Machine Learning: Considerations for Fairly and Transparently Expanding Access to Credit
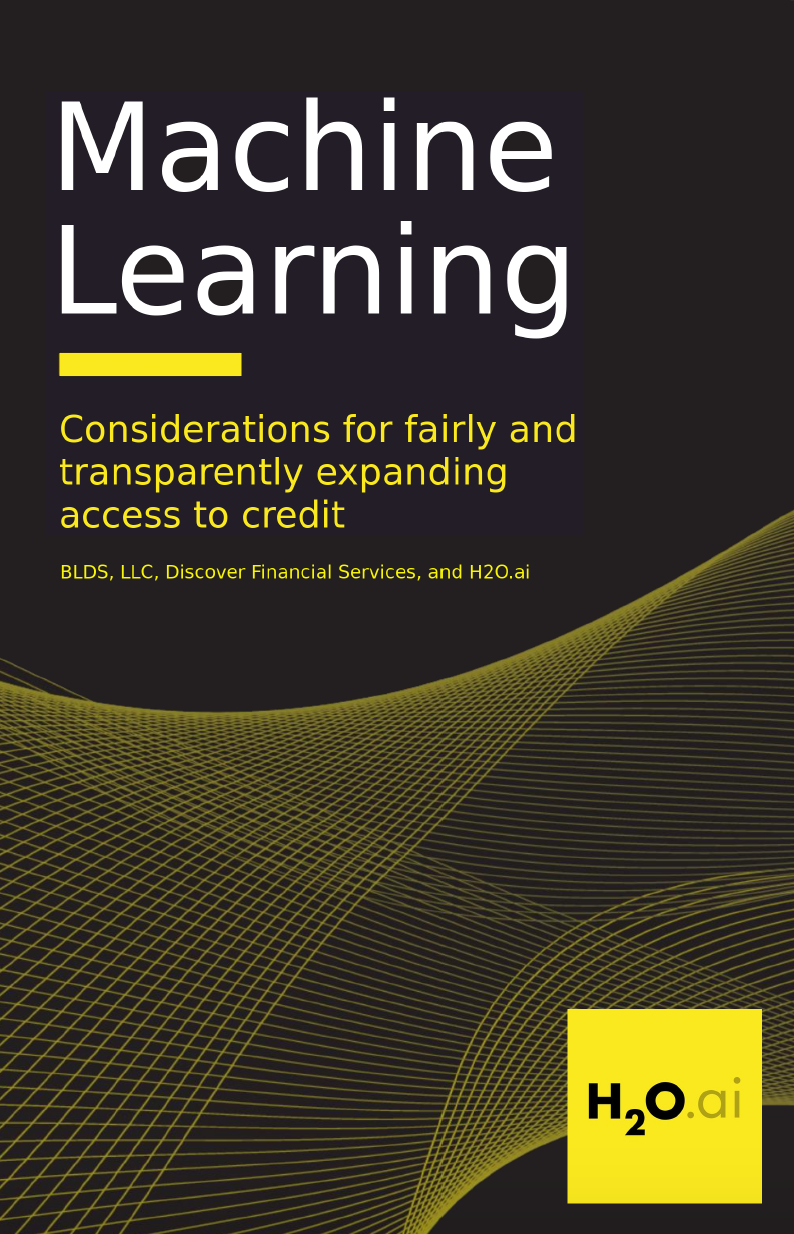
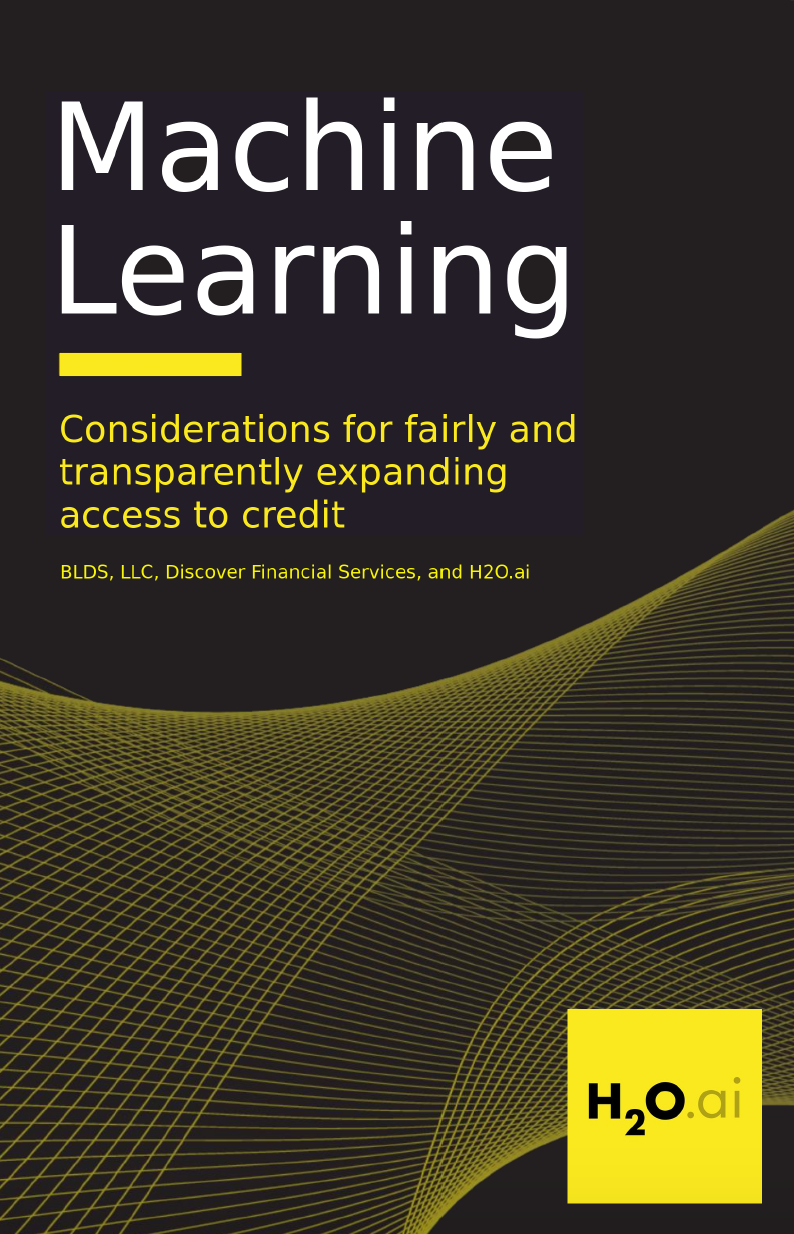
Experts from Discover Financial Services, H2O.ai and BLDS came together to create a common framework and set of definitions of key ML concepts, so that market participants, regulators, policymakers, and other stakeholders can all speak the same language. There has been long evidence and cases of discrimination in access to capital and lending opportunities in financial services. With the introduction of machine learning, major concerns have arisen in regards to perpetuating historical human bias and in explaining credit decisions to consumers.
In this paper, we aim to define discrimination and interpretability in the context of fair lending and machine learning, and then to discuss important considerations and opportunities for machine learning in the equitable and transparent use of algorithms to underwrite credit lending decisions.
Contributors:
Steve Dickerson, SVP, Chief Analytics Officer
Discover Financial Services
Patrick Haggerty, Director & Senior Counsel
Discover Financial Services
Patrick Hall, Advisory Consultant
H2O.ai
Benjamin Cox, Director of Product Marketing
H2O.ai
Arjun Ravi Kannan, Senior Manager, Emerging Capabilities
Discover Financial Services
Raghu Kulkarni, Vice President - Data Science
Discover Financial Services
Kate Prochaska, Senior Counsel & Director, Regulatory Policy
Discover Financial Services
Nicholas Schmidt, AI Practice Leader
BLDS, LLC.
Melanie Wiwczaroski, Director, Enterprise Fair Banking
Discover Financial Services