ENTERPRISE GENERATIVE AI
Model Validation
Enterprise h2oGPTe provides an evaluation framework for testing models in context-specific scenarios to ensure relevance, address domain-specific risks, and avoid misleading conclusions.
Metrics are tailored to capture critical outcomes, benchmark real-world workflows, and ensure transparency for compliance, risk mitigation, and user confidence.
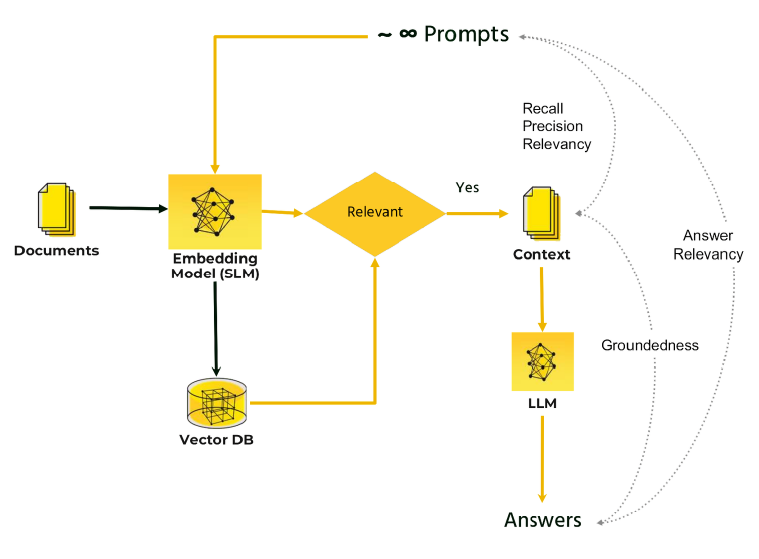
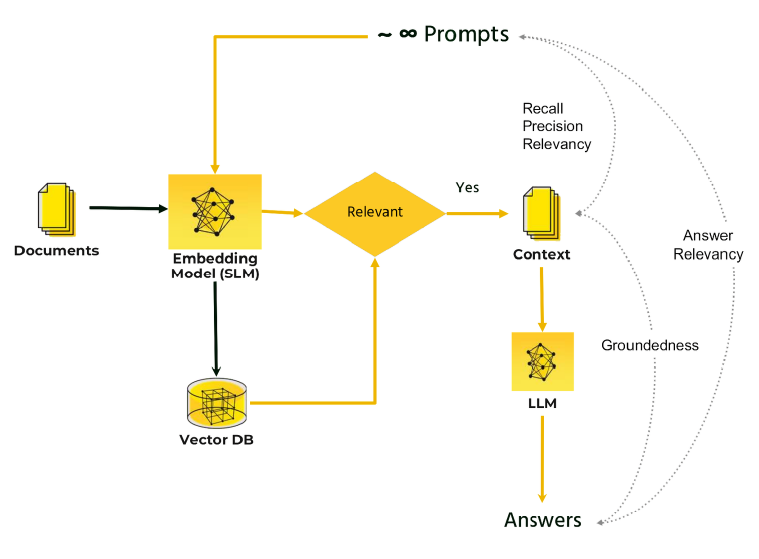
RAG evaluation
h2oGPTe evaluates RAG systems to assess retrieval accuracy, groundedness, completeness, and relevancy of responses. Safety metrics evaluate the system's adherence to ethical guidelines by preventing harmful, biased, or privacy-violating content.
Practices include layered filtering, human-in-the-loop reviews, and explainability tools for toxicity detection, alongside counterfactual analysis and sentiment evaluation for bias. Privacy protection uses techniques like NER and adversarial testing to mitigate sensitive data leakage, ensuring compliance with ethical and regulatory standards.
