Background
The rapid spread of COVID-19 has presented the world with unprecedented challenges and new norms to adapt to: workforces going completely virtual and digital, little to zero travel, and significant uncertainty for small businesses.
The pandemic has had an immediately visible impact on the financial and economic health of many nations. The United States saw 21M new Jobless claims ( a 30 Sigma Event )1, banks hitting their lending ceilings2, and potentially most important one-third of renters failed to pay April rent3.
In response to these challenges, the United States Congress as well as the Federal reserve have launched over $2 Trillion stimulus programs to try to stabilize the economy while citizens lockdown and combat the spread of this pandemic. Much of this stimulus small business lending and interest rate adjustments to try to prevent businesses from shutting down or individuals failing to pay their mortgages. Now more than ever it is critical that companies understand their risks when it comes to lending and outline a strategy for lending in the COVID-19 environment.
Historical Context
Part of what makes the response to COVID-19 so difficult is that it quite literally is a challenge the world has never faced before. During the Global Financial Crisis a deeply mispriced housing market was exacerbated by extremely over-leveraged financial instruments and the Dot Com bubble was the result of wild overspeculation on the growth of internet companies, but these are both materially different then what the world is facing today. Meanwhile, it is impossible to compare the current response to the last major pandemic which was approximately a century ago.
What AI Can Do:
Response and execution will be critical for financial institutions and H2O.ai believes the way to do that is with AI and machine learning. Many banks are already under fire for branches not being able to accept PPP (Paycheck Protection Program) applications or not having the infrastructure to support this new initiative. There are a few core areas where AI and machine learning can be applied during the COVID-19 response:
Automated and Enhanced Risk Modeling: There are many different models that banks apply to understand their credit risk and in our current increased risk environment, these models should be revisited with new economic data and new enhanced modeling methodologies. These are systemically important models to banks so there should be additional focus on explainability and understanding of why these models are coming to the conclusions that they are. Because response time and execution is absolutely critical right now for small businesses and individuals to not default on their current financial obligations, banks and lenders should be deploying automatic machine learning (AutoML) to update and improve these models as quickly as they can.
Probability of Default(PD): A probability of default model uses multivariate analysis and examines multiple characteristics or variables of the borrower, and it will usually account for credit or business cycles by either incorporating current financial data into the generation of the model or by including economic adjustments.6
Loss Given Default (LGD): The amount of money a bank or other financial institution loses when a borrower defaults on a loan, depicted as a percentage of total exposure at the time of default. A financial institution’s total LGD is calculated after a review of all outstanding loans using cumulative losses and exposure.7
Exposure At Default(EAD): The total value a bank is exposed to when a loan defaults. Using the internal ratings-based (IRB) approach, financial institutions calculate their risk. Banks often use internal risk management default models to estimate respective EAD systems. Outside of the banking industry, EAD is known as credit exposure.8
Foreclosure Risk: Analyzing historical foreclosure time-series data and incorporating external/economic data to get deeper predictive insights to potential future foreclosure trends.
Forbearance: Requests for forbearance, essentially a temporary stop and prevention to foreclosures, have increased by a factor of 10 in response to COVID-19 concerns.
Administration & Processing: Some initial concerns about the roll-out of the stimulus plan features such as the PPP were around financial institutions’ readiness for the volume of applications and decision making process around this administration. H2O.ai believes that AutoML can and should be applied to rapidly increase lenders abilities to rapidly process these applications and quantify the risk profile of each of these applicants. Whether it is applying NLP to automatically gain insight into the characteristics of these businesses, or classification to automatically sort these applications intelligently to optimize handling, there are plenty of opportunities for banks to take advantage of technology to create efficiency at a critical point in time.
Explainability: It is more important now than ever before to understand why your predictive models are coming to the conclusions that they did. Perhaps more importantly, leveraging tools like Driverless AI or Q to avoid unnecessary complexity in highly volatile environments can be critical being resilient to further macroeconomic shocks.
Scenario, Sensitivity, and What-If Analysis: After your model has been trained & backtested it can be critical to analyze how it would perform in new or historically rare scenarios. Having automated and interactive scenario modeling tools enables you to recreate black-swan situations like the Global Financial Crisis with one click.
