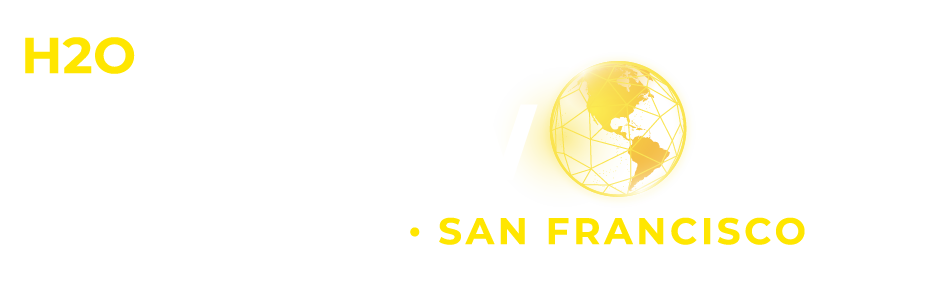
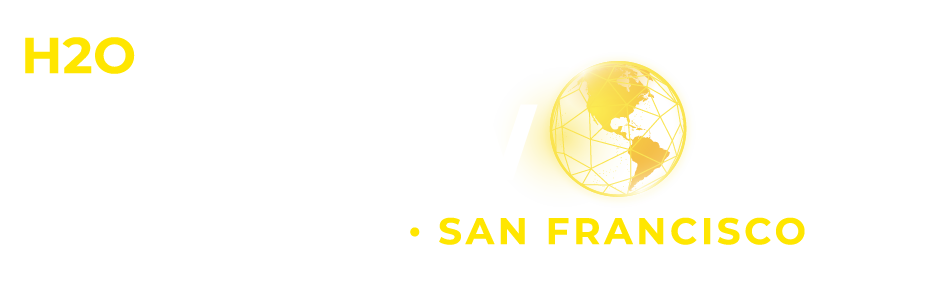
Open-Source AI: Community is the Way
Alexy Khrabrov, Open Source Science Community Director, IBM
AI-generated transcript
00:07
Hello everybody, my name is Alexy Krobrov. I'm the open source science director at IBM Research at our XLR Discovery team, which is a global team helping scientists solve hard problems facing humanity, such as climate change, drug discovery, and so forth.
00:24
I'm also recently elected as chair of the newly established Genitify Commons at the Linux Foundation. So we have very strong alignment with H2O on open source science. You can reach me at chief scientist almost everywhere on Twitter, Telegram, my own website.
00:42
I'm very happy to talk. So my email is Alexy at chiefscience .org. It's my community email. My IBM email is Alexy@ibm.com. And LMAvalanche is an event that I created and ran in June next door at the Contemporary Jewish Museum.
00:55
On a short notice, we basically did a meetup leading into the Databricks Summit. And we gathered 1 ,000 people, snaking around downtown San Francisco, that just shows again how important and sort of after this topic is.
01:12
So we need a community. We're here, we gather together to learn about these topics. There's a very natural question, why are we here? We could have sat at home, drink coffee or wine and read a bunch of blogs as we usually do, right?
01:35
But we took some time and we came here. We meet each other, we talk to each other. What is the reason for this? Why do we come to computer conferences? And I must say, I'm also founder of Scale by the Bay, which is a 10 year old conference, which is next week.
01:48
And I'll invite you guys to it shortly. But I also run a meetup by area AI, which is most established AI meetup in San Francisco area. And again, we came back after pandemic. We're on at full speed. We got getting 100 people after a summit.
02:04
We got full house after PyTorch conference. So why do people take time and come long distance? So it's not just communities, not just camaraderie. I think open source has very special and similar dynamic, right?
02:18
People like to work together if this is their passion, right? I mean, you might come to the office because now you have returned to work policy, but we need humans to gather together and ascertain certain truths.
02:30
So when I was an immigrant 30 years ago, coming to America, I read this book by Max Lerner called America as a Civilization. Everything I know about the US, I learned from that book. I don't need anything else.
02:40
I came here, the book is true. I hit the ground running. Highly recommend it. It was a bestseller published for 50 years. For some reason it kind of came out of vogue, but it's very true. And so one observation in that book was that if you look at every American court drama, right?
