Artificial Intelligence And Machine Learning For Optimizing Devops, It Operations, And Business
EMA Top 3 Report and Decision Guide for Enterprise
The Disruptive Impact Of Artificial Intelligence And Machine Learning On Devops, It Operations, And Business
Enterprise Management Associates (EMA) research shows that leveraging artificial intelligence and machine learning (AI/ML) for DevOps, IT operations management, and business management is the top priority for enterprises in 2018 and beyond. AI/ML brings data-driven intelligence to DevOps, IT operations, and the enterprise to optimize processes, recognize relevant trends, proactively prevent issues, rapidly detect and resolve problems, and enable human staff to make optimal and fact-driven decisions.
Guidance for AI/ML Product Decisions in DevOps, IT Ops, and Business
This EMA “Top 3 Decision Guide for Artificial Intelligence and Machine Learning in DevOps, IT Operations, and Business” provides guidance for enterprises seeking to optimally leverage today’s AI/ML capabilities, depending on their individual situation and priorities.
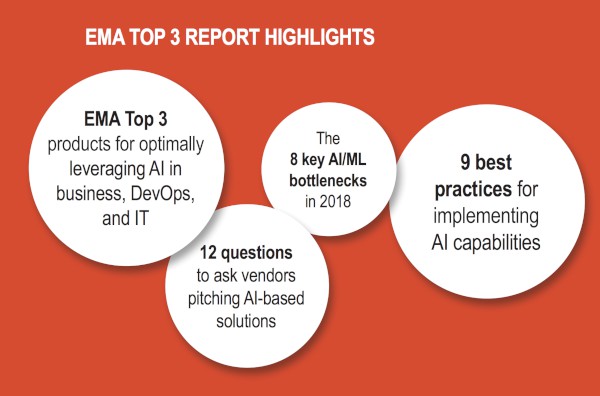
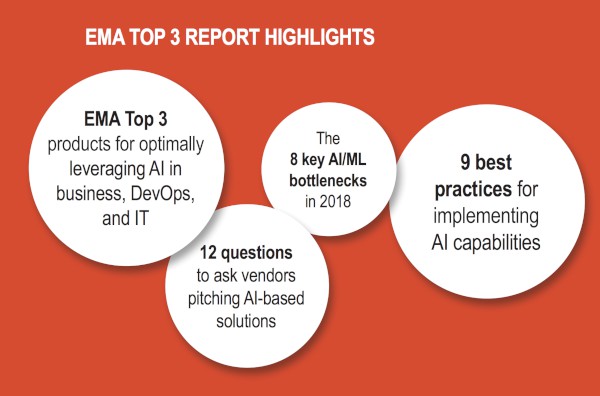
Why AI/ML is Disrupting Every Industry
The disruptive character of AI originates from the core expectation of AI/ML technologies enabling enterprises to make optimal data-driven decisions that are aligned with their corporate strategy, immediate priorities, and compliance obligations.
Enhancing human capabilities through AI: Complexity is the most critical challenge for employees in DevOps, IT operations, and business. Decision makers are faced with a rapidly growing number of data sources, technology options, and competitive requirements. Optimal decision-making requires the ability to quickly identify how all of the different data sources can be put together into one big picture that shows the outcomes of alternative courses of action. Artificial intelligence can act as this “complexity reducer” by identifying potentially relevant trends and anomalies in these vast bodies of data and by revising its output based on human feedback and past project outcomes.
AI-driven automation: AI-driven automation can eliminate simple repetitive tasks to enable staff to focus on activities where human intuition, creativity, skills, and situational awareness are critical. AI-driven solutions that best include humans into their decision and automation workflows have been significantly more successful in the market compared to solutions focusing on a black box approach to AI-driven automation.
