H2O Driverless AI with IBM Power
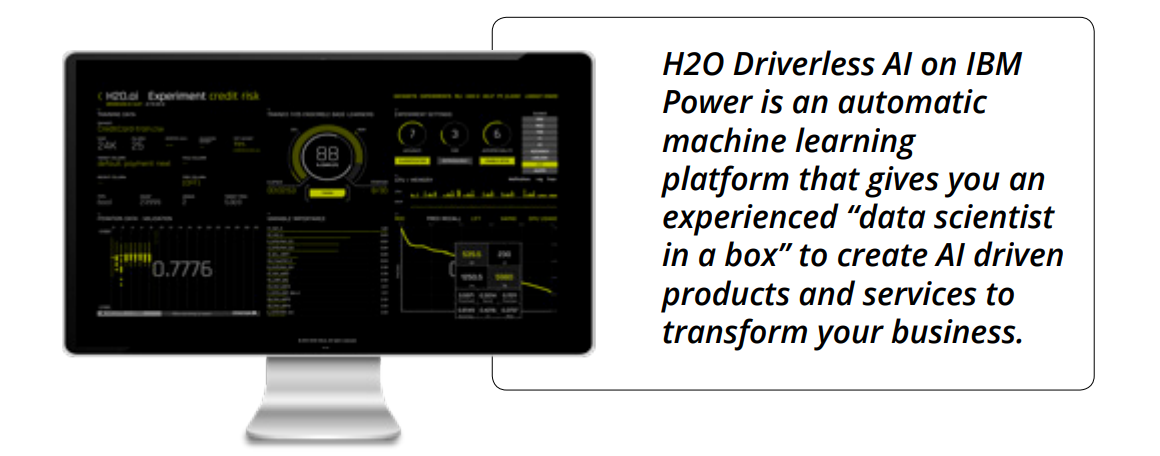
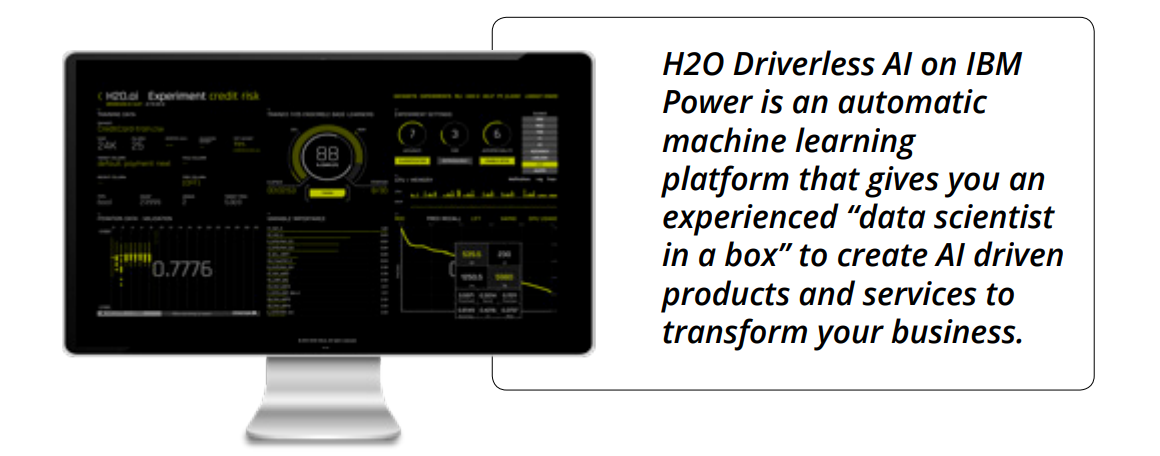
Delivering AI at Scale
Businesses everywhere have realized that their unique data is key to competitive success and now want to put that their data to work with AI. To scale, data science teams need to adopt new tools and techniques that will allow them to get better results and quickly deliver more insights to the business.
