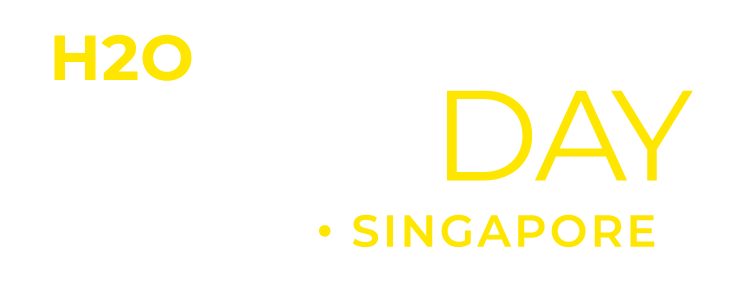
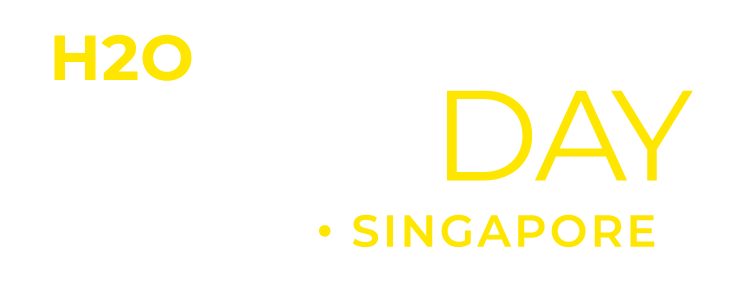
GenAI Training + Workshops - Singapore 2023
Speaker Bio
Shivam Bansal | Director, Customer Data Science - Kaggle Grandmaster
Shivam Bansal is a renowned data science leader, currently serving as the Director of Data Science at H2O.ai. He boasts the prestigious title of a 3x Kaggle Grandmaster, exemplifying his expertise in datasets, notebooks, and discussions, with top rankings in each category. Shivam's prowess extends to winning five Kaggle competitions and securing ten Kaggle awards, along with victories in several offline AI and data science contests. Academically distinguished, he graduated as the Valedictorian from the National University of Singapore with a Master's in Science, Analytics.
His career spans diverse industries including banking, finance, insurance, retail, healthcare, and manufacturing, specializing in AI solution building for varied business challenges. He excels in machine learning, AI application, product development, and full-stack engineering. Shivam has a proven track record in leading teams, AI product and project management, and supporting pre-sales and customer success teams in AI and data science. His notable achievements include driving C-level engagements and business transformation with a focus on quantifying business goals into actionable data metrics.
Chun Ming Lee | Senior Principal Data Scientist
Chun Ming Lee is a seasoned data scientist currently holding the title of Senior Principal Data Scientist at H2O.ai. An acclaimed Kaggle Grandmaster, Lee has demonstrated exceptional skill in data science competitions, securing 1st place in both the 2018 Jigsaw Toxic Comments and 2020 Jigsaw Multilingual competitions. He also achieved 2nd place in the 2021 Coleridge Initiative Show US the Data and 2022 Feedback Prize competitions.
With a robust academic background, Lee holds a Master of Business Administration from London Business School and a MS in Information Systems Management from Carnegie Mellon University, graduating with highest distinction. His professional journey includes roles at Barclays Capital, NEC Corporation, and Orbis Investments, displaying a diverse expertise in both technical and business domains. Lee's proficiency extends to fluency in Japanese, certified by the Japanese Language Proficiency Test Level 1, and he has passed all three levels of the CFA Program.
Genevieve Richards | Data Scientist
Genevieve Richards is a Data Scientist at H2O.ai, showcasing a rich history in the financial services industry. She is skilled in Scrum, R, Python, Agile Methodologies, and Customer Service. Genevieve's strong engineering background is underpinned by a Bachelor of Information Technology in Computer Science from QUT (Queensland University of Technology). At H2O.ai, she has been instrumental in various roles, including her current position as a Customer Data Scientist.
Her work at Commonwealth Bank in the AI Labs team focused on using AI for social good, particularly in detecting abuse in transaction descriptions. Genevieve's academic achievements include a Bachelor of Arts in Linguistics and Psychology from The University of Queensland, and she has been recognized on the Dean's Academic Excellence List at QUT. Her certifications include the Foundations of Behavioural Data Science for Business from the University of Sydney and a Certified Scrum Master from Scrum Alliance.
