Know Your Customer
Using Artificial Intelligence and Machine Learning to Help Financial Institutions Increase Compliance with Know Your Customer (KYC) Regulations
Recent years saw an increased focus on regulations enforcing anti-money laundering (AML) laws in both the US and abroad. One of the key components of AML is a Know Your Customer (KYC) guidelines – a globally developed set of directives designed to ensure that companies collect detailed anti-corruption due-diligence information to know exactly whom they are doing business with and avoid interacting with parties involved in illicit and unlawful business.
While it’s difficult to assess the total amount of money that goes through laundering circles worldwide, organizations such as UNODOC (United Nations Office on Drugs and Crime) estimate that between 2-5% of global GDP, as much as 2 trillion US Dollars, may be laundered every year. KYC and other related regulations are designed to provide a framework for financial institutions operating on a global scale to use data to combat the money laundering problem. However, it is often challenging for companies to determine precisely what information to gather as part of the AML risk assessment and to set up operational procedures to handle large amounts of data from multiple sources.
While certain processes can be outsourced to third parties, the final responsibility for compliance ultimately lies within the financial institutions themselves. With an increased effort on enforcing adherence to AML standards and a growing number of penalties levied against global financial institutions in recent years, companies must step up their efforts to improve their understanding of the AML requirements and develop efficient solutions for risk assessment and mitigation. For financial institutions, KYC compliance is not an optional requirement. Regardless of whom you are doing business with: international or domestic corporations, non-profits, or foreign governments, failure to follow AML guidelines can result in audits, fines, or a ban on conducting business in specific countries or regions.
With millions of transactions going through every day, how do you differentiate between the potentially risky and compliant transactions? How do you weed out the false positives and focus on the records that may require further investigation? To help make sense of the evergrowing volume of information, many companies are turning to Artificial Intelligence (AI) to help them navigate the sea of data, analyze suspicious transactions, and identify patterns and trends that might point to risky and malicious behavior.
Changing fraud landscape requires new approaches to detection tools and practices
Money laundering and financial fraud constantly evolve to avoid detection in manners similar to smuggling, trafficking, etc. As new and promising techniques are developed to identify and flag suspicious behaviors, illicit business methods change and adapt to stay under the radar. Monitoring these complex and constantly evolving practices using legacy methods based on business rules and traditional statistical approaches can be slow, laborious, and costly. Trying to manually deduce patterns from merchant behaviors by sifting through large pools of unstructured data, often in different formats and languages, and dealing with a possibility of a high volume of false positive results can consume extensive human and infrastructure resources, and not get an organization any closer to being assured that they are dealing with a legitimate business partner.
Using conventional automation and data mining techniques for fraud detection is further complicated by the fact that there’s very little organized historical data available for companies on what types of activities to look for, and what types of transactions should trigger alerts.
Machine Learning (ML) and Artificial Intelligence (AI) are fueling innovation by transforming the way organizations view data analytics. Instead of giving computers rules to solve problems, businesses are granting machines access to data and asking them questions, so that they can learn and “think” of solutions for themselves. These technologies are ideally suited for building a comprehensive, 360° view of the customer by integrating information from various sources, studying the typical money transfer patterns and learning to differentiate between usual and suspicious behaviors. AI solutions are able to find patterns that rule-based tools aren’t able to detect, and can continuously learn and adapt in response to changing behaviors, environments and regulations.
A global survey of 424 senior executives from financial institutions and fintech companies and leading experts in the field found that nearly six in ten (58%) respondents believe that machine learning will help them “greatly enhance” their risk-assessment processes. Furthermore, 29% of surveyed executives expected their companies to use AI specifically for KYC and AML monitoring within the next three years.
Using AI to solve AML operational challenges
Most companies operate in a reality of a complex IT landscape, where legacy systems coexist with modern applications. And while IT modernization and enterprise data integration initiatives are high on today’s CIOs agenda for companies of all types and sizes, most organizations still have to deal with data that is scattered throughout a variety of disparate data stores.
- Unified pool of data: The true advantage of AI-based monitoring lies in its ability to harness, cleanse, and validate data from multiple sources. Data collected from internal and external sources gets aggregated in a central pool, where it can be consolidated, annotated and enhanced to make it more searchable, context-sensitive and relevant for specific users. AI and Machine Learning solutions help facilitate Master Data Management (MDM) – a methodology that delivers a single view of data from disparate, and often conflicting, sources. MDM also helps identify relationships between pieces of data, creating a complete view of all interactions between parties, products and events that help build a more complete, consistent, 360° view of a customer.
- Probabilistic matching: When a financial services company tries to integrate data from multiple sources, they inevitably run into an issue of duplicate records and inconsistent formats. For instance, Jon Taylor, J.R. Taylor, and Jonathan R. Taylor may be the same person, but a conventional database isn’t able to flag these records as possible matches. An AI solution can help implement probabilistic matching, allowing banks to consolidate and integrate information pertaining to the same customer, building a more complete picture of whom they are working with.
- Pattern-based vs. rule-based analytics: Consider a situation where a bank’s client transfers a large sum of money to his cousin’s account overseas, and the cousin’s wife withdraws it. The familial ties between the people involved in this transaction are not obvious to the client’s bank (the cousins and their spouses may not share last names, for example), so a rulesbased engine is not likely to flag this transaction as suspicious. A pattern-based approach however, can learn that if similar transactions occur on a regular basis, it might signal possible money laundering activity that warrants further investigation. AI tools that analyze and learn from patterns without preconceived sets of rules are indispensable in situations where connections between parties or types of transactions are less-than-obvious and may not trigger red flags, but patterns of unusual behavior might still indicate suspicious activity.
