H2O Feature Store
Infusing data with intelligence
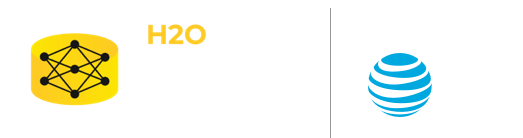
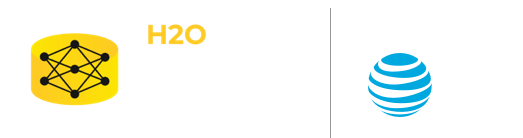
Valuable intelligence is often buried inside large volumes of data, and in today’s modern organizations, data is created and stored across many different technology stacks and business applications. Data permeates all organizational functions, and therefore, requires consistent collaboration between technical teams and their business stakeholders. Features stores are the key to effortlessly extracting and managing the relevant information within your data that is needed to build and deploy high-performance machine learning models.
What is a Feature Store?
Features are the independent variables that exist within a given dataset. Those variables must be converted into a format that can be used by machine learning models in order to provide some large-scale analysis or predictions surrounding a specific use case. The grouping of these key variables then creates a feature set which serves as the training data for a machine learning model. This process of feature engineering is often time consuming and artisanal based on the knowledge and experience of the data scientist.
Feature stores address the complexity of data quality requirements by providing a central repository that connects information across disparate systems to bring all of an organization’s data assets together in one place. It also enables the organization to re-use all of their data and feature engineering efforts across multiple use-cases. This simplifies the ability to house, access, manage and share features across machine learning projects, providing profound improvements in efficiency, governance and reliability across all AI initiatives.
The H2O Feature Store
Intelligently extracts, manages and optimizes the feature engineering and model building process by seamlessly connecting information across platforms, establishing quality, consistency and transparency throughout the machine learning lifecycle and ultimately bringing together the diversity of skill sets needed to deliver transformational value with AI.
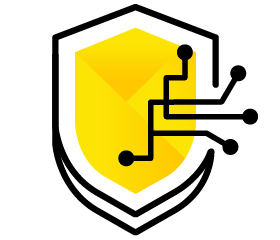
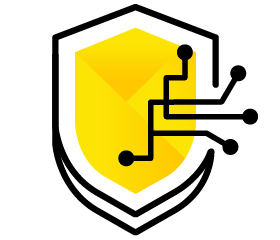
Provide security and governance
Automate data versioning and tracking of lineage provides transparency across the entire machine learning workflow to ensure compliance with company policies and government regulations. Embedded encryption methodology provides masking across data and models at an enterprise level, allowing for features and models to be used in production while maintaining adherence to privacy requirements and ethical standards.
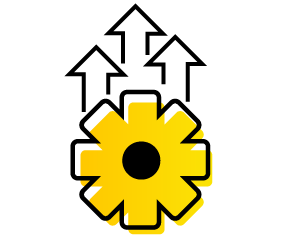
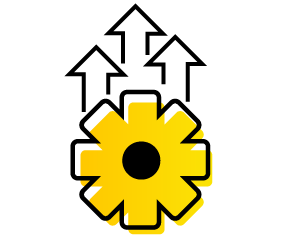
Improve operational efficiency
Reduce duplication of ingestion, storage and computational efforts with an API integration that optimizes feature management and model deployment without the need to re-code machine learning pipelines. Receive proactive recommendations, generate new features and easily discover related features with metadata tagging.
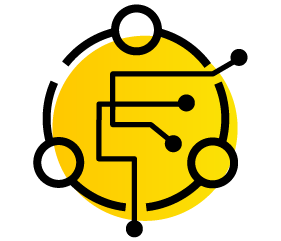
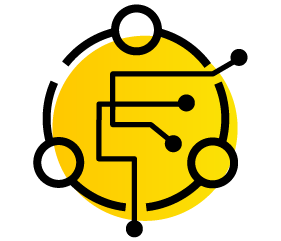
Promote AI collaboration
Improve access to and sharing of features across all machine learning pipelines, extending their usability across projects, teams and partners. By establishing a single source of truth for data, teams can access the most relevant information feeding predictive models and quickly employ them for newer problems while also maintaining close alignment between data science teams and their business unit counterparts.
