TELECOM
Transforming Call Center Operations:
World’s leading telco cuts costs by 70% by finetuning H2O small language models at the edge
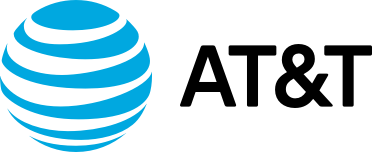
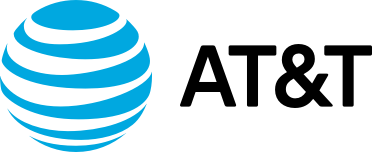
75%
latency improvement
Faster time to value
500%
scalability
Business velocity
70%
cost reduction
Higher ROI
AT&T, a renowned broadband connectivity provider, chose H2O.ai as its AI technology partner to drive business transformation across multiple use cases. One key area of focus is optimizing call center operations and elevating customer experience.
The company receives 5 million customer calls annually, resulting in a vast amount of recorded, transcribed, and summarized interactions. To unlock the value of these conversations, AT&T is leveraging the power of AI and language models to improve customer service and support, enhance customer experience, increase operational efficiency, and inform business strategies with data-driven insights.
Solution powered by H2O.ai’s Gen AI
In order to reduce computational costs and improve scalability, AT&T decided to distill Large Language Models, such as GPT-4, into three smaller fine-tuned open source models. This approach enables AT&T to easily deploy AI-powered language models in a production environment, driving business value with proven ROI.
For 20 key categories in their 80 multi-label classification system, they leveraged Danube 1.8B, a cutting-edge small language model fine-tuned with H2O LLM Studio. This enabled AT&T to extract actionable insights from customer interactions (ex: customers scheduled and technicians never showed up).
Business Value
The SLMs ensemble was able to get 91% accuracy, which is very close to the previous and much more expensive solution. Also, because they took 10 categories from Llama, 20 from Danube, and 50 from the classifier, they achieved 35% cost relative to the previous solution, with Danube representing 10%.


Results
The new approach built upon Small Language Models yielded significant improvements, such as a substantial reduction in processing time, increased transcript processing capacity, and reduced costs.
Why Small Language Models and H2O.ai?
Small Language Models (SLMs) are revolutionizing the way we approach AI-powered applications. They are more compact, nimble, and take significantly fewer resources to deploy when compared to Large Language Models. Additionally, SLMs are great at handling specific tasks and can be tailored to perform well within a narrower scope.
The H2O Danube series consists of compact foundational models, each containing 1.8 billion parameters. These models are designed to be lightweight and quick to respond, making them suitable for a broad array of use cases, including: retrieval augmented generation, open-ended text generation, brainstorming, summarization, table creation, data formatting, paraphrasing, extraction, chat, and more.
H2O LLM Studio, in turn, comes into play when a customer needs a custom solution. Created by our top Kaggle Grandmasters, this no-code fine-tuning framework empowers organizations to build their own state-of-the-art Large Language Models for enterprise applications.