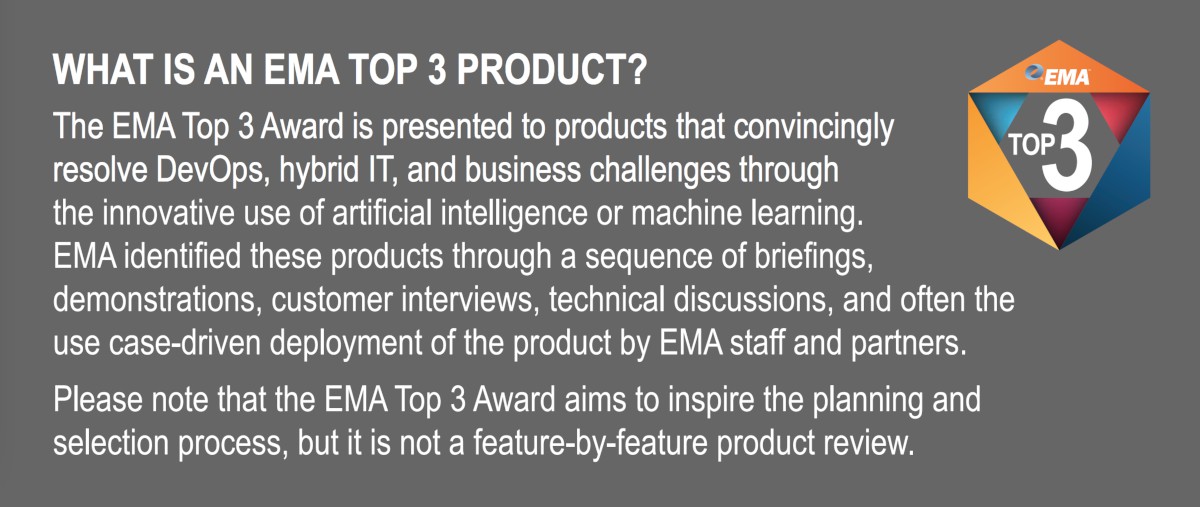
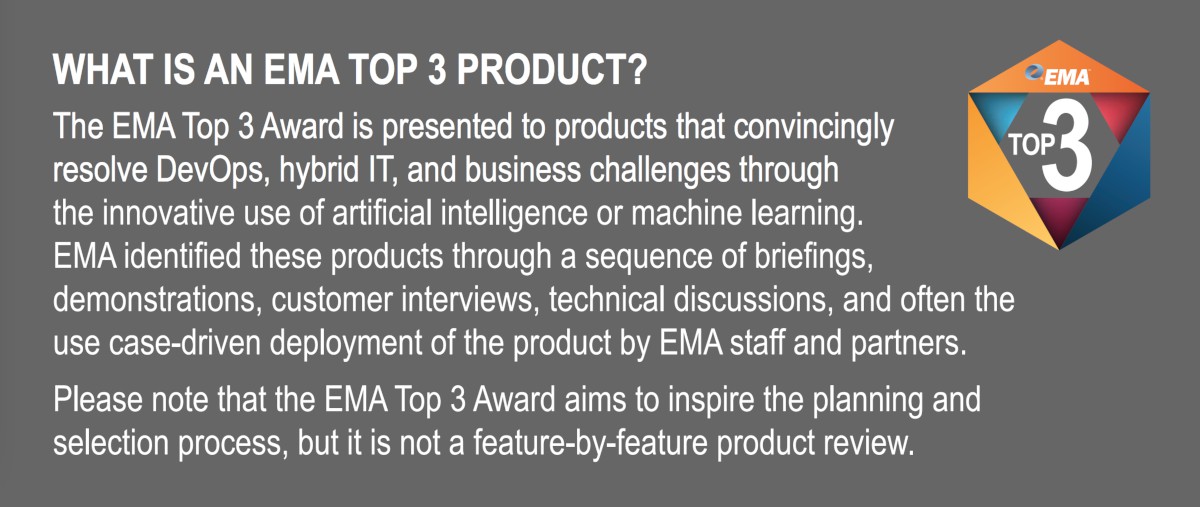
AI BOOST FOR DIGITAL ATTACKERS
EMA’s concept of the digital attacker aims to optimize an enterprise’s ability to deliver customer value faster, cheaper, and at better quality compared to the competition. Therefore, enterprises need to minimize operational costs to be able to focus most of their resources, skills, and capabilities on increasing customer value, instead of spending often 50 percent of their budget on “keeping the lights on.” AI-driven DevOps, IT operations, and business systems can unclog this bottleneck by showing human decision makers how to optimize and automate their current infrastructure without increasing operational risk, how to accelerate the DevOps pipeline while increasing release quality and decreasing cost, and how to provide intelligent solutions for business users to maximize their time spent on essential activities that require human judgement, creativity, general knowledge, and empathy.