- Solutions that get smarter with time: Unlike conventional data mining tools, AI technologies can learn from previous cases and ongoing updates and incorporate this knowledge into future searches and analyses. It can also add new information to past knowledge, flagging, for instance, transactions linked to regions that have recently been classified as unstable.
Creating a 360° view of a customer using data across key domains: Industry, Relationships, and Interactions
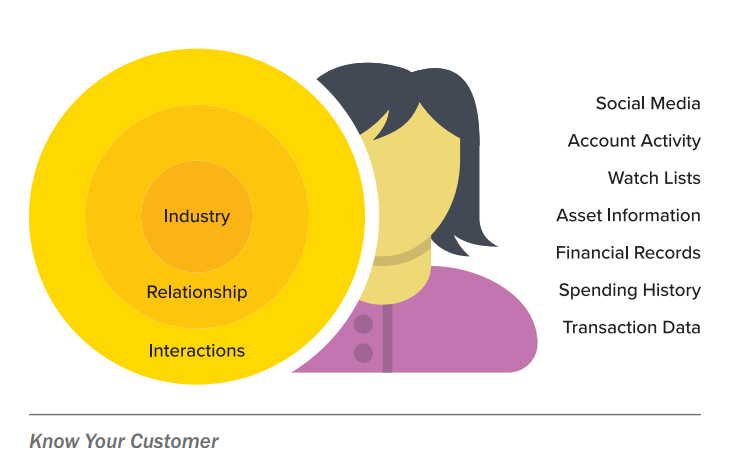
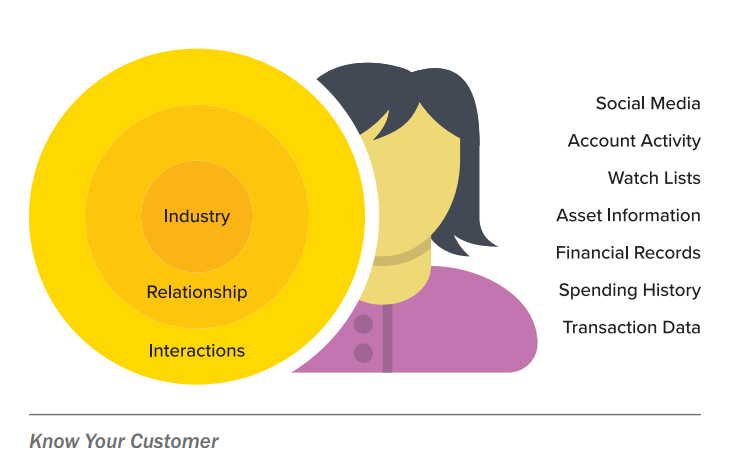
The ultimate goal of using AI for KYC compliance is not to build a customer profile, or to mark off the items on a government compliance checklist. The real purpose of KYC compliance is to really know your customer at the point when you are doing business with them, even when you have thousands or millions of customers and prospects.
Each organization needs to develop a risk-based approach by analyzing multiple data points across the key domains, including Industry, Relationships, and Interactions and apply this organizational knowledge to all dealings with existing and perspective clients.
Industry: Governments, third parties, and individual companies maintain lists of industry-specific watch lists that help identify connections with blacklisted organizations within a specific vertical. Manually checking against these lists is hardly practical, and new events, shifts in markets and political landscapes can drastically change these watch lists in a matter of hours. An automated AI solution can combine data from surveying the news, social media and other sources to maintain the most accurate picture of what’s going on in a specific industry or market, and what new threats might emerge as a result of recent events.
Relationships: Spending history, account activity, account activation and deactivation, transaction data, current and past asset information are among the data points that can provide a holistic view of a person’s, or business entity’s profile. But when evaluating a prospective client, it’s also important to look at their relationships with other individuals and institutions. Sources such as social media, online publications, and financial records can help identify links between your prospective business partner and other entities and people.
Interactions: Ongoing monitoring of the client’s interactions with other parties can help identify patterns of unusual behavior, which may need to be further investigated. While there’s no framework or formal set of recommendations on what should be regarded as suspicious interactions, certain deviations from typical patterns may trigger further probing to look at the significance of specific events or transactions. Modern Day communication patterns shaped by the rise of social media and other peer-to-peer interactions can provide a wealth of data when examining a prospective partners’ social and business interactions.
A 360° customer view helps build strong marketing strategies
The wealth of information that is gathered and analyzed for KYC assessments allows businesses to build a comprehensive, 360° view of their customers, unlocking nearly limitless opportunities for creating more effective, tailored, and personalized marketing and sales tactics. Having an in-depth understanding of a customer’s preferences, patterns, likes and dislikes, purchase and spending history, and other behaviors can help financial services companies identify and retain their most profitable clients by tailoring campaigns and upsell strategies to their specific needs. A comprehensive KYC approach can help deliver superior customer experience at a point of service, improve campaign effectiveness and help banks anticipate and prevent churn by spotting unusual activities and offering targeted programs to keep customers happy.
Naturally, no amount of data gathering and monitoring can completely prevent and control all activities that may cause harm to a financial institution’s business and reputation. However, putting in place intelligent triggers and thresholds, combined with organizational knowledge, judgment, and experience, can help companies increase KYC compliance, reduce the risk associated with illegal business practices and design better tools for marketing and sales. Emerging technologies such as Artificial Intelligence and Machine Learning are ideally suited to help you on your journey of safeguarding your business, enhancing its resilience against potential threats, and getting to know your customer better for creating and maintaining more intimate, personalized and targeted business relationships.