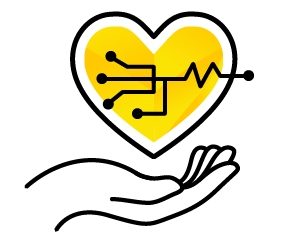
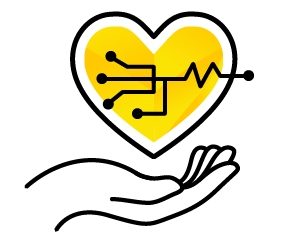
Ensure accuracy and reliability
Validate models with confidence through backtesting and time travel to ensure accuracy. Establish trust with visibility and credibility throughout feature development and model deployment to include automated bias identification and drift detection. Create superior feature sets with integrated human feedback loops that ensure optimal model production and deliver incredible model lift. This enables faster and more accurate response times to changes in model performance.
Data scientists then optimize the data with feature engineering, which is used to extract the most signals from a given dataset to drive machine learning models. Centralized access and control across the feature engineering and model building process means quality and consistency are tracked and maintained across feature sets.
The feature store uses a smart matching engine to proactively recommend high quality, already engineered features to use based on the unique data and business case a user is working on.
Machine learning algorithms then actively monitor and provide alerts about data quality and drift to ensure users are aware when model performance might be impacted in any way.
Data can be accessed in batch, in stream, or on-demand as required. Data and machine learning engineers can also configure H2O Feature Store to perform data quality tasks and maintain version control over those tasks, to ensure data scientists and business users alike are accessing the best quality version of the data needed to drive value.
Business users can gain insight into which pieces of information are influencing predictions, the importance of specific features in driving those predictions, and, using subject matter expertise, they can adjust the information being used and how it is being interpreted by the models surfacing those insights.
Accelerate Innovation with AI
Data permeates all organizational functions, and therefore, requires consistent collaboration between technical teams and their business stakeholders. With a unified view of all the data powering their AI initiatives, leaders can cohesively guide their organizations through the AI maturity journey, while reducing computational spend, increasing data science productivity, optimizing performance across all models in production and maintaining governance and security standards at scale. The H2O Feature Store serves as the nucleus to machine learning operations, and with artificial intelligence powering that nucleus, teams can innovate together faster than ever before.
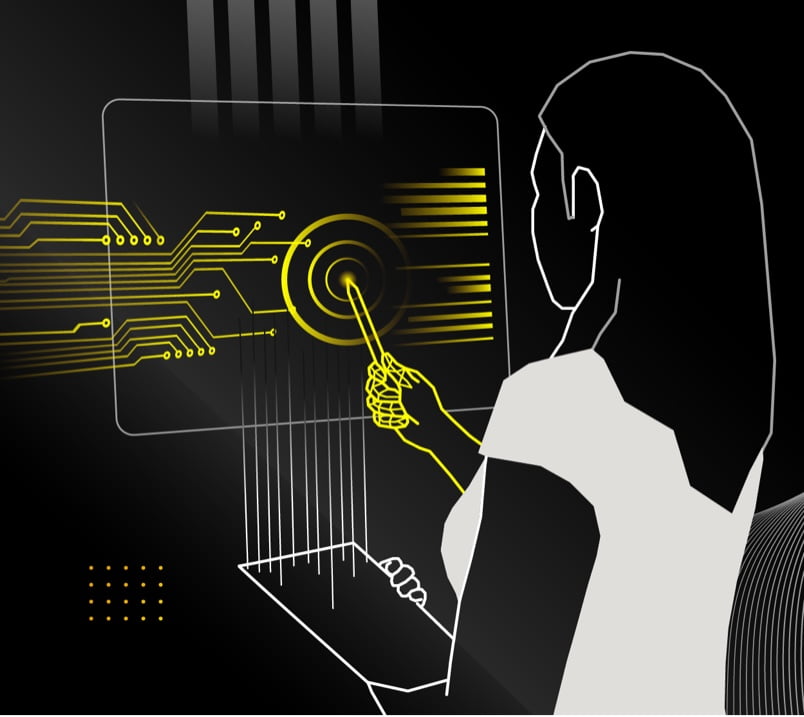
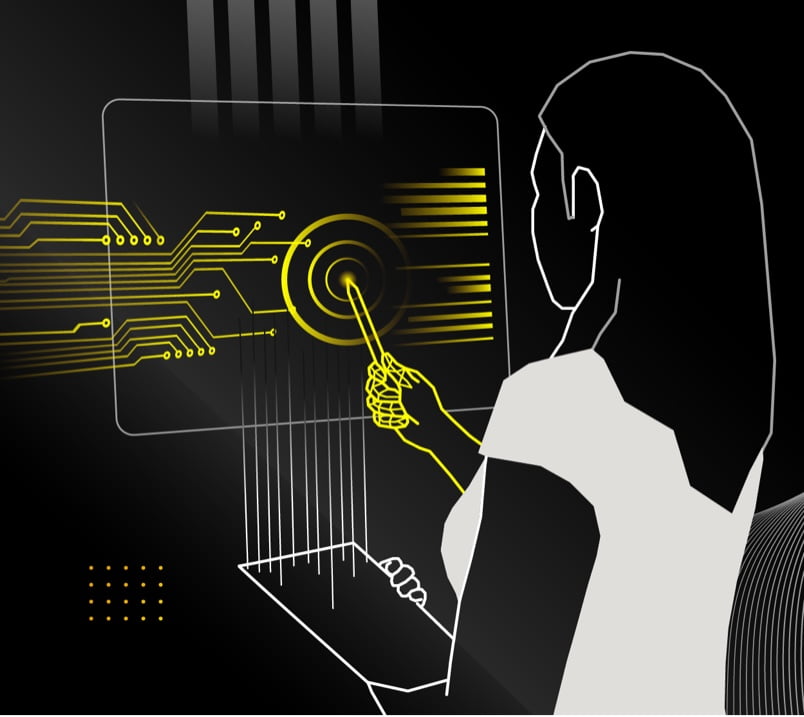
Data and ML Engineers
Streamline data quality management across machine learning pipelines.