Augment & Enrich Data: Platforms like Q Lending enable seamless transformation & augmentation of data sets. It can be extremely valuable to be able to attach external historical macroeconomic data, public markets data, mobility data, 3rd party mortgage analytics data, google trends, or census data to predictive models.
What to Consider Going Forward:
While most firms are completely focused on handling the challenges of the present, it is important to not lose sight of what may come next. As of April 19th, the United States has seen over 21 Million new jobless claims.
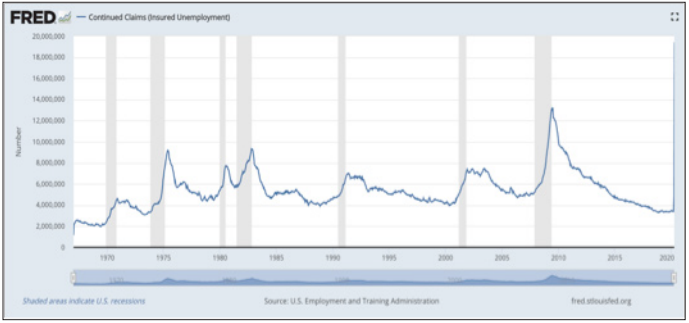
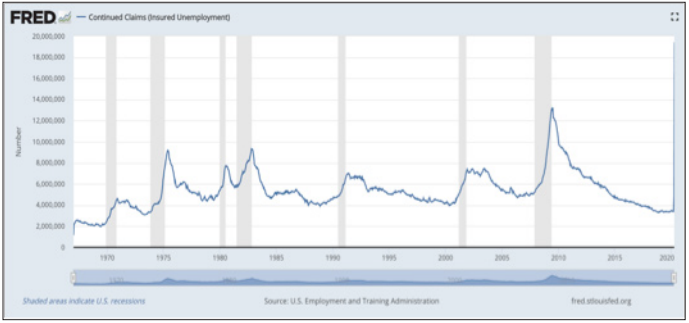
It is important to preface any future speculation that the one common theme among economists, banks, and finance professionals is that there is no consensus on how extreme or long the results of this could be.9
Given all of the various macroeconomic theories being considered at this point in time, it is best to just focus on what we know and the data that we have, then discuss potential responses.
21 Million Unemployment Claims over the last month
4.4% Unemployment Rate in March, from 3.5 in February
31% Percent of Renters did not pay April Rent
27 Days of cash reserves is the median amount across all small
businesses
How AI and Machine Learning Can Help
H2O.ai has developed a new set of AI apps by combining the H2O Q and Driverless AI platforms together to bring automated insights and automated machine learning to sets of business problems. H2O.ai created a new Q Lending App to address the challenges in the mortgage and loan risk modeling space. Given the rate at which lenders are taking on and creating new loans, it is more important than ever to deploy the best methods to determine how likely a borrower might be to default on these loans.
With the new Q Lending App, public data sets & internal data sets can be added to enrich the data set used to create predictive models.
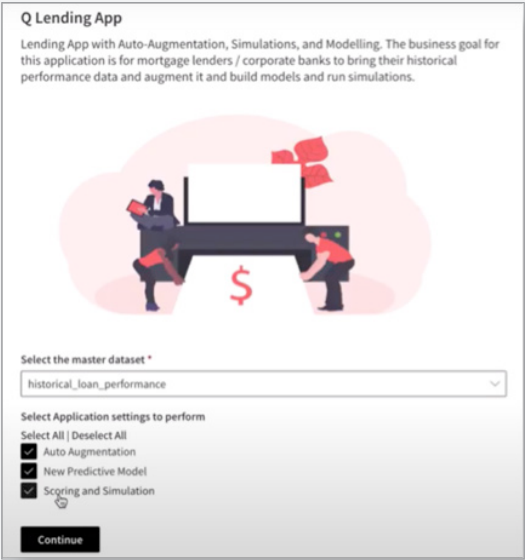
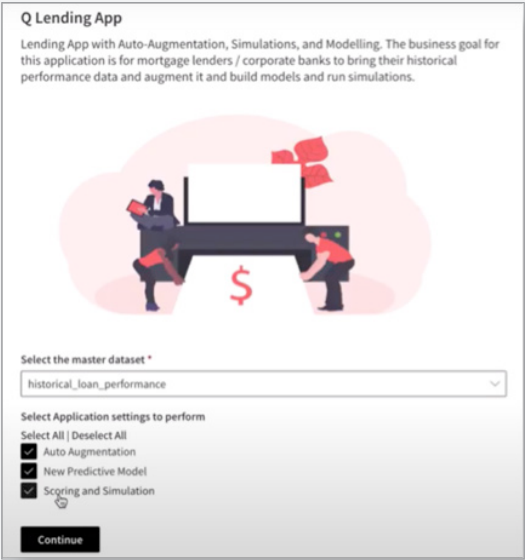
Public data such as State Census information or internal private data that might help predictiveness can be appended and joined into the data set for the model.
