Explainable AI
Increasing transparency, accountability, and trustworthiness in AI.
Explaining Explainable AI
Explainable AI is in the news, and for good reason. Financial services companies have cited the ability to explain AI-based decisions as one of the critical roadblocks to further adoption of AI for their industry.
Transparency, accountability, and trustworthiness of data-driven decision support systems based on AI and machine learning, or more traditional statistical or rule-based approaches, are serious regulatory mandates in banking, insurance, healthcare, and other industries.
From pertinent regulations to increasing customer trust, data scientists and business decision makers need to understand the challenges and opportunities with explainable AI. Being able to explain and trust the outcome of an AI-driven business decision is now a crucial aspect of the data science journey. H2O.ai is committed to driving innovation and solutions that make explainable AI part of your company’s AI success story.
University of Alabama at Birmingham’s Data Science Club recently interviewed H2O.ai’s Patrick Hall – watch their podcast on Machine Learning Interpretability, here.
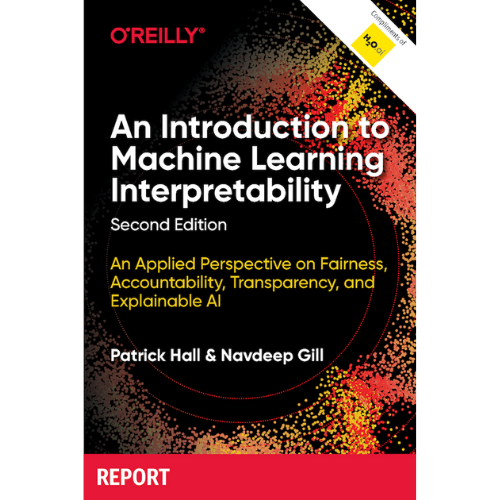
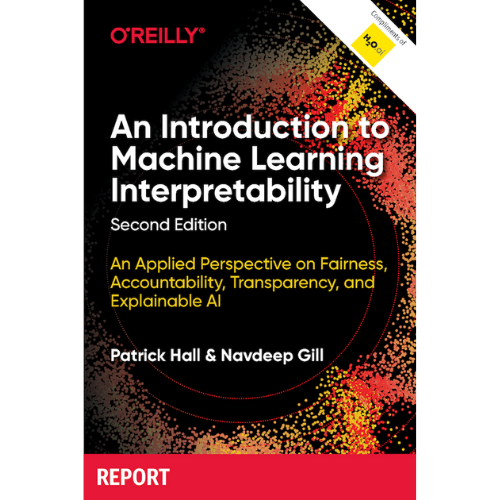
An Introduction to Machine Learning Interpretability Second Edition
Download this book to learn to make the most of recent and disruptive breakthroughs in debugging, explainability, fairness, and interpretability techniques for machine learning. In this report you’ll find:
- Definitions and examples
- Social and Commercial Motivations for Machine Learning
- A Machine Learning Interpretability Taxonomy for Applied Practitioners
- Common Interpretability Techniques
- Limitations and Precautions
- Testing Interpretability and Fairness
- Machine Learning Interpretability in Action
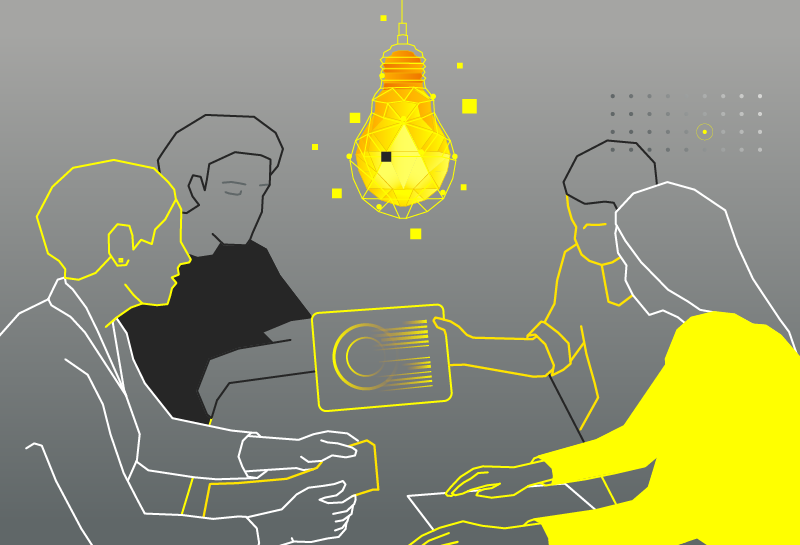
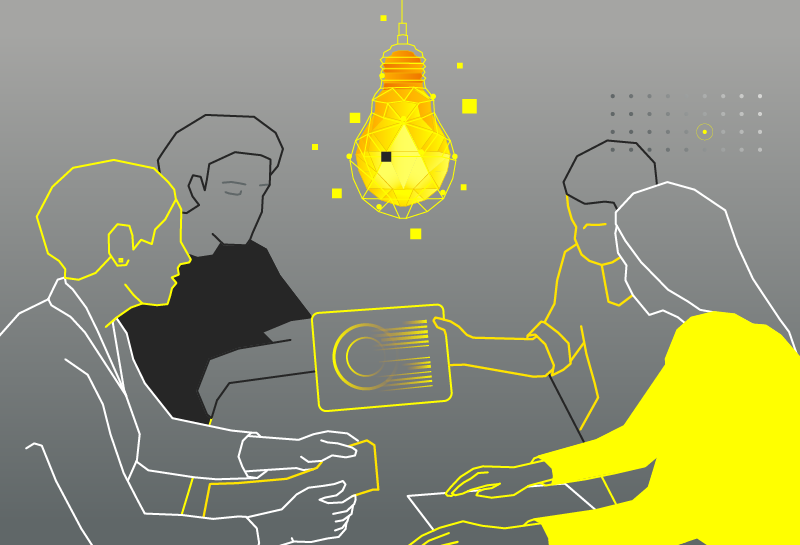
What is Your AI Thinking?
In this three part blog series, understand the challenges and opportunities in the realm of explaining AI, and how technology can help build trust in AI.
How to Get Explainable AI Today
H2O Driverless AI does explainable AI today with its machine learning interpretability (MLI) module. This capability in H2O Driverless AI employs a unique combination of techniques and methodologies, such as LIME, Shapley, surrogate decision trees, partial dependence and more, in an interactive dashboard to explain the results of both Driverless AI models and external models.
In addition, the auto documentation (AutoDoc) capability of Driverless AI provides transparency and an audit trail for Driverless AI models by generating a single document with all relevant data analysis, modeling, and explanatory results. This document helps data scientists save time in documenting the model, and can be given to a business person or even model validators to increase understanding and trust in Driverless AI models.
Play the video to watch MLI in action.