02:56
Or kind of a movie drama, it ends with a big public scene. The truth is revealed in the public setting. such as a courtroom or at a wedding, everybody is gathered, right? The main characters run in and some final truth is revealed, some mission of love or secrets and so forth, right?
03:16
So why is that? As humans, we need to ascertain the truth together, especially given the uncertainty, given huge amount of information, right? You can think like, we should go this way, we should go that way.
03:29
And of course, now in this climate, a lot of information we get is marketing, right? And obviously smart folks do their job. They tell you that their product is good and that you should buy it. And especially in Silicon Valley with a sense of big data, I think we see the kind of predominance of this line of thinking and it takes a lot of hard work to actually understand where the direction of the future is.
03:55
And so I think open source does a very important service in addition to being available, transparent and collaborative. Open source gives us a mechanism by which we ascertain the direction of the future.
04:09
Where should we apply our efforts? And specifically, I think in the case of LLMs, right? There is so much information packed in so little time that we gather like this and we figure out together what we should do, right?
04:23
And obviously, different gatherings have different focus. So if it's a vendor conference, you know, this is what we should do as customers, right? Or if it's a community conference, what we should do with the set of open source projects?
04:33
I really love the fact that this is both, right? Like this is best of both worlds. So most importantly, when you see something like a machine learning model, the key questions for business adoption surrounding this model are trust, transparency, performance.
04:50
Note that these are all claims that cannot be made unilaterally. So when you see a company saying that our model is trustworthy or it's our model is safe for business, or you see yesterday OpenAI made an announcement, right, that we have all this...
05:04
new GPTs that will be verified for usability by their own safety guidelines. You don't know really what the safety guidelines are. You know that they put a huge amount of work into these systems of safety and responsibility, but you do not know how they work, right?
05:20
This is all the judgment of one company. And what is extremely disturbing, I've just seen on Twitter, my friend posted, if you want to use a SaaS platform run on AI, such as Google Magic Editor, if you want to edit your photos, apparently it will not let you do things that consider as harmful.
05:36
It will not let you edit driver licenses. It will not let you edit people's features, because it will think that you are basically forging something, right? And obviously in this case it makes sense, but the SaaS systems make decisions for you and you are not aware of them.
05:52
So this kind of claims cannot be done by one company. Moreover, as in this case of the Magic Editor, many people will object to this, right? We need to ascertain and adjudicate these claims. This is very important, right?
06:07
We should kind of step back. In any way, we see a claim of trust or performance, right? Because the model should solve a business task. If it needs to answer a specific question, who will judge how well it does it?
06:20
Of course, the company will say, the vendor will say, it does, you know, it works well. But as we know from previous machine learning research, the only reliable way we learned is the task -driven common task setup, where there is a benchmark.
06:34
First, we agree. What is the valid benchmark for this kind of task? Right, and then we have a leaderboard and we have a community process. So one of the bodies which is working in this fashion is ML Commons, who heard about ML Commons.
06:46
ML Commons is a consortium which basically runs ML Perf benchmark and then it runs other benchmarks following that ML Perf benchmarks measures performance of clouds and large systems for machine learning.
06:57
And it came about because multiple companies needed to basically share the market, right? And if you want to choose a cloud provider for your machine learning workloads, you need to ascertain clients' performance.
07:08
You need to know how much it costs, right? To run something. And so because it was a clear market need, the companies understood that they cannot do it by unilateral claims and performance tables they publish themselves.
07:20
They need to actually compare each other. So the ML Perf came out and ML Commons was formed as a consortium hosting it and then other benchmarks followed. So we need this, right? We need open source community around LLMs to think how we measure trust, performance and responsibility claims.
07:39
And there are other bodies doing this. So this is the kind of, you know, just one year of our open source science activities. So open source science is an initiative which we created at IBM Research in partnership with NumFocus.
07:53
So it belongs to NumFocus, which is the most established nonprofit in data science. Who here heard about Jupiter and Pandas and NumPy? who heard about NumPy? Okay, who heard about NumFocus? Not many people.
08:07
NumFocus is a foundation, right, which hosts them. So this is, you know, a small kind of in terms of budget, but big in terms of, you know, what it does. So that's another reason, right? We don't really have enough marketing in open source community.
08:21
We need to figure it out. Now, what is the open source generative AI? So there are four major components. First of all, there is, you know, there is a dataset. Again, we often don't see, we don't hear where the data is coming from.
