one to use as your embedding, as your LLM, because nowadays you have so many open source, which is wonderful. It's like how you choose your team. Like choosing your team members, hiring people, the thinking, the approach, the knowledge that they have.
15:49
Because they say, okay, what kind of things that you need? So choosing LLM is like choosing team that you're going to recruit, right? Which one? That's why in Arabic language and all those things, you need to have a specialized embedding.
16:02
It's the culture of LLM, right? Orman Society of Minds. So it was the Orman Minsky many years ago. So going back to choosing your team, it's a very interesting topic. I'm sure you get asked every time, I'm starting a new place, new bank, new fintech.
16:20
I need to build a whole or even entertainment media. You're going to start a whole data science team build. What is the kind of secret sauce? How many caps? So the Kaggle Grand Masters are good at competitions, then you have industry, deep industry domain expertise, you have the industrial data scientists, and then you have the professors from the university.
16:46
You happen to have a good channel. So Vijay and his team and what not. So maybe it's a good to give the audience an idea of how to build a team. I share with you a little bit, talking about team. In Wells Fargo we have several thousand of models that we manage to run every aspect of the bank.
17:11
And we have somewhat large team. We have 1500 people data scientists in our team, a thousand of them in the US, about 500 of them in India. And people for institutions like us, we have a, we recruit about 100 people.
17:31
people out of college every year. And we train them because we have the resource to do that. But most of you probably don't. So then how would you do it? So when we look at this, there are a few things, right?
17:47
When we do data science and all of this. It's really people that we need to put together people with various talents, different backgrounds, and to be able to find a unicorn, somebody that can do everything is hard.
18:01
So we have to find people that could in certain things, may not be good in other things. From business knowledge, because at the end of the day in data science and AI, we build business solutions. That's at the end of the day.
18:14
What's in it, what the business will get out of it. So the business knowledge is important and that's a very specific domain. And then you need to have good data scientists, people that can work on this.
18:27
And of course you also need machine learning engineer because you need to put this into production. So thinking about people that can put this thing into production, people that can model, that people can understand business.
18:40
So different scale that you have, different company, in a smaller company you have to do everything by yourself. Some of you probably do that. I have to do everything myself. Why can't I ask anybody?
18:53
In large company, like in my world they are very, very specialized people that dealing with data, that's not part of the 1500, they build models, people that build models, and maintain models that's different.
19:07
And then we have technology partner who really operationalize the infrastructure. So a really different kind of thing but need to think about how to put all of this knowledge together and depending on the scale of the company and what you do, you probably have to recruit people that can do them all.
19:24
May not be very expert in certain area but can do them all. And if you are bigger company, want to be specialist, then build people. people with deep knowledge are very specialized. So on the LLMs, how are you validating these LLMs differently than regular machine and LLMs?
19:44
Thank you for the question. I'll give you a little bit background on this in the US, especially in the financial institution. In the US, in financial institution it has called SR 11 .7. SR 11 .7 especially how to manage model risk.
20:01
Every model is wrong. This is the thing that is very important for people who build model. Be very humble, right? Because all this model will be wrong and it can be wrong and when they're wrong they can create harm either to the company or to the people that subject it to the user.
20:18
So we always very, very humble in terms of model and we need to really understand how the model will be wrong. So that is really the area of model validation. How can we test model rigorously? to understand where the model is weak.
20:34
So that's model validation. And model validation in principle, we really just like people that do information security, you want to hack this model, you want to find the vulnerability of model, in what situation, in what condition model will be wrong.
20:56
And in tabular data, you can do that very easily. And some of you that want to see some example, my team released tool called PyML, Python Interpretable Machine Learning. And as you can download and use it, in fact today they released a 0 .6 release that's fully compatible with H2O.
21:16
Every H2O model can be tested, can be validated with PyML. And so looking at few things, model weakness, which area that the model will be more wrong. If you look at AUC, you look at mean square error, it's not only just single number, but in what situation that the AUC will be bad.