Data and machine learning engineers are tasked with ensuring access and maintaining quality across all data systems. The process of ingesting, storing, and computing substantial amounts of data quickly becomes repetitive and time consuming without a systematic approach to manage data quality and machine learning distribution at scale. The H2O Feature Store allows engineers to streamline data quality management across all machine learning pipelines, reducing the time spent on repetitive tasks and compute costs, as well as improving the ability to provide consistent, quality data across company AI and analytics projects.
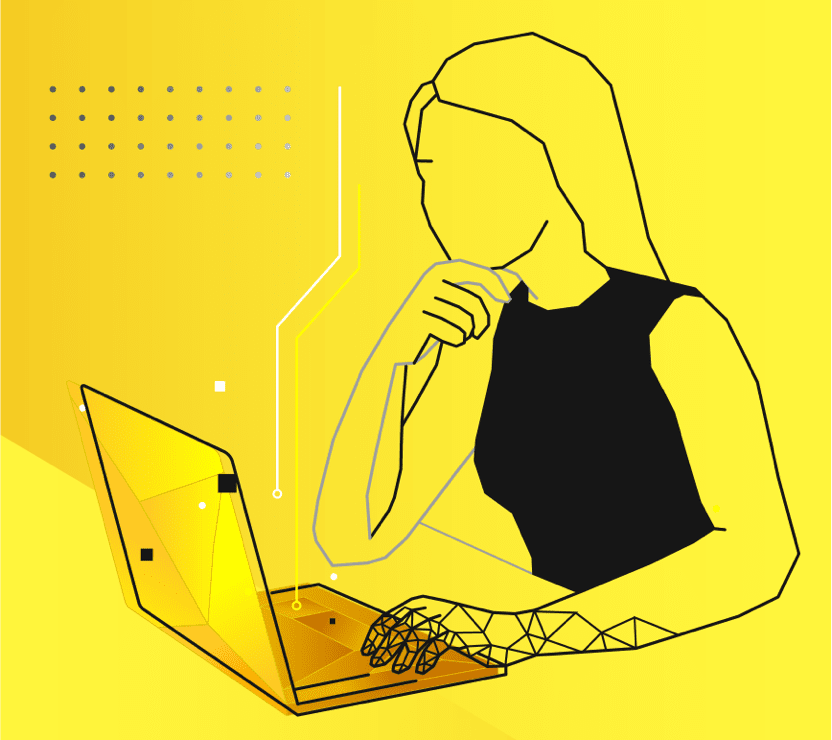
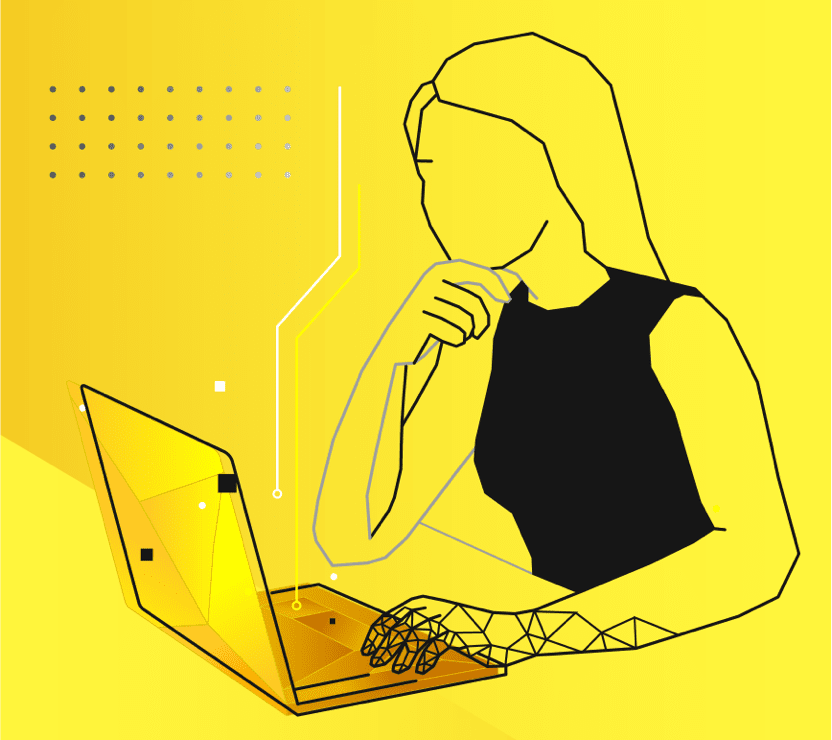
Data Scientists
Optimize model production and performance for all data science projects.