08:35
For GPT -4, we explicitly don't know because it's not divulged, right? But for many others, it's hard to understand where the data is coming from. And where the data is coming from leads to surprises who hear heard about the in -ron dataset, the email dataset from in -ron.
08:52
So that was a court case where, you know, email from a company in -ron which failed, right, and lost money to customers. the email was attached as a court record. And now this is actually a publicly available set, one of the largest corporate emails.
09:11
So, and a lot of fellow lambs, which you can see a high -end face, are trained on datasets, which include the Enron dataset. So, when somebody has some business questions pertaining to email, sometimes, strangely, you see business emails spilling out.
09:25
And people in companies who care about privacy, they freak out, because they see business correspondence. They can see threats of business correspondence, clearly, which is internal, coming out. And if folks are not aware of this history, of this public dataset, they will not know what's going on.
09:41
And they will raise alarm, which happened in many cases. So, again, you should know where the data is coming from. And depending on your attitude and your decision -making about this data, you need to be able to clean it.
09:55
You need to be able to rectify it, filter it, mask it, retrain, and so forth. So, we need to focus on the data. You need to have available data, not just have it available. We need to have it very conveniently available.
10:07
So, modern and big dataset apps use Lake Houses. You use distributed dataset databases. If you identify the problems your dataset, you need to be able very quickly to identify all the training documents, which came as a problem, and do something about them.
10:23
So, we need to solve this problem. We need to have community hosted Lake Houses. We need to understand where the data is coming from. IBM approach is we have a clean set of data. We spend a lot of work.
10:34
We have our own lake house, built in open source, and we're able to do all of this, right? And I hope that we can contribute something like this to the community. But this is, again, very important. So, we need to think about data.
10:46
Models, there is a lot of talk about open source models. As you heard, it's very hard to understand, actually. Are they really open source, right? And it's almost like if somebody gives you an executable, right, you can run it for free, but it doesn't have the source code.
11:01
It's not open source. So if somebody gives you a model, you can run, but you don't know what's inside of this. It's not open source. However, even if somebody gives you the model, and you know the architecture of the model, and you have the scripts to train the model, and you can do it.
11:16
If you don't know what data was used for this model by the vendor, you will not be able to reproduce the results, right? So we have a situation which is different from traditional open source. So we need to understand different stages of open source and soft models.
11:29
The current OSI approved licenses do not cover LLMs. We do not have the definition of open source AI. So this is a problem which we need to solve. OSI is a small, non -profit initiative working hard.
11:42
They've been going around the community, presenting their vision. I think we all need to help them. If there are lawyers, legally -minded folks in the audience, please connect to me. We're kind of putting together a working group of various organizations.
11:56
companies which should be able to help with this. I think it's a number one question, right? Let's define the standards of openness. Let's define openness frameworks. Let's rank these models. Let's give them clear badges, how open they are.
12:11
We have applications. I think this is the elephant in the room. Have anybody seen a talk where LLM is deployed to production to replace a big part of existing business such as a call center? Has anybody been in such a talk?
12:27
I guess nobody, right? We did not see such a talk yet. All the talks I've been to about LLMs are toy problems. People are talking to artificial examples, right? Maybe somebody deployed it and somebody is using it.
12:39
I have not seen a single talk where a major bank came out and said, hey, we deployed this thing, interplaced our customer service. It didn't happen yet. Why it didn't happen yet? Because it's very hard, right?
12:50
So we have things like land chain. Things like Lama Index. Startups are trying to get into the space. But as you know, startups often lack the experience. Nobody will give them access to the SAP, to the Oracle Financial Rejection Database, right?
13:06
It's very hard. It will take more than a year. So now a year since deployment of Judge GPT, we do not have a single example of a major institution deploying LLM for business at scale, right? At least I have not seen a talk like this.
13:19
If you have seen a talk like this, please let me know. And I think H2O is very well positioned to probably give the first talk. I am really looking, you know, sure enough, and all like, when you do this, let me know.
13:28
Because I think, like, this is the kind of an example what is needed. It's not enough to have the technology. It's not enough to have best engineers. Because nobody will let your best engineers access your most important financial customer data.
13:45
Without a clear assurance, what will happen to this? Because nobody knows yet what's going to happen to it. Is there, I'm going to slurp all of my SAP data. I don't know. Like, can you assure me that it won't?