Vishal Sharma, PhD | Data Science Lead
Vishal Sharma, PhD, is a leading data scientist with a strong academic and professional background. He earned his PhD in Electrical and Computer Engineering from the National University of Singapore, focusing his thesis on electricity price time series forecasting using recurrent neural network approaches. Vishal is currently the Data Science Lead in Solution Engineering at H2O.ai and an Adjunct Faculty member at NUS, specializing in Gen AI. His expertise lies in deep learning neural networks, time series forecasting, and Natural Language Processing (NLP). With a keen interest in applying ML/deep learning models to real-world commercial projects, Vishal has experience in the industrialization of AI, driving end-to-end machine learning solutions. His professional journey includes significant roles at DBS Bank as VP and Senior Data Scientist, where he led AI projects and developed credit risk assessment models, and at Fujitsu Singapore, where he focused on developing recommendation systems. Vishal's skills are complemented by his quantitative modeling and time series analysis expertise.
Timothy Lam | Enterprise Solutions Engineer, Data Scientist
Timothy CL Lam is a Client Facing Data Scientist at H2O.ai, with over 8 years of experience in the Asia Pacific region. He is a trilingual professional, certified in solutions from AWS, Microsoft, and Databricks. Specializing in Python, R, and GUI-based software, Timothy has developed industry-specific prototypes and proofs of concept. He is also skilled in generative AI, machine learning, and deep learning. Previously, he worked at Alteryx as a Solutions Engineer and at IBM in Business Analytics & Watson Platform Presales.
Timothy holds a Bachelor’s degree in Business Administration from The Chinese University of Hong Kong and has completed an exchange in Mathematical Statistics and Probability at IÉSEG School of Management. His expertise includes graph databases and data mining, and he is proficient in Cantonese and English.
Read the Full Transcript
00:19
Thank you for the great insightful talk. Hi everyone, my name is Shivam Mansell. I'll be taking through to the next session, which is the Hands -On Advanced Workshop. Before that, I would like to really thank Sri again.
00:33
And can we all have a very warm round of applause for Sri for the great insightful session. All right, so let's get started with the Advanced GenEI LLM Hands -On Training Workshop as well as certification.
00:54
I'll be one of the instructor along with my colleagues. So I would like to invite Vishal, Chunming, Jen, and Timothy on the stage. All of us will be taking through a series of highly packed agenda, which will be focused on foundations of GenEI, LLM.
01:10
We'll be doing a lot of hands -on activity. We'll be creating our own RAG solutions. We'll be creating our own GenEI apps. We'll be fine -tuning the models. We'll be doing data prep as well as evaluation.
01:22
So please welcome Vishal, Jen, Chunming, Timothy, and myself, Shivam. Okay, so let's get started. Before I start, there are just five great takeaways I would like to mention that all of you will have after this end of the training.
01:46
Why they are great? Because all the labs, all the trainings have been structured in a way that you really get very hands -on understanding of the foundations of GenEI as well as you are able to apply that understanding of large language models.
01:58
RAG, we heard RAG. quite a lot in Agus presentation. Thanks Agus for making a lot of insightful statements around RAG as well as evaluation, eval GPT, why it matters and why it is necessary for organizations, data scientists to use it.
02:13
AI apps, we saw a couple of AI applications, GenAI apps in the demo from Shree and tuning. We had tuning quite a lot. There was a fine tuning component and today's lab will also focus on tuning your own model, fine tuning your own model with a domain, with your own data to make my GPT.
02:33
So everyone of you can have your own GPT. So like I said, this is a hands -on session, hands -on workshop session and To access the platform, I guess we have also given some instruction, prerequisites are on the table.
02:50
So very first lab focuses more on the foundations and the ecosystem. But I suggest if you guys can also try logging into the platform, take a look at signing in with your email IDs or the user IDs that have been given to you.
03:05
We also have a lot of lab assistants throughout this room as well as the other room who are watching us on the Zoom. So if there are any queries, any questions, please feel free to raise your hand and just take assistance from our lab assistant people and they will be happy to assist.
03:22
There is also a public Wi -Fi. So the Wi -Fi password details, let me just show it here. So the Wi -Fi is NTUC and password is I Love NTUC all small. You all can log in. Yeah, I guess it is NTUC public.