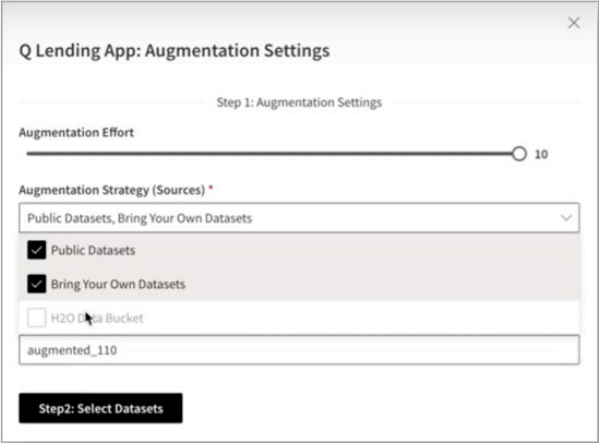
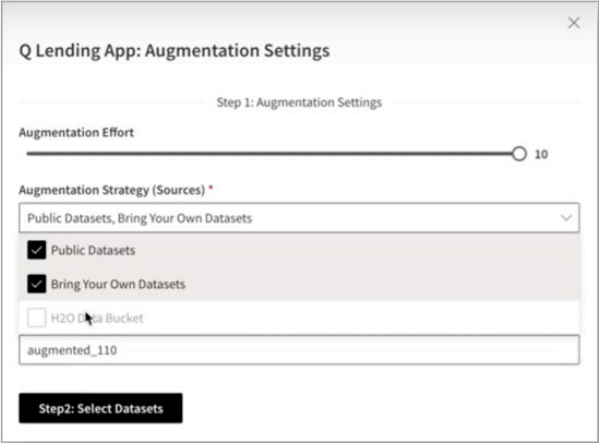
The Q Lending App can directly bridge to Driverless AI to run the full suite of predictive models.
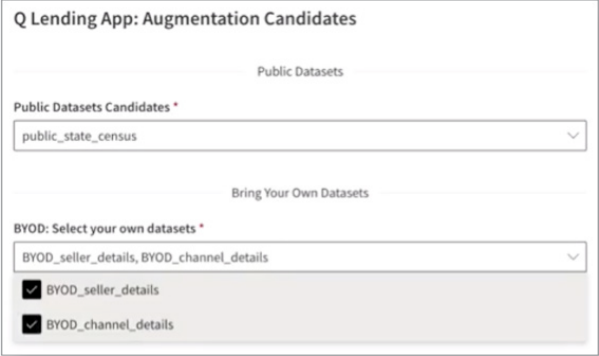
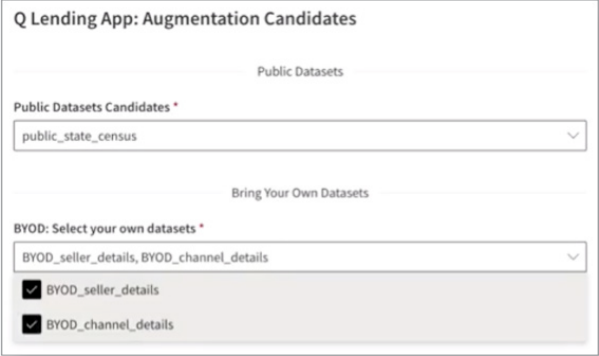
The Q Lending App can directly bridge to Driverless AI to run the full suite of predictive models.