13:56
right, what will happen if the system starts to basically send around key data. I think I'm a little bit slow, so I need to catch up. So basically, it turns to these questions. Linux Foundation is up a new group called Genitify Commons.
14:09
It's hosted under the FAI Data Foundation. I've been a founding member of this and I get elected as a chair. So we're going to move forward as a Linux Foundation and we're going to be behind open source AI.
14:20
So we made a choice that AI should be open source. We will need to define it better, but basically we will not allow, right, as a community, single company, group of, you know, oligarchy of companies to hold the kind of secrets in a black box and in an unknown fashion tell us what to do with it.
14:39
It goes contrary to all the open source ethos and fortunately, IBM is kind of firmly behind this line of thinking. didn't follow IBM's history, we contributed Linux to the community which made data centers and commodity big data possible which led to basically the rise of big data which led to the rise of ML and AI.
15:00
And same thing happened with Java. And I think we can do this again with open source AI. But obviously Linux foundation is the biggest body in the industry and I am very excited that we're gonna make a lot of progress.
15:12
If you want to work with us, we can invite even folks who are not members of the Linux foundation. If you have specific interest in open source AI, we have this work streams as I outlined before, data models, applications and openness, and we need help, so please find me.
15:29
So this is another example where we apply open source AI to science, this is a hackathon hosted by Chansey Kerberk initiative just recently and multiple teams used this technologies to basically access bibliographical data about science and help advance science with open source tools.
15:49
This is just a reminder there is more to life than selling stuff on the internet. IBM research is a global organization so extremely hard problems and I think open source AI is as important for human guide as for commerce.
16:02
Again, if you're interested in science, reach out to me. So I'll just briefly touch upon this, something we don't need to talk about. LLMs are much more than mechanical Turk automation. We will see an actual digital transformation, that's another reason we didn't see the talk about business deployment yet because LLMs will not only automate low level workers as mechanical Turk did, LLMs will actually replace whole layers of middle managers because middle managers are modems, routers, the network nodes, they connect top level strategy to low level execution and because LLMs are actually much more powerful as human like workers.
16:47
There will be a new kind of worker, an engineer with a human in the loop empowered by this tool. I also propose that the effect of this will be digital transformation 2 .0, which I think will be an actual digital transformation to actually transform the makeup of companies.
17:05
Again, we need open source AI to make sure this is fair, this is transparent, because actual workforce will be redone based on this. So we need open source at all levels, because it will lead to decisions leading to actual digital transformation, finally, which will arrive.
17:21
Another topic I want to touch upon, industrial AI, we need all kinds of elements of life, operated like machines. We're not talking about this yet. We have charts, we have text, images, everybody shows cute pictures.
17:34
I mean, show me how to operate a tractor, if you don't know how to do it, you're going to capsize. There is a lot of hard things in industry which are waiting. And three things are required in industry, ownership.
17:46
You need to own your model. Your model needs to be small. If you want to deploy it in a tractor, it should not tell you the meaning of life. It should not reason with you about political issues. It should operate a tractor.
17:57
It should assemble a car. And these LLMs are coming. We may call them small specialized models or small specialized agents. Again, these things need to be open source so the businesses can adopt them and use them.
18:09
Again, this is Clement DeLang tweeting about the future where enterprise and AI will be small models. And we need multiple bodies in AI to collaborate. Finally, I want to invite you all to this conference which I run for 10 years.
18:23
It's in Auckland. It's this amazing place which looks like nothing you've seen that's an old Masonic temple. And we're going to have a LLM training Monday, November 13th for developers. Test driven LLM development.
18:35
Hands on. Again, the training like nothing you've seen before. We do the best training for our conferences once a year, just one. It's not a repeatable event. It's a bespoke training. And then we have two days of technical talks.
18:48
So Chris Lautner, the creator of LLVM, Swift and new language called Moja, which will speed up AI by hundreds of times. He's going to be there and multiple leaders in LLMs. But also software engineering, distributed systems, so everything H2 again is very good at.
19:04
So I think we're kind of aligned in this context. So there's a code here H2 30 for 30% off all the passes. It's only valid like a couple of days. Use it again. Reach out to me if you have questions about this conference.
19:16
I'll be around. And that's all I have.