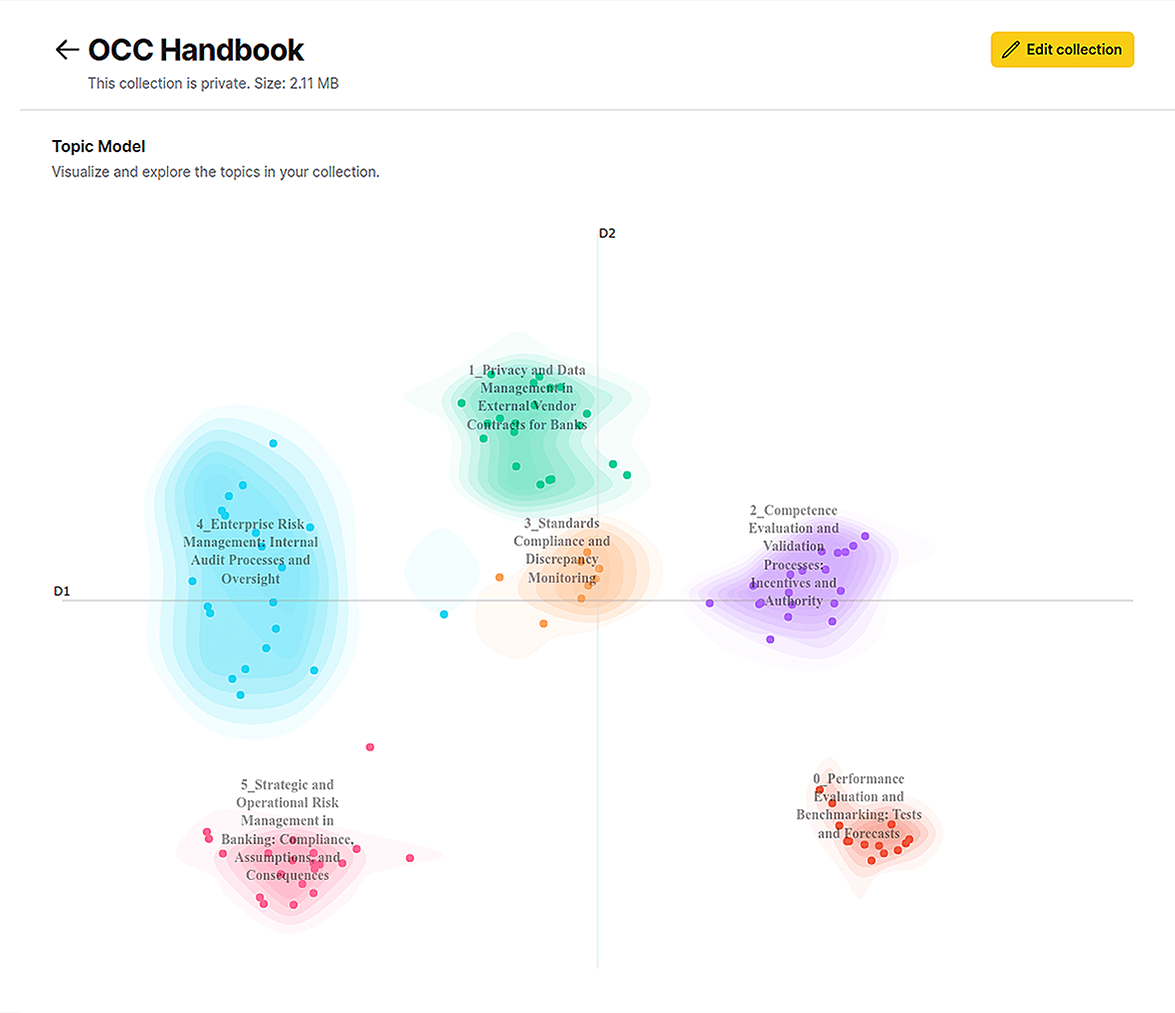
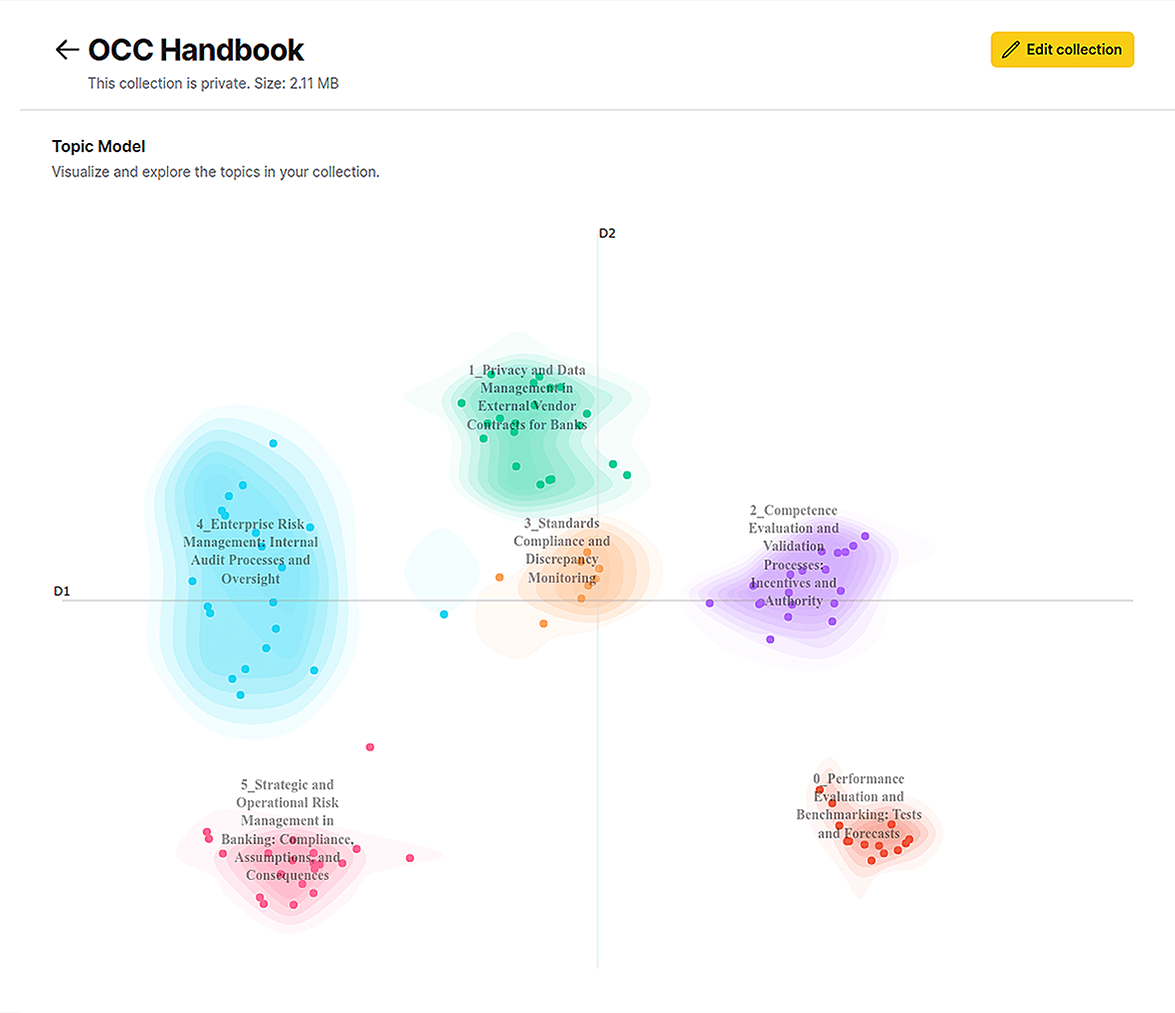
Question generation
Automated Q&A pair test generation process
1. Stratified sampling: Utilize topic modeling and sampling techniques to ensure diverse and balanced test cases.
2. Generation using LLMs: Generate questions across topics and query types, including:
- Simple factual
- Multi-hop
- Reasoning
- Numerical inference
- Yes/No or multiple choice