04:12
All right, so let's kickstart the first lab. Like I said, because in the first lab, we also heard about ecosystems from Shree stock. I will be talking about what is a typical Genia ecosystem, what organizations should consider while creating a Genia ecosystem.
04:28
Again, as you can see on the top, the whole agenda is backed towards various activities. We are starting with this crash course on foundations of Genia LLM various terms related to RAG related to LLM fine tuning.
04:42
After that, we will be talking about the that it will be all hands on with lab one, lab two and three, four onwards. So let's get started. So when we talk about foundations of a JNA ecosystem, what essentially we mean?
04:55
So it all starts with datasets. Data sets can be in the form of documents. This is the, by the way, the same diagram Shree described, but I would like to explain it in more detail and I would like to connect it how all of you will be able to create your own ecosystem with this.
05:08
So it all starts with datasets. Data sets can be the form of structured data and structured data. And when we talk about unstructured data, it could be various types of documents, PDFs, audio files, videos, web pages, et cetera.
05:21
Now this data needs to be consumed in some way. This data needs to be accessed to make the use of from the JNAI perspective. And that's where the first step about data preparation comes into picture where we need some form of data massaging, data cleansing, and that data needs to be processed so that it can go to the next step.
05:41
Now there are two pathways. One, this data can be used to convert question answer pairs. So let's say there are reports, files. Those files can be converted into tabular data, which are question answers.
05:54
One column becomes a question instruction. The other becomes a response, which is an answer. And the other type of data processing is about creating embeddings. Like you have all the text, all the documents, it needs to be parsed.
06:07
It needs to be extracted from those document. It needs to be chunked so that a long text becomes portions of the text. It needs to be indexed. And then embeddings needs to be created. Just to mention for the people who are non -technical, embeddings is the numerical representation of text.
06:24
It essentially means if there is a sentence, which is a sequence of words, how it will appear in an n -dimensional vector space, n -dimensional mathematical space. So we need to convert that data to embeddings with this step.
06:37
Once we have data prepared, it can be passed to a fine tuning engine. Now there are many open source models available, but you may want to have your own model. So for that perspective, you would do some form of fine tuning.
06:51
You would take an open source model, fine tune it with your own question answer pairs that you have got in the data preparation step. And then you will get myGPT, custom GPT. At the same time, prompt tuning is also important.
07:04
Prompt is the new IP, which we also mentioned in the keynote. Prompts are essentially your questionnaires, your inputs to the LLM models against which any output could be generated. So that prompt also needs to be tuned.
07:18
The reason being LLM may be generic, LLM may be fine tuned, but the question is how you are using it, how you are consuming it. That becomes an IP that becomes core to the organization. At the same time, if I talk about the other flow, if we have embeddings, that embeddings needs to be processed by some LLM workers, some large language models, plugins or modules with the goal of how these embeddings can be generated.
07:43
be used in a way where they can respond to a user query. They can respond to any questions given by the users. And the result of these, like we mentioned, is MyGPT. You can have ShivamGPT. You can have NTUCGPT, H2GPT we already have built.
08:00
And we have built that using the same process, data to fine tuning, and then create MyGPT. Now this MyGPT can be accessed via LLMOps via APIs. It can be exposed to external systems. And your Google Sheets, your Zoom meeting invites, your teams can start leveraging custom GPT for the purpose of text generation and making some alerts based on the content.
08:26
As well as if you look at the bottom side, RAG, retrieval augmented generation, is a very popular term in this LLM space. It essentially means we need a system which can leverage your private data, which can leverage your documents.
08:42
and cannot just answer generic questions, but information which is tailored to your organization, information that is present in those documents that is extracted from this RAG system. And today's first lab is focused on that and we'll do a deep dive.
08:57
We heard a lot about eval. Eval is really important. There are a bunch of open source models and in the community as it is growing, a lot of open source models keeps on coming. They need to be evaluated.
09:08
They need to be evaluated, not just, which is more accurate, but whose hallucination is less. Which model works well in solving math question? Which model works well in troubleshooting questions? So there is a different type of eval that goes into who this ecosystem.
09:27
And now if you talk about the last layer, consumption layer, it is the user layer. Users need to access. They need to connect to these RAG systems to pass the prompts, to pass their instruction, to pass their questions.