21:35
So model weakness identification, you look at model robustness. If the input has noise different, is the model still going to perform? You look at resilience. Under different situation, is the model going to still work fine?
21:50
That's resilience. And then we talk about reliability. How reliable is the output? How uncertain is the output, the decision? So that's in tabular data we do that. Now in LLM, one of the key important on this, all of this is really explanation, try to understand what the model does.
22:09
That's where all the XAI, explainable AI, try to explain what the model do. Now in LLM, it's a little bit more challenging because you have a big black box. Explainability is a little bit more difficult to do.
22:23
Now explainability, if you talk about the embedding, is really understanding how good is the embedding. in what topics the embedding is done well, in what topics not done well. So you start from that embedding quality.
22:38
You have to see how the embedding quality. And then you look at all the principles that we talk about, model weakness and identification, and all of those things has to be done. For example, if you do for REC, which is going to do a lot this afternoon, right, Retrieval Augmented Generation, it has information retrieval site to develop to establish the context, that's you need to do precision recall and you need to identify what condition precision recall is not good.
23:07
Beyond just single measure of, which going to learn properly this afternoon. And then you also have to do robustness, how robust? Because in LLM, the difficult piece is a lot of data coming in when you train it.
23:23
When you test it, how do you know that this testing data is not part of the training? In Tafelo data, you can do it. You can test it, you can see it, but if you have a free -train model like in LLM, you don't know.
23:36
So it might be this testing data, actually it's not fair because it's already in training data. So that's the difficulty, right? So you have to be more creative, how to do it, how to make sure that the testing data is not in the training data.
23:51
Then you do something like a negation test. If you negate it, the answer is still correct. You do invariance test. If I alter it, if I shuffle the word, is it going to still working? If I change it into synonym, is it going to still working?
24:08
The dual -directionality test, if I make it becoming more, is it going to be more? So a lot of testing that you have to do to make sure that the model will generalize well when you put it in production.
24:22
So the conceptual soneness of an LLM is a new territory? Yes. The model conceptual soneness of sound, this model, is really how sound or how good the embedding is. Small number of large models or large number of small models?
24:44
So three question is, do we want one model with 100 billion parameter? Or 100 model with 1 billion parameter each you use? So I think this is going to be a still continuous debate, but if it really depends on my understanding.
25:08
In real world, in real world, we don't build model for general purpose, like chat GPT or GPT4. They try to build foundation model for large general purpose. It can do math, it can do a practical question, it can do all of those.
25:29
So it becomes generalist. Check of all trade, master of none. So just like people, somebody will be in... But you showed me a paper yesterday where it was that basically the GPT -4 is beating the medical specialized met plan models.
25:52
I think it really depends on my opinion. So let me go back a little bit and I'll answer that then. And then when you also do testing, this is the problem with LLM testing today. People make a claim, oh this one better than the other, this one better than the other, better than the other.
26:15
I don't believe any of those. The reason is, better one than the other based on your testing data, how you test it. And the testing data, you can always craft it. in a way that this will be better than the other.
26:34
So it's really testing data dependent to make that claim. At the end of the day, it has to be your own problem. With your own problem, you test it whether this is good or not. Because even testing that I command all the sting people that try to do, Helm, that Stanford put, right?
26:57
It's right to test it for all kind of capability. Oh, it's nice. But for real application, is that the test that you want to do? No! Because it's specific domain, your own data. So all of those is good to know, but it's meaningless.
27:16
So we have to test it with your own things. Now, if it's really small subject, narrow application that you do, you're not trying to use your model to be able to answer a gymnastic question or swimming question, then you don't need the big model.
27:36
You need very small specialized model. That's all you need. Specialized model that you do. And even if you do rec, which you're going to learn a lot today, Retrieval Augmented Generation, the data is your own data, right?
27:50
Your document. So retrieve that document. So you need small embedding for embedding model. You don't need big model for that. And then you retrieve the information. Once you retrieve the information, you send it to large language model to do summarization.