Data scientists explore data to create features that feed new predictive models or improve existing ones. When new data comes in, data scientists have traditionally been required to recompute the entire machine learning pipeline, often creating redundancy and inconsistency in the manual coding of the same features multiple times. The H2O Feature Store allows data scientists to optimize model production and performance for all data science projects by storing and searching through existing features, highlighting the newest, most innovative feature sets and accelerating the timeline in which a new machine learning model can be built and deployed.
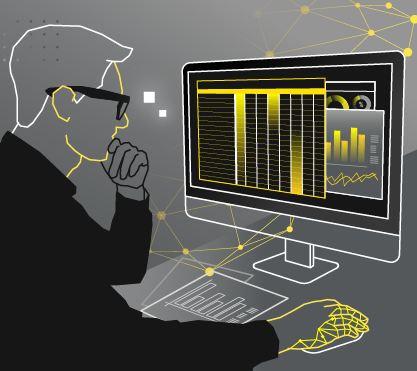
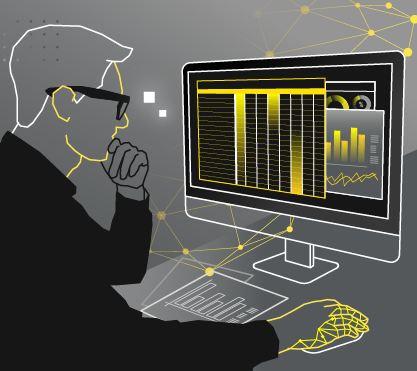
Business Analysts
Advance key objectives with collaborative analytics tailored to address specific use cases.
Business analysts and citizen data scientists possess a very clear understanding of the data within their respective domains, and serve as the data stewards for their business unit initiatives. Typically, without the skill set to perform data engineering or data science activities, business analysts need help with data wrangling and model development. With the H2O Feature Store, business analysts can easily see which features are influencing key use cases and models without the need to write any code. The ability to drag and drop various features into a visualization tool makes it simple to apply filters and aggregations to support the development of business reports and insights.
Feature Store in Action
AT&T carries more than 465 petabytes of data traffic across their global network on an average day, and turning data into actionable intelligence as quickly as possible is vital to their success. AT&T, co-creating with H2O.ai, is putting the final touches on the H2O Feature Store internally to reliably and securely handle large-scale and real-time production workloads.
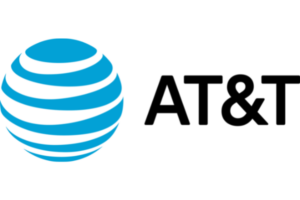
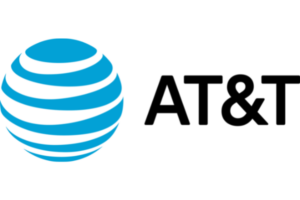
We’ve built our internal AI-as-a-Service (AIaaS) platform leveraging H2O’s AI Cloud services, and we believe the co-invention and co-development of H2O AI Feature Store is going to be one of the most impactful elements in our platform. With the H2O AI Feature Store, we’re building AI solutions that are much faster, more accurate and robust in a fraction of the time.”
Prince Paulraj, AVP of Data Science
Contact us to learn how the H2O Feature Store can help infuse intelligence across all of your data systems.