09:38
And basically they talk to their data. They talk to their documents. They do document chat, document conversions. And the other example, other way which this myGPT RAG can be used is integrating them in different applications.
09:53
Like we saw a couple of examples, say contact center may use RAG and create their own application. At the same time, so this is the ground layer, which is Janiyia layer. At the same time, if we talk about an ecosystem, we should not also forget the roots, which is the predictive ML, which is the traditional ML, where data sets can be passed to several engines.
10:15
It could be open source, auto ML, it could be any private model, model building tool. You can train your supervised model. You can deploy them in ML ops. You can create custom applications. But now with the arrival of custom GPT Janiyia ecosystem, these LLMs can be integrated.
10:33
And the outcome will be creating Janiyia apps. And apps essentially could be seen as a different term for a use case. Every team, every use case can help. their own app with the power of both predictive AI and generative AI.
10:45
So the top layer is predictive AI, the bottom layer is generative AI. And this is a glimpse of how an organization should create a gen AI ecosystem because it really covers end to end picture. It really covers various aspects.
11:00
And H2 .ai also has created their own ecosystem based on the same idea. Like if you talk about various tools to create models, we have driverless AI, hydrogen torch for fine tuning LLM studio for data preparation data studio for app creation app studio.
11:19
And this bottom box that we see where there are different components of vector DB LLM workers, eval studio and an LLM integrated this whole box essentially becomes a rag system from which users can make questions queries and get their answers.
11:35
So every perspective, like I said, every aspect, be it predictive ML, be it data prep, fine tuning, drag, JNAI apps, integration, evaluations, all of these are covered when we talk about in this JNAI ecosystem.
11:47
And this really essentially completes the entire picture why what is really the main components of a particular LLM ecosystem and how organization can leverage all the various activities around LLMs as well as predictive AI.
12:05
And to mention what we are going to do today, all the labs have been structured in a way that we will be covering all the perspective, all the aspects from the data preparation using a notebook style as well as the UI based methods, fine tuning similar in a notebook as well as through a UI, rag using a Jupyter notebook as well as UI.
12:26
We will be able to create one custom application in lab two where you will you all can talk to your LinkedIn profile and ask questions about give me a summary of my profile, give me some hashtags and we'll be able to deploy that app also.
12:39
So stay tuned for all these labs, which we'll be covering very shortly. So in summary, again, this is a JNAI ecosystem. What is really a groundwork? What is really a foundation to start any LLM JNAI activity?
12:55
Okay. So I'll take a pause. I can see a lot of hands on activity. People are trying to log in, which is great. And I think it's good because I am going to maybe take another 10 minutes to explain various concepts again for the benefit for users who have no familiarity, who may be coming from different domains.
13:17
Some foundations of LLM and I'll be using just one slide promise to talk about LLM, so rag, JNAI apps. And in the meantime, you guys are trying to log in. So if you continue doing that, that will be great.
13:31
So a lot of technical terms, a lot of. A lot of topics that you have came across since morning. So I will take maybe a few minutes just to walk through what these are because these are really the foundations, fundamentals and I promise these will be very beneficial in the labs that you will go through today as well as going forward.
13:51
So LLMs, let's start with the most important piece, large language model. So what exactly is a large language model? Very deep neural network that have been trained, that have been trained on vast amount of text data that are capable of generating highly cohesive text and text generation, content generation is not only purpose of these LLMs but also various other outputs can be extracted.
14:15
Say content generation, I mentioned one, summarization, RAG, information retrieval, a lot of NLP tasks such as extracting the sentiment, even performing traditional NLP problems like we want to classify it and using LLMs in a smart way.
14:34
And why they are large as the name itself suggests, because they are trained on very large data, maybe petabytes of data, maybe all of the internet, all the Wikipedia. They typically have very large architectures, very large deep neural networks, and they need very large compute, large GPUs, large memories to really hold all those weights to do the training.
14:56
And if you talk about training, the training of these models have been done with one clear objective. If you show a model all the text, but we hide some tokens within the text and let model figure out what could be the right keyword given the context nearby, given the next near surrounding words and can the model predict what is the next token, we call it as next token prediction, as well as in some of the architecture, there's a concept of mask language modeling, where we mask some of the keywords, we don't show some of the keywords to a model, but show all the other text, all the examples and model tries to learn what could be the middle text.
