Time Series
Time-Series in H2O Driverless AI
Overview
H2O Driverless AI delivers superior time series capabilities to optimize almost any prediction time window, incorporate data from numerous predictors, handle structured character data and high-cardinality categorical variables, and handle gaps in time series data and other missing values.
Key Capabilities of Time-Series in Driverless AI
- Automatic handling of time groups
- Robust time-series validation
- Time-series specific feature engineering
- Integration with existing feature engine
- Automatic pipelines generation
Driverless AI handles various time groups automatically, for example, different stores and departments.
Time-series in Driverless AI offers robust time-series validation. It uses past information only implying there is no data leakage - it accounts for gaps and forecast horizon.
Date features like day of week, day of month etc. AutoRegressive features like optimal lag and lag-features interaction Different types of exponentially weighted moving averages Aggregation of past information (different time groups and time intervals) Target transformations and differentiation
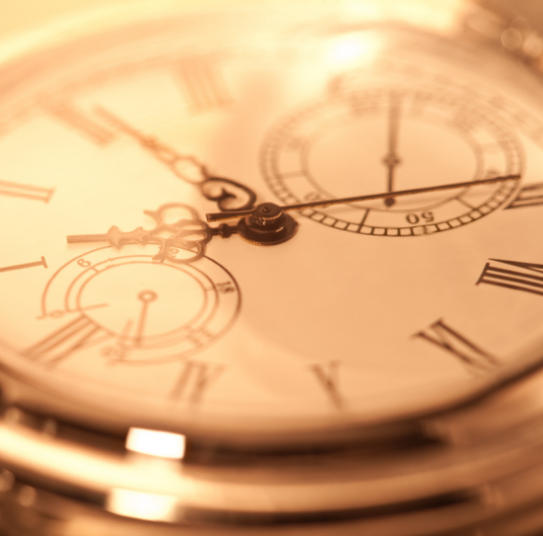