28:08
Right? You get this data from your own document, retrieve from your document, and then you summarize it. You only need, depending on the language that you want to use, you only use large language model to write, to do English, to summarize from the knowledge of this.
28:24
If you just try to do writing better, writing English better, I think anything bigger than 3 billion you don't urge. will do a decent job. A 7 billion parameter model like Lama 7 billion will do a very, very decent job.
28:39
You don't really need a 100 billion parameter model. So that's from practical at the reason. We like the MISTRAL 7B. It has a very good attention mechanism, overlapping windows. So it gives a very reasonably long context as well.
28:53
So 7B has been doing really well for us open source MISTRALs. But I think there is a thought that even the largest models are actually a combination ensemble of 20 billion or 30 billion models. I think this is what we learn as well from the traditional machine learning.
29:11
If we have a few KGG Grandmaster in the room, the Wienerty Berkeley is really stacking, model stacking. You stack multiple models. Different model you stack you average them you're going to be you're going to be the winner you stack multiple model It's model to different thing.
29:28
They all Relatively accurate, but they are different model. They work very well. So I I think as this field mature I think my hands We going to be the same thing small model you're going to have mixture of expert I heard that actually GPT -4 is a mixture of expert to it's not a large model But combination of a small model created a mixture of expert which is model stacking work very well So that's when come back to the okay 100 You probably need a certain threshold so that the English contacts or whatever language contact can be captured Well, probably 3 billion parameter model.
30:05
We have a hundred of them probably better than 300 300 billion parameter model we Probably one of the last of the few questions Retrieval augmented we talked about by the way eval studio Shortout for that.
30:20
You'll see it's a very powerful way to connect value at your models continuously today will Probably train you on some of the evaluations that you can use the LGBT or eval studios One of the things that I mean a goose voice is so well respected by the SEC by the regulators and others in the US That sometimes some of the models we want to build are going to try to mimic your accent So that here it's very natural accent, right?
30:49
It's not so natural in the US. So in fact kind of a that bent of the Indonesian English Saudi station English Models, when do you fine -tune models and when do you want to use rag? Well, I would say the first Approach it should be if rack and do the job do rack right because that's the easiest way to do Right and use a you don't have to you don't have to train models.
31:26
So if you can use REC, that will be the first option. But sometime in a specific domain, it has very, very, make very difficult differentiation in the most certain domain. Like, we use our large language model for model documentation, for documenting model, documenting model validation.
31:47
So the context is very specific. And if we use language model that is not trained specifically, it's difficult to find a small nuance, the difference in small nuance. So if you have problem that small nuance make big difference in term of decision, then you have to train specifically, fine tune your model.
32:15
But if not, then... I would start with REC because that's a lot of tool out there. The H2O has this tool that's turnkey, right? Easy to use on the REC. And most industry application today is all REC today.
32:36
In banking, in a big banking and all those things. What kind of use cases? Maybe you can touch a little on the use case. Yeah, yeah. It's a use case in a bank. We all have thousands, millions of documents, millions of documents.
32:56
Finding information. For example, I give a few examples. Finding what rule, what regulation are applicable in certain jurisdiction. For us in the US where every state has different regulations, there's all kind of different regulators as well.
33:15
Finding what is applicable in my area. So we have a lot of policies, thousands of policies. It used to be a lot of Q &A. Very difficult to find information. Now it's very easy to find. You ask questions, you have Q &A, people can get the information.
33:29
We use it to help customers to have questions. Remember the person that do customer service, they are not really the very well paid. So you have item over. So you have language model that can help do a REC, information retrieval to help that.
33:47
You do sales pits or making some kind of pits to your clients on the commercial bank side. So it's very helpful to do something like that. So myriad application that you can do because we are finding information in the company is very, very difficult.