15:32
Now imagine if this exercise is done over petabytes of data or all the internet or very large architectures, what is the result? Result is there is an LLM which starts to learn a lot of patterns, a lot of structure, a lot of representation about words, how they are connected, how they can be, what is the relationship between them?
15:52
And essentially that can be used further for various domains, various specific sub tasks. So we call this as pre -training stage. So every LLM has a pre -training stage where the goal is provide all the large data, let model learn in an unsupervised way and the result is a base foundation model, which then can be taken to the next step.
16:13
So foundation model, which is the next topic is really like a base, the core as the name itself says, it's really a foundation of an LLM. And it is surprising to know the JNEI LLM got picked up since last one year or so, but the core architecture behind the foundation model is something which was really in 2017 in the paper, Attention is all you need.
16:37
The most important paper in the field of NLP and the architecture's name is Transformer Model. And this Transformer Model was something unique, something special because it was leveraging a concept of attention.
16:49
Now what is attention? Like if you talk about even this room, there may be multiple noises coming, but all of you are trying to listen to my voice. You are trying to pay attention to the most relevant context because I am speaking.
17:02
Exactly same thing happens in the case of LLMs, in the case of attention. When we show all these models, various texts, the model tries to figure out what is the most important section in the chunk, in the text, and tries to figure out the relationships around it.
17:19
So that's how attention works. And this attention is applied in a parallel way, in a multi -head fashion so that there is not just one learning. It is multiple variety of learning which can be adapted together, which can be blended together to really understand everything around the texts.
17:37
So foundation models, transformers have really been the core for everything. And why they have been picked up recently also because now fine tuning as a concept came up. When this LLM started getting popular since last one year, fine tuning started becoming one of the need for many organizations because when they saw success in a lot of foundations models around large language models, the idea was can we fine tune them, can we tweak them so that it answers only to our domain.
18:09
It answers only specific to in my style. Let's say we want to fine tune an LLM so that it answers in a tone of a financial analyst. So that is the concept of fine tuning. Like we provide some signals, we provide some additional training data, we provide specific things to a foundation model with the goal of creating my GPT, custom GPT.
18:30
And we are going to cover that. We are going to touch that in lab three where all of you will be able to create your own GPT as well. We touched upon data prep, data preparation ETL also. And why this is important because as researchers started exploring the field of fine tuning, as well as organizations started to explore the idea of JNAI LLMs for them, the most common problem was how to really consume the documents that they have.
18:54
How to really leverage the data that they have. So that data was required to be converted into this question and so peers, into instruction tuning pairs. And that's where the idea of data studio data preparation ETL for LLMs came up.
19:08
And this was also proven in some of the recent papers, very popular papers, textbooks are all you need Falcon paper tool LLM. These are a couple of papers that highlighted the idea of why good data is important, why data in a nice structure is important because they really tested with or without data cleansing, with or without data preparation.
19:29
without converting them to QAPS and the results had significant benefits after data was passed through a pre -processing engine. So here I'm really touching two points, two perspectives. When it is about documents, ETL essentially means convert those documents into a form which can be used by LLM models.
19:48
Plus, these documents also need to be processed for the purpose of parsing, chunking, indexing, embeddings. And if you talk about any text data, some of the considerations that all of the people need to make need to ensure that that data also is acceptable to use.
20:07
Any toxicity needs to be removed, any sensitive information, PII information needs to be removed. We need to ensure only quality text retain so that if we use the data in the next steps, those models also learn right things.
20:21
So, this is about ETLs and again we have a very dedicated lab, lab 4 .1 where you will go through how to perform some data preparation steps. And the last topic in this section is about evaluations and evaluations concept is again similar to what we mentioned earlier.
20:38
There are various points, there are various models, there could be models focused on generating something, some models focused on retrieving something, but the idea should be which model works best in what situation.
20:51
There could be many methods to evaluate, there could be metric based evaluation like let us evaluate a model's performance with against some of the human label text. Let's calculate metrics like blue score which is essentially just matching how much overlap is between the label data and the generated response.
21:10
Or the technique which is getting more popular is asking LLM to act as a judge. Like one LLM generates a response but we ask another LLM act as a evaluator, evaluate the response of this model and give me a score between 1 to 10.
21:26
And when we do this for all the response, all the prompts for various models, we basically get a leaderboard that is coming out from an LLM. So, LLM as a judge itself is one common idea. In fact, in our RAG system what we have done, we have added the concept of self -evaluation.