3. Quality self-check: Apply evaluation metrics to select high-quality test cases.
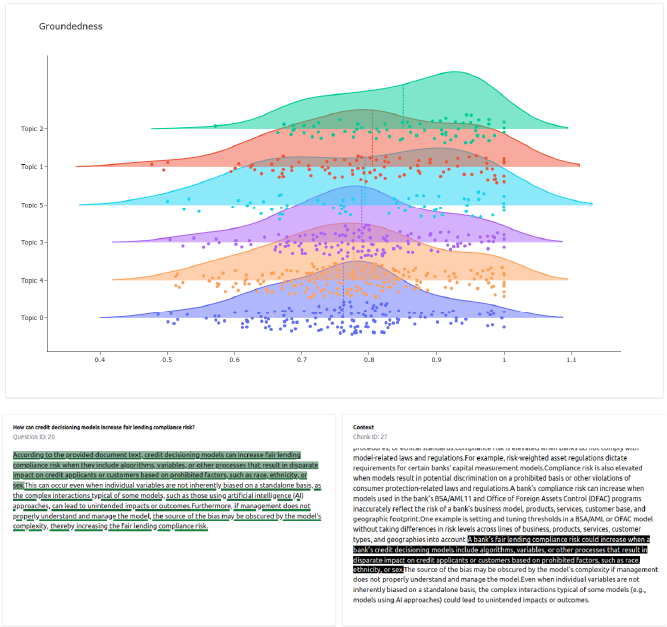
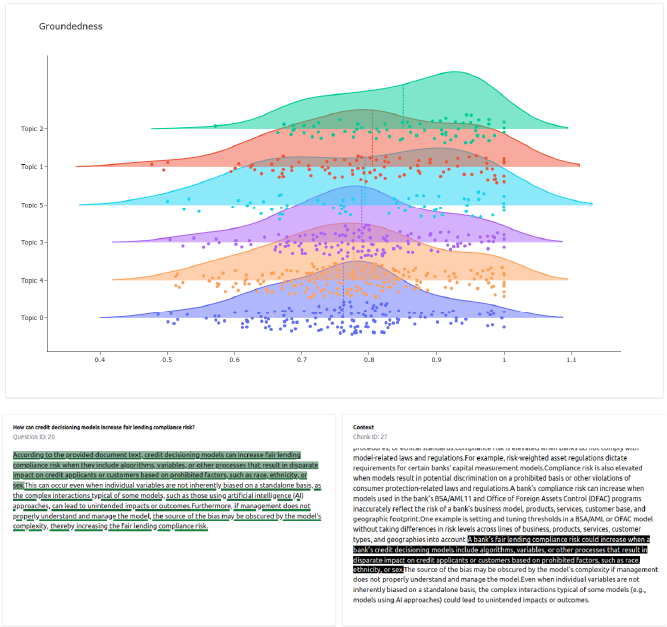
Transparent and explainable metrics
Regulatory compliance requires accountability, transparency, and risk mitigation in AI systems. h2oGPTe enables robust auditing and reporting to meet compliance with industry standards, building trust and user confidence by making decisions interpretable and validating outputs.