Faster AI Development
Increasing the business impact of AI by solving a wider variety of business problems is a key goal of every successful data science team. H2O Driverless AI is optimized to run with GPU acceleration and automates key portions of the data science process including feature engineering and parameter tuning to dramatically reduce the time needed to produce accurate models.
Time Series Helps Forecast Sales, Predict Industrial Machine Failure and More
With the time series capability in Driverless AI, H2O.ai directly addresses some of the most pressing concerns of organizations across industries for use cases such as transactional data in capital markets,
in retail to track in-store and online sales, and in manufacturing with sensor data to improve supply chain or predictive maintenance.
Trusted AI Results
Delivering machine learning results your business can trust is a key goal of data science teams. H2O Driverless AI delivers highly accurate models with machine interpretability that helps explain how the models work to the business. Delivering trusted and transparent results increases adoption of AI and also allows your company to comply with government regulations.
Easy AI Deployment
Model deployment remains one of the most common challenges for data scientists. Models can take weeks or even months to reach production and may be modified to work with production systems. H2O Driverless AI creates ultra-low latency automatic scoring pipelines for easy deployment. In addition, H2O supports training, testing and model versioning so that data science and business teams can work together to bring models from data science to production in minutes, not months.


Key Features of H2O Driverless AI
AutoViz – Exploratory Data Analysis for Big Data
H2O Driverless AI AutoViz automatically creates data plots based on the most relevant data statistics to help users understand data prior to starting the model building process. This is helpful for data scientists and data engineers who want to better understand the composition of very large data sets and see trends and possible issues such as large numbers of missing values or significant outliers that could impact modelling results. interpretability that helps explain how the models work to the business. Delivering trusted and transparent results increases adoption of AI and also allows your company to comply with government regulations.
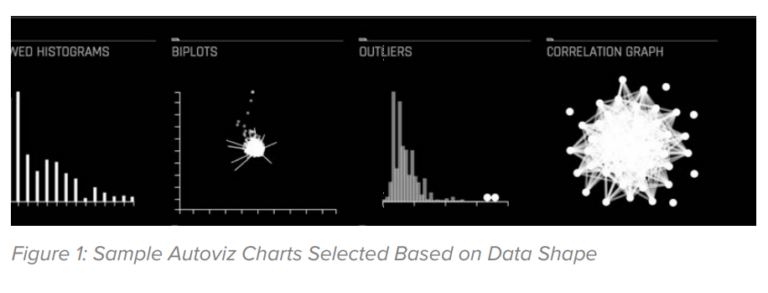
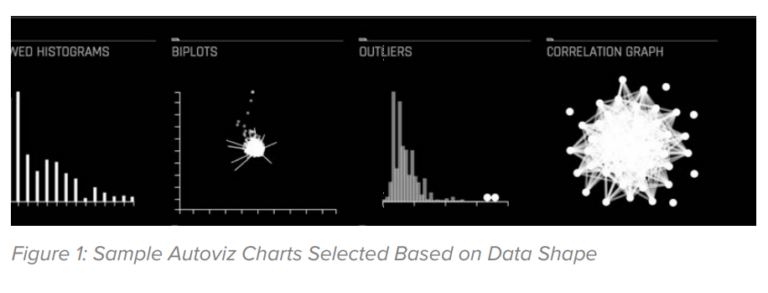
Automatic Feature Engineering and Model Building Feature engineering is the secret weapon that advanced data scientists use to extract the most accurate results from algorithms. H2O Driverless AI employs a library of algorithms and feature transformations to automatically engineer new, high value features for a given dataset. Included in the interface is an easy to read variable importance chart that shows the significance of original and newly engineered features.
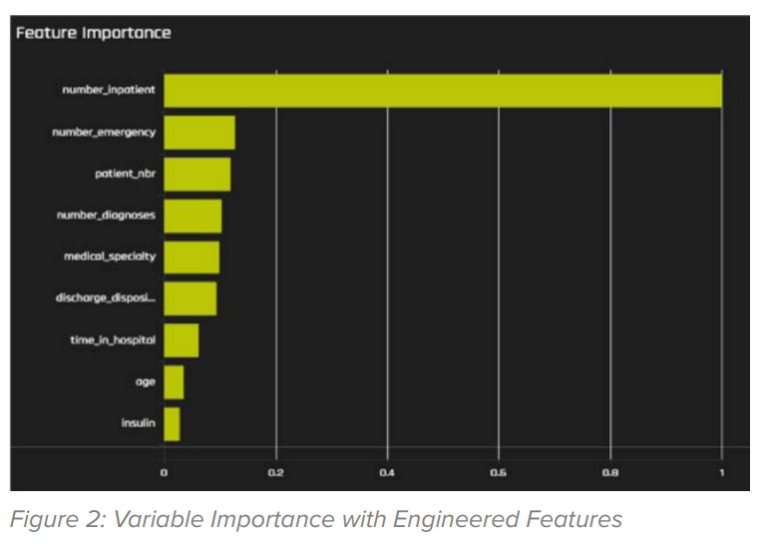
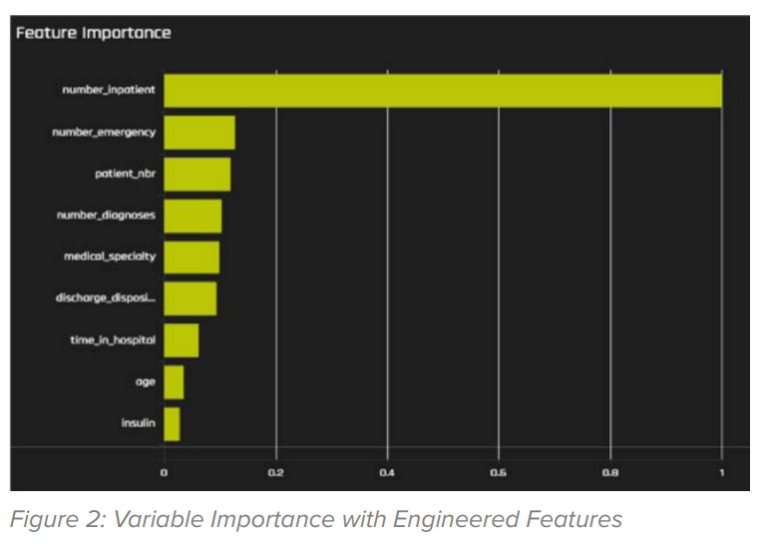
Machine Learning Interpretability (MLI)
H2O Driverless AI provides robust interpretably of machine learning techniques and results. In the MLI view, four charts are generated automatically including: K-LIME, Variable Importance, Decision Tree and Partial Dependence. Each chart helps to explore the modeling techniques and results more closely. These techniques are crucial for those who must explain their models to regulators or customers.
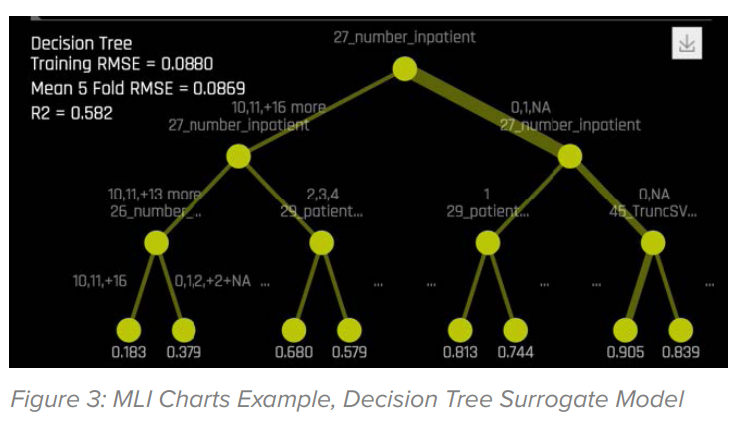
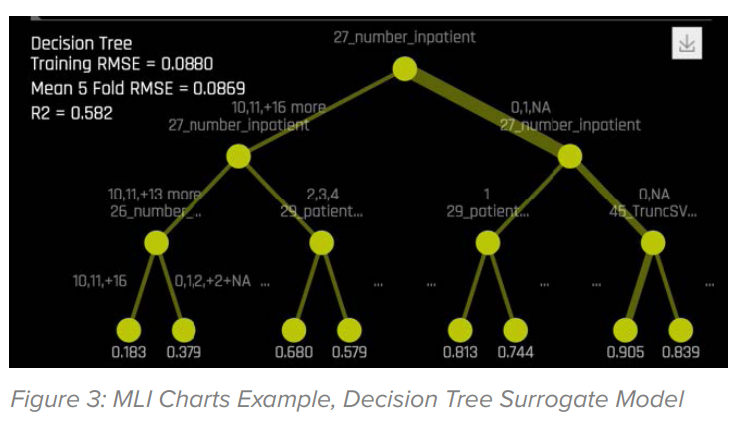
Automatic Scoring Pipelines
H2O Driverless AI automatically generates both Python scoring pipelines and new ultra-low latency automatic scoring pipelines. The new automatic scoring pipeline is a unique technology that deploys all feature engineering and the winning machine learning model in a highly optimized, low latency production ready Java code that can be deployed anywhere. This technology is critical for enterprises running models that need ultra-fast scoring for real-time applications running on a range of devices.
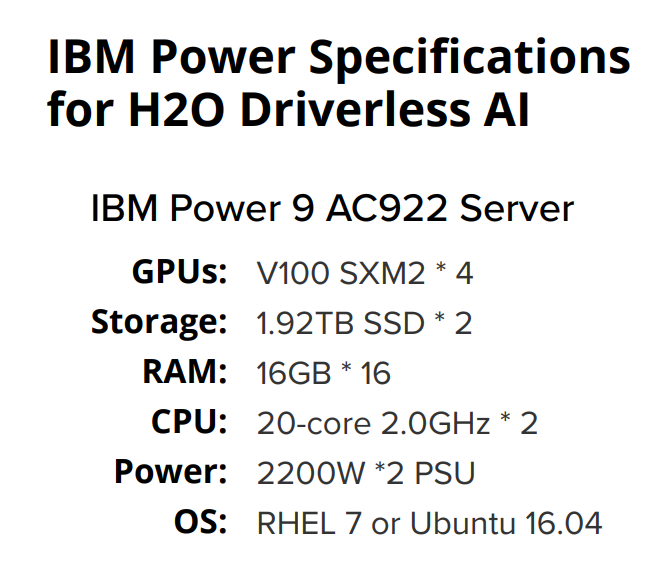
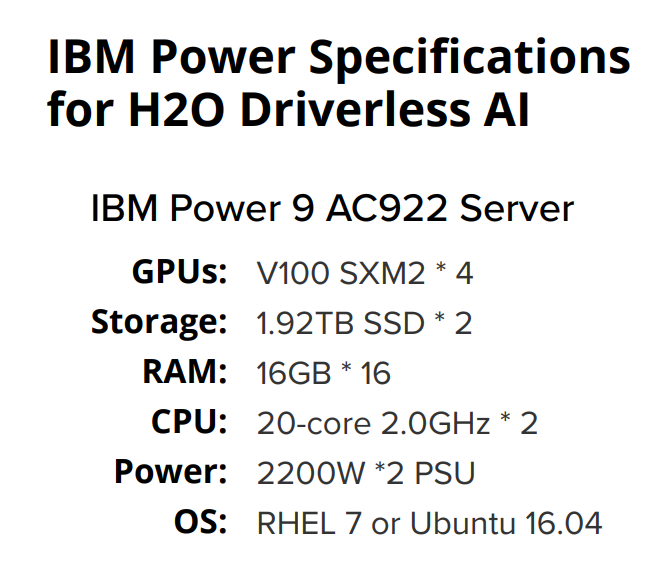