21:43
Once the LLM generates the response in the first phase, the next phase becomes we want to evaluate himself. So these techniques are really important essential. Again for the idea because LLMs are really different from a traditional machine learning model.
21:59
There is no concept of accuracy, which model is more accurate. Rather, the concept is which model works best in chat, which model works best in retrieval, which models works best in troubleshooting, etc.
22:10
So this thing really triggers organization to create their own eval systems. One more important point why this is important, which I mentioned in the trial. training slide, when we do pre -training, we are just passing all the data to the training.
22:25
And when we talk about consuming these, there is a high chance of leakage because the data has been trained only on the, all the examples that it has seen, but when we want to consume it in a different form, it will stick to something it has learned.
22:38
So in that case, it is important to have your own prompts as the evaluation because your, your prompts may be different than any other organization. That's why we need something like say, Eval GPT, where there can be a leaderboard of evaluations that come up, leaderboard of various models that come up against the prompts that the organization have set up themselves.
23:01
And this type of leaderboard can be blended with what is out there in the market, GPT4, or could be all the open source models that are coming. And the evaluation could be based on say something called ELO rating, where it is similar to a chess game.
23:16
where all players starts with same score, but as humans starts providing feedback like out of against this prompt, this model work best on the other prompt, the other model works best. Ultimately, the result is this model starts getting a ranking or a rating.
23:32
And ultimately, we get this type of a leaderboard, which model works best in what type of a scenario. So this is the concept of evaluation. And again, we have a lab. The last lab is all about evaluations.
23:45
So this was about LLMs. Next I would like to touch upon RAG because RAG is going to be very heavy focus for today's workshop also. So let me try to explain what is RAG essentially, what one step deeper in terms of what RAG essentially means and why it is becoming more popular.
24:05
So as the name itself suggests, retrieval augmented generation. So there are two components in this type of system. One is a retriever where the goal is to retrieve the right information from your documents and a generator, which is similar to an LLM given a question, given a prompt, what is the response.
24:22
So it all starts with leveraging the data that you have all the company data, private data. We need to pass it to a model which can convert this data into embeddings vectors. And we need to save it in a vector DB because vector DBs are different than traditional JDBC or other type of databases, but vector DBs are more optimized to store vectors and provide capabilities of vector search very quickly.
24:46
Because in the next step, we want to extract relevant chunks against user query. What are the relevant chunks? Because when user starts accessing this RAG system, they provide a question. Let's say what is the impact for Commonwealth Bank of Australia?
25:01
They provide this question. So one way could be with this question directly passed to LLM. In that case, the chances of hallucination will be very high. But in case of RAG system, it is passed through the same pipeline of embeddings.
25:16
user query is passed to an embeddings. It is checked against vector DB. What are the top K relevant chunks? So concept of re ranking or ranking most similar context is extracted. And then we get a relevant context, which could be say top five documents.
25:31
So now the user question becomes user question plus context. So instead of just passing in LLM one prompt, now we pass question plus context like what is the N -pad for CBA given these context. And now the response that we get is a very reliable response because we have really we are really showing model the grounding information, the context around it.
25:54
So this is essentially very high level view of what rag is. And again, today's lab one is all focused on that. We have also used this same principle to create enterprise H2o GPT enterprise a rag system where we can just connect to any type of data sources.
26:15
start asking questions on the fly and the model starts extracting the right information from the documents Also highlights the references also extract where this information came from and then users can use it programmatically in other ways Maybe connect this to other places Maybe in their existing applications or some or maybe just directly chat with it So talking about integrations, let me quickly show how some of the integrations that we have done One of the interesting one of the interesting Integration that we did was in Google sheets.
26:50
So this Google sheet that you see is automatically filled using two models One is a generative model where we just provided a prompt given a company name Let's say what's the website towards the industry give me a sales page.
27:03
Give me a data science pitch So this is a generative component, but we also connected it with a rack system where we provided a lot of documents About the annual reports of these companies and ask questions like give me annual revenue So if I show you the script around this, this is a this is a straightforward Python plus JavaScript type code which we integrated in Google sheet and we connected it to our Rack system with our API key as you can see if I just highlight and You can see some of our prompts.