These measures reduce risks of harmful deployment, support continuous improvement by identifying weaknesses, and promote targeted updates to enhance model performance and adherence to regulations.
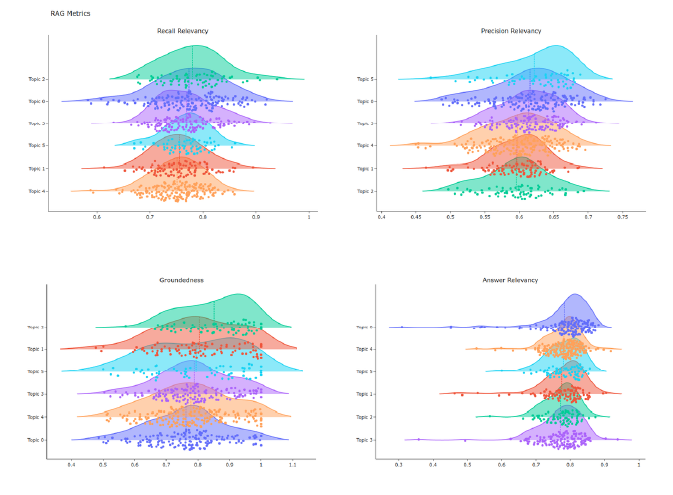
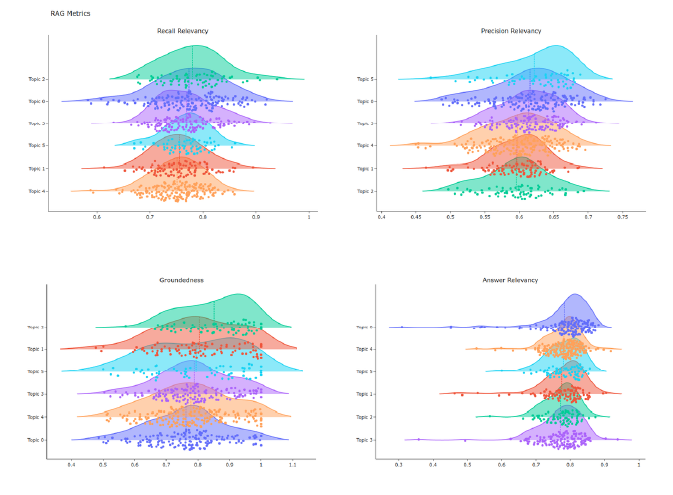
Visualization of results
h2oGPTe visualizes results including marginal analysis using distribution and violin plots to represent performance metrics like accuracy and groundedness across dimensions such as topics and query types.
h2oGPTe provides bivariate analysis using heatmaps to depict performance metrics in relation to topics and query types, offering deeper insights into relationships between these factors.
Enterprise h2oGTe provides:
Calibration
Calibration that bridges machine metrics with human judgment to deliver trustworthy evaluations in regulated domains. Conformal prediction ensures reliable uncertainty quantification through transductive and split methods, achieving a balance between accuracy and efficiency.
This approach enhances automated evaluations with transparent confidence intervals, reduces labeling through active learning, and adapts seamlessly to computational demands.
Weakness detection
Weakness discovery to identify performance gaps in generative AI models through marginal and bivariate analyses, clustering failure cases to uncover patterns such as hallucinations or challenges with "why" questions.
These insights enable targeted improvements, focused retraining, and effective risk mitigation, ensuring safer and more robust deployments.
Robustness
Robustness in Retrieval-Augmented Generation (RAG) systems to deliver accurate and coherent responses across diverse inputs, including adversarial, noisy, and out-ofdistribution queries.
Testing covers ambiguous, noisy, edge-case, and domain-specific jargon inputs, evaluating the system's capability to manage ambiguity, errors, and complexity with reliability.