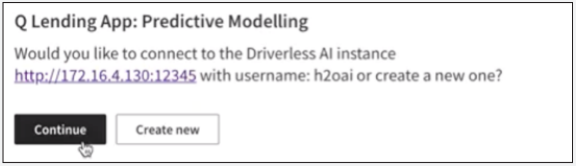
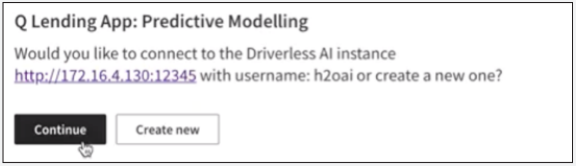
Here Q prepares the data, runs the various simulations and experiments.
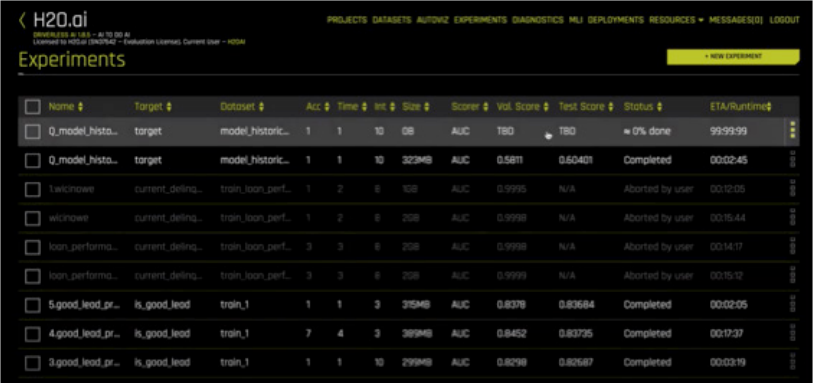
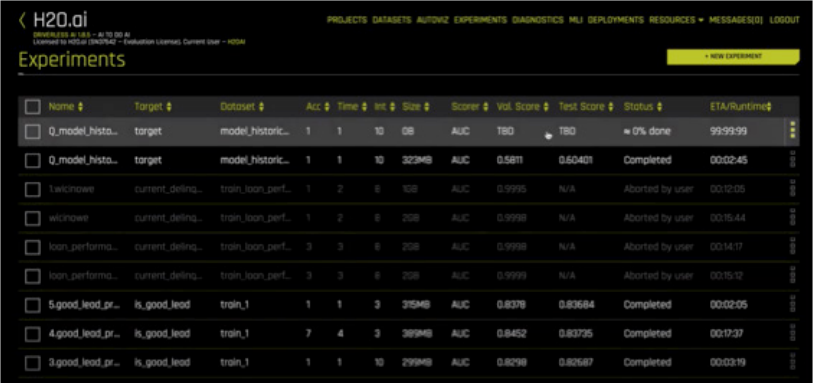
Once the experiment is finished, the dashboard displays the probability of default for the portfolio of loans as well as many variables we can adjust to determine extreme scenarios.
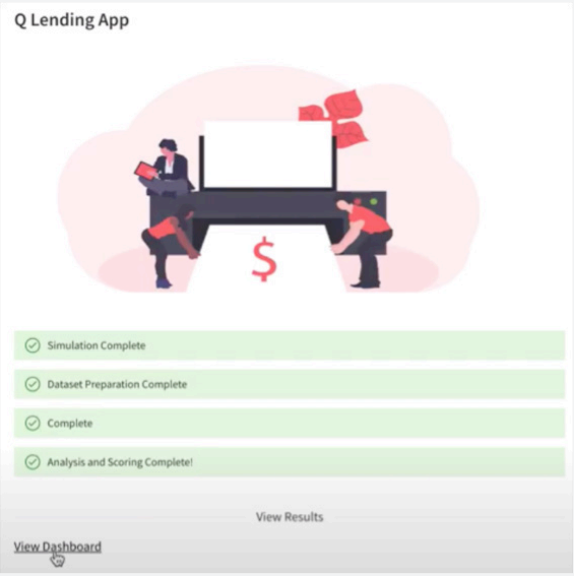
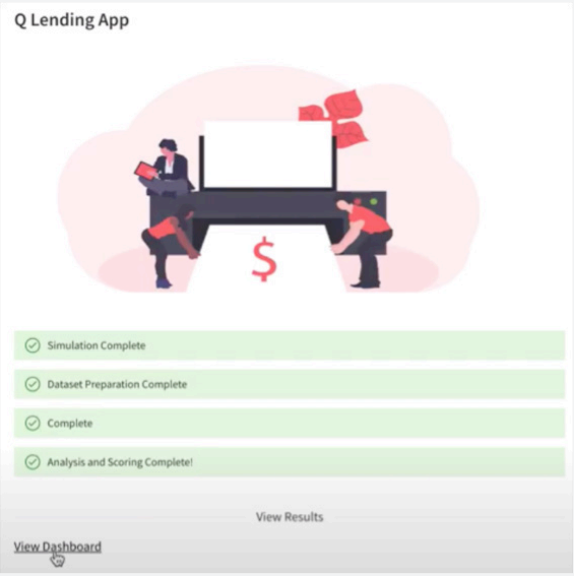
This dashboard is entirely flexible and interactive so catastrophic scenarios such as a sharp rise in unemployment can be analyzed.