34:06
And the best way to do this is really that's the REC application. Probably the final question for regulating AI. There's a lot of dooms. AI doomers, we are AI tech optimistic and the extreme side, which we think the world needs so much help that AI can be a force for good.
34:29
What are the perspectives you're hearing? Yeah, the problem with all this regulation are really a lot of self -interest of various groups try to lobby, that's in my view. I hope the US will go to the right side and if you go to the lobby, you're going to create rules, it's a lot of lobbyists and lobbyists here are paid by somebody and who has the money to pay?
35:05
Really the big corporation that have a lot of interest to protect themselves. So I hope the regulation will not pick a winner. Microsoft will be the winner, Google will be the winner, and the rest are the losers, especially the open source, because the open source doesn't have a lobbyist.
35:25
That's the biggest worry that I have. That will be a terrible thing. In my opinion, AI, algorithms, large language model, it should not be regulated. What should be regulated is the layer of application.
35:41
You apply it for certain purpose. That needs to be regulated, because that's where the danger is. So the application layer needs to be regulated. The large language model itself should not be regulated.
35:55
That's my view, because the damage, the harm to the society, the misinformation, or whatever things are the application layer, that needs to be regulated. Well, AI is math, right? Math has been handed to us for generations, centuries of innovation, and math has incredible transformative power, and I think it needs to be in the hands of the people.
36:23
For us, open source, you mentioned interestingly, we don't use the word lobbyist, but the real love for open source comes from the community. We did not announce this event longer than a couple of weeks, but look at the audience, they're all here.
36:40
It's a global movement of open source enthusiasts, makers, creators, doers. They are voting with their hearts and minds and using the platforms. So I think open source has the people on its side. I think that's what we have experienced with our own journey at H2O.
37:06
So we are super strongest believers and supporters of open source, so the models, come in the hands of Organizations and the people every purpose needs a larger language model. Yeah, and if it's a good purpose it will get enough audience and enough momentum and The world is is an oyster to change at this point.
37:30
So here is an incredible time frame to be in AI So welcome to Hitchcock's in AI world That's just an incredible I think we're very exciting I want to do last word here three of my may because it's very exciting time for you.
37:45
I I was born 25 years too early in this world I When I have my PhD in machine learning in neural network was 1995 So when I graduated my PhD in machine learning, I cannot find a job In machine learning so I have to design a real machine right three So I you add I came I become I design car engine for Ford Motor Company because no such job of machine learning.
38:15
So I felt like I was born 25 years too early and now my daughter studied at Carnegie Mellon, studying AI. Man, what a wonderful world for many of you to be at this time. So I think you should cherish it.
38:30
I want to say one thing, building AI model becoming a lot more easier and become very easy today. Testing this model is not easy. So you need to pay attention on the testing, not only on the building and then when you do testing, be humble and be honest because if not, it will bite you.
38:53
So I think it's the skill of to be able to test not only to build model or providing application is super, super important. It's an inspiring journey. I mean from the beginnings here in Yogyakarta to all the way till everywhere in the world.
39:16
I mean in terms of parting words for the audience, where do they go from here? I spoke about this, it's exciting time build model but build it in a very responsible way. Thinking about what harm, what potential harm that this model can do because this model can become very discriminatory to people, to people with different socioeconomic level in all of these things.
39:47
So don't build model, don't be evil. To use this model, don't be evil. And it's very, very important thing about your grandma approve this. You build an application like this. Super important. Fabulous.
40:06
I know there's a roomful of questions and interactions. that are waiting to happen. Agus will be here sometime before lunch for sure. Definitely meet him and get inspired like we do. We're really fortunate to have the likes of Agus on the side of the open source community.
40:31
Thank you. Thank you. I think it's having a big audience with the technical background. It's always entertaining for me. Always very energizing because I thought, three, my job is doing management entertainment.
40:43
I get paid to do management entertainment, talk to senior manager, senior manager all the time. So dealing with technical people is always my excitement because I still write paper and write code. So thank you so much.
40:55
Thank you.