Overcoming Obstacles to Machine Learning Adoption
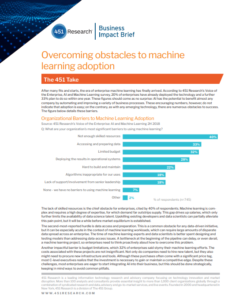
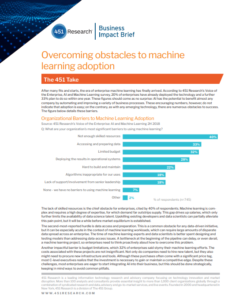
The 451 Take
After many fits and starts, the era of enterprise machine learning has finally arrived. According to 451 Research’s Voice of the Enterprise, AI and Machine Learning survey, 20% of enterprises have already deployed the technology and a further 33% plan to do so within one year. These figures should come as no surprise: AI has the potential to benefit almost any company by automating and improving a variety of business processes. These encouraging numbers, however, do not indicate that adoption is easy; on the contrary, as with any emerging technology, there are numerous obstacles to success. The figure below details these barriers.
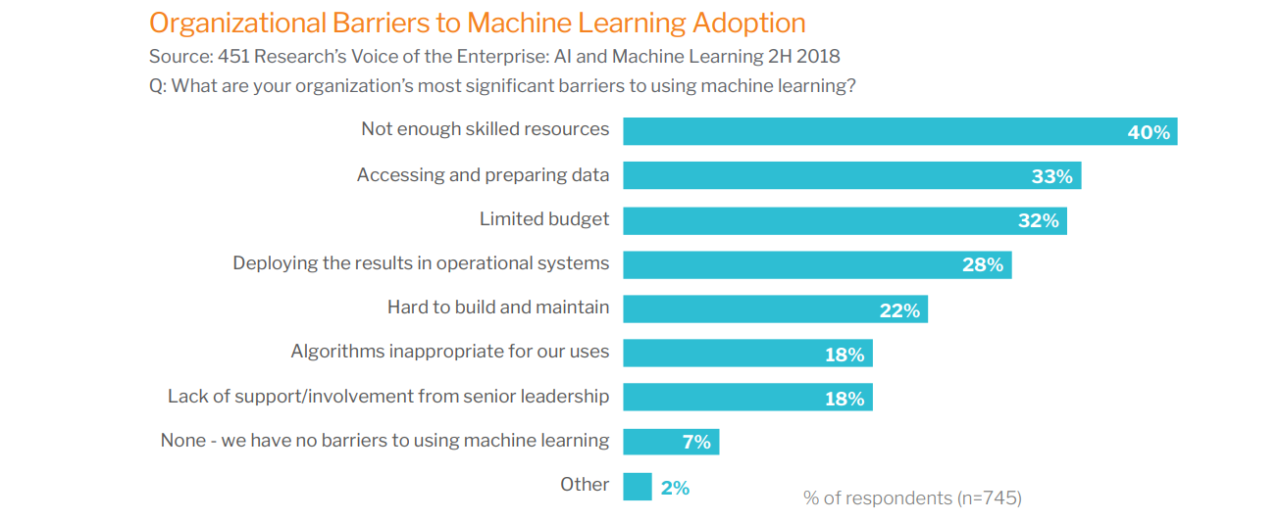
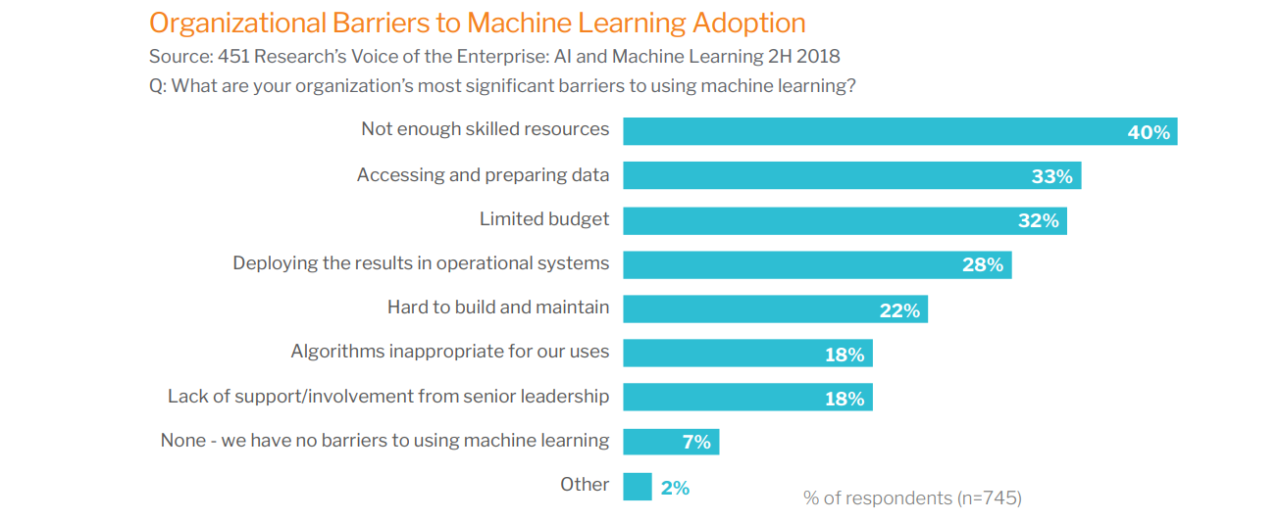
The lack of skilled resources is the chief obstacle for enterprises, cited by 40% of respondents. Machine learning is complex and requires a high degree of expertise, for which demand far outstrips supply. This gap drives up salaries, which only further limits the availability of data science talent. Upskilling existing developers and data scientists can partially alleviate this pain point, but it will be a while before market equilibrium is established.