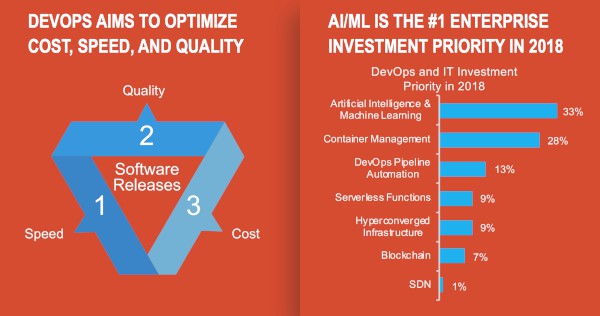
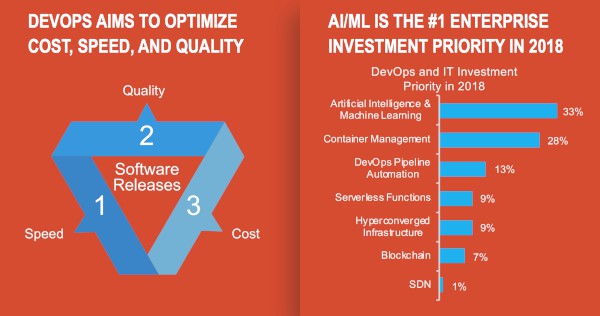
The Four Core Capabilities of AI
AI can solve the unsolvable in business, DevOps, and IT operations due to four core capabilities:
- Complete tasks without explicitly coded instructions
- Receive reliable results for cases that were not explicitly included in the training set of example
- Rapidly identify relevant information in vast bodies of data
- Increase prediction quality based on continuous feedback
The 1993 demo of LeCun’s first CNN
Note that none of these elements is new or revolutionary in 2018. Yann LeCun showed in 1993 how his AI software, the first so-called convolutional neural network (CNN), was able to reliably recognize handwritten numbers without having received any rules or instructions, but was fed with tens of thousands of examples for each number instead. LeCun’s CNN even worked under difficult conditions, such as sloppy handwriting, dirt on the paper, or a faulty pen. LeCun’s CNN still is the foundation for many modern AI/ML products, such as the self-driving car, language recognition, or calculating mortgage risk.
Complexity Reduction as the Key Value Proposition of AI
AI/ML models can solve tremendously complex challenges without a single line of code, simply by ingesting example data. All of the EMA Top 3 vendors in DevOps, IT Ops, and business are leveraging AI/ML exactly for this purpose, providing human operators with predictions and categorizations derived from large and often seemingly unrelated sources of structured and unstructured data.
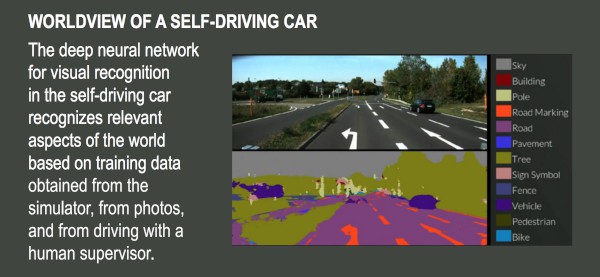
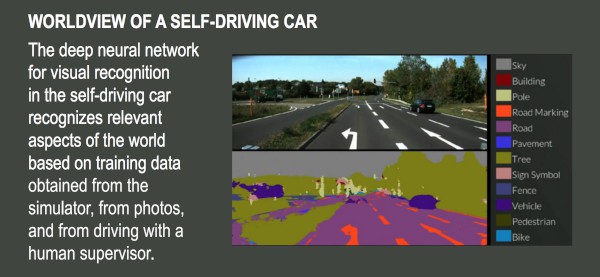
REALISTIC EXPECTATIONS ARE CRITICAL
There is a term in AI research called the “AI Winter” that describes a period of near standstill in terms of AI research. The AI Winter lasted from the late 1980s until the early 2010s and was triggered by a large degree of hype and exaggeration around the AI topic, with little corresponding research progress to show.
Today, companies are running the risk of slipping into another period of exaggerated expectations due to AI companies fueling imaginations by painting images of systems with truly human-like intelligence. The term “AI” implies that this technology enables machines to purposefully act in the same way a human would. This report demonstrates that humans have not come any closer to human-like machines since LeCun’s breakthrough in 1993.
This EMA Top 3 report is all about spreading excitement around the real capabilities that AI/ML can deliver today and in the immediate future. These capabilities are tremendously powerful and can change business models and industries. To prevent another AI Winter, vendors and enterprises must develop a common understanding of expectations around AI/ML technologies. As the following three examples show, AI/ML enables users to solve problems that formerly were impossible to solve.
The Key to Understanding the AI Discussion
To successfully leverage AI/ML for business, enterprises must let go of the idea of developing Data, the android from “Star Trek: Next Generation,” for optimizing data centers or automating software testing. However, if they manage to curb exaggerated expectations, AI/ML already has the capabilities required to disrupt any industry and dramatically increase human productivity.
The basics of AI/ML today are simple and easy to understand. Instead of coding IF-statements, creators simply provide an algorithm with a large number of training documents that show the connection between features (independent variables) and results (dependent variables) in many different ways. They need massive compute capabilities to enable the algorithm to minimize prediction errors.
Once the model is fully trained, it can make predictions based on new input that is similar in character to the training material and users can expect predications at a comparable level of reliability. This level of reliability can be higher than that of a human worker, but keep in mind that the AI/ML model does not have any human-like capabilities to “fill in gaps” when dealing with input variables that are different from the training set. In this case, the model might not produce any results, or it may produce dramatically wrong results without having the capability to notice them as such.
The following examples show tremendous business impact by leveraging AI/ ML in a realistic manner, instead of aspiring to human-like decision making.