27:35
We are directly configuring these prompts into Google sheets as a tool And now the moment we we add a new company here all this information automatically gets pulled in and To mention where where what is this URL basically this URL is Our rag URL.
27:52
So here you see this is a public Publicly available H2G PT and on the top I have this H2G PT E which is our rack system Which I just showcased about CB example. So if I show one of the collection So one of the collection is about annual reports of those companies from which that information is coming Fortune 500 company's annual report 2022.
28:18
So this it already contains all the companies, all their PDFs. And what this Google sheet is doing against a prompt is it is extracting this information. There is another LLM which is styling it. And then we have this auto filled GPT sheet ready.
28:32
Right. So this is one latest integration that we did. The other integration that I would like to quickly show is meeting AI application. So in lab two, you all of you will be able to create these type of applications.
28:45
This is one example that we are using internally where every online meeting that we are doing, every Zoom meeting, team meeting, it is directly connected to this rack system in which every conversation which is happening given after taking the consent, all the conversation is passed in the form of.
29:02
translation in the form of the transcription as well as the entire content is passed to the same H2G PTE. We have configured the prompt in order to get meeting summary, meeting action items, meeting transcript, a lot of hashtags.
29:16
If I just quickly show one of the tools that our team also helped build is called Document AI and one of our grandmaster mark. Some of the people Ryan from US, they were demoing it yesterday. And I integrated this video, which is a meeting and plugged it in this meeting system, which used RAG to generate responses around various categories.
29:40
Like what's the summary of this meeting? What's the action item? What are the hashtags? Give me a title. Also translate it for our multilingual user. Also give me a sentiment. So now every meeting which is happening on the other side, RAG is generating various types of results.
29:55
And behind the scene, it is similar type of a code that you see similar type of a playground H2G PTE, similar type of a RAG connection that we are doing. And again, all of you will be doing something similar in lab one.
30:08
One more topic I would like to touch upon is a rag is not just limited to documents, not just limited to PDFs. Which I just wrote a code where we can provide YouTube videos, YouTube URLs. This code performs the RAG directly on the videos.
30:33
It extracts the video, it downloads the video, converts it into frames, ingest those frames into the system. And then we can start asking questions on the video. It's not on the content of the video.
30:43
It's not on the subtitles. It's not on the information, but it is on the video which is essentially provided. So let me pick up my collection from which it got added. Let me start this job. you So, in the notebook which I provided, we provided a video, you are like process the video, it uploaded it into RAG system, it generated the embeddings and then now we are able to access it directly within this RAG UI.
31:23
So, in this collection, let's take a look at some of the chat. So, I was just playing around before this workshops where I was asking questions directly on the chat. So, one of my questions was how did the net profit sales change for each quarter?
31:38
Please also response in a tabular format. Let me just rerun this. And this answer, this response that came up, which is first extractive response and the other is more intelligent response where this information was also calculated like how much change happened.
31:55
And if I show you the references, there is no Next information, it is all image, it is all video frame from which this information got extracted. So this is a use case in which we extended the rag, not just on documents, not just on PDFs, but also on frames, so on, so on videos, also on various other unstructured data types to show these type of examples.
32:19
Let's see if it loads up. Okay, yep, it just came now. So maybe I can zoom in because it is loading slowly. So here you can see one of the first reference shows just a table, just the chart. So the most important reference, most important information it got from this frame and all these numbers, all these tables it extracted from the table.
32:49
There was no text, but rather this information. And we can even have a speaker talking about these. We can even ask questions directly on video frames. Video or images, then just documents. So this is about rag, rag integrations.
33:06
I would just like to touch upon two more topics very quickly before we jump into the hands -on. So prompt engineering, one of the other important considerations in GNI, everything that I was showing has a prompt against it, prompt is the questions.
33:21
This could be the questions which we ask as a user, or these could be preconfigured prompts against various expected outcomes. And prompt is the new IP like we are saying because the way we configure these prompts, the way we use some of the techniques, say, we ask them act as an evaluator, act as a business media person, and then generate a response.
33:41
Or you do prompt chaining, where essentially you chain multiple prompts together. So these ideas becomes your IP and against the prompt, you can get relevant results. We'll see a bit of prompt engineering in both lab one, lab two, as well as lab three.
33:58
related to prompts is guard railing, very important for enterprise JNEI. When we talk about LLMs as a service, LLMs to extract information from our