The second-most-reported hurdle is data access and preparation. This is a common obstacle for any data-driven initiative, but it can be especially acute in the context of machine learning workloads, which can require large amounts of disparate data spread across an enterprise. The time of machine learning experts and data scientists is better spent designing and testing models than addressing data-access issues. A bottleneck at the beginning of the pipeline can delay, or even derail, a machine learning project, so enterprises need to think proactively about how to overcome this problem.
Another impactful barrier is budget limitations, which 32% of enterprises said stymy their machine learning efforts. The costs associated with these projects are not insignificant. Not only do companies need to hire new talent, but they also might need to procure new infrastructure and tools. Although these purchases often come with a significant price tag, most C-level executives realize that the investment is necessary to gain or maintain a competitive edge. Despite these challenges, most enterprises are eager to start integrating AI into their business, but this should be done strategically, keeping in mind ways to avoid common pitfalls.
Business Impact
ENTERPRISES SHOULD LOOK TO TOOLS THAT DEMOCRATIZE MACHINE LEARNING. The scarcity of machine learning expertise means that most companies may not be able to hire the talent for these projects. However, enterprises can supplement human resources with vendor-provided automated machine learning products that abstract away the complexity of machine learning, allowing developers, data engineers, data scientists and savvy business analysts to reap the benefits of the technology and to act as a multiplier for their team.
ENTERPRISES NEED TO PRIORITIZE TIME TO INSIGHT. Machine learning projects are often unwieldy, so leaders need to maintain focus to ensure they deliver value. Enterprises should consider machine learning products that are compatible with existing big-data infrastructure, contain model-scoring features and provide model-deployment functionality. These capabilities accelerate the machine learning development process and help promote successful outcomes.
ENTERPRISES REQUIRE OPTIONS THAT DON’T BREAK THE BANK. Between new hires and infrastructure investments, machine learning projects can be expensive initially. Luckily, vendor-provided tools can mitigate costs by scaling existing human and compute resources. Although machine learning will still require an investment on behalf of the enterprise, spending this money wisely will pay dividends in the long run.
Looking Ahead
Despite the hurdles, machine learning seems poised to make further inroads in the organization – the era of ubiquitous enterprise AI is right around the corner. Consequently, the problems outlined above – the paucity of skilled resources, data accessibility and budget restrictions – will likely continue to impact enterprises, at least in the near term. Luckily, a healthy ecosystem of vendors has emerged to address these common issues, making the adoption of machine learning feasible for almost any organization.
One approach provided by these vendors is automated machine learning, a technique that automates many of the steps in the machine learning process. As vendors continue to improve and evolve automated machine learning, these products should be able to address not only today’s biggest barriers but also those on the horizon. For example, an emerging obstacle for enterprises is the explainability of black-box decision-making by machine learning models. Companies need to understand the inner workings of this technology to root out instances of algorithmic or data bias. Already a number of vendors have begun to roll out interpretive capabilities that allow enterprises to extensively test, understand and explain models before deployment.
Given the transformative potential of enterprise machine learning, every company should be thinking about its AI strategy, if it hasn’t already developed one. The most successful adopters will be those enterprises that consider the predominant barriers to machine learning and address them proactively.