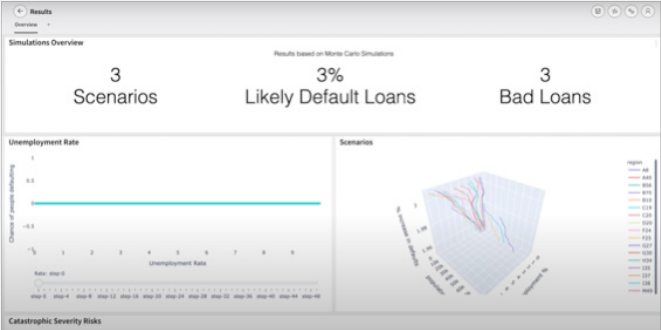
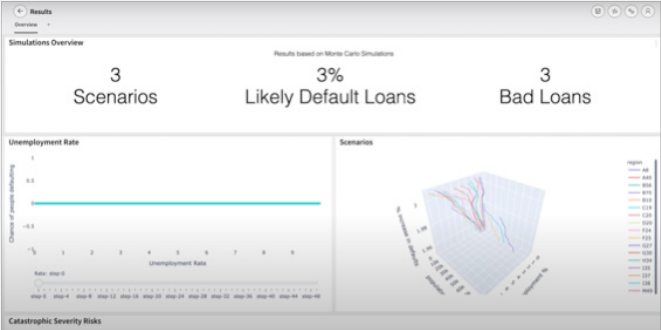
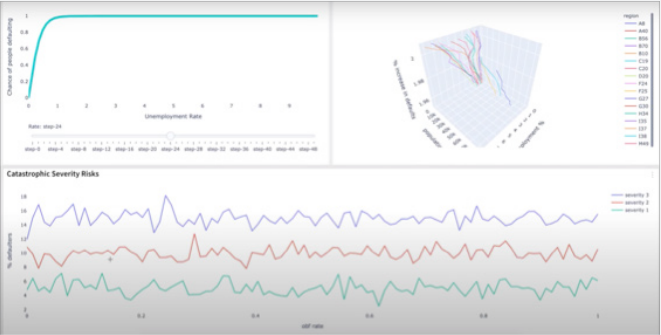
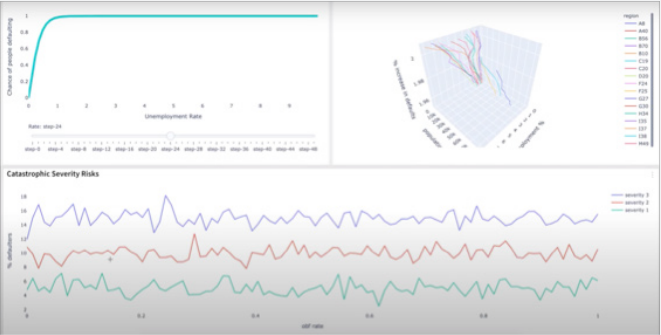
Conclusion: COVID-19 has created unprecedented uncertainty for consumers and businesses alike. However, banks and lending institutions have a significant responsibility to quickly respond and address the PPP demands from customers and the government. This is a daunting challenge but lenders have an opportunity to look to innovate existing processes and leverage new AI and Machine Learning such as the new Q Lending App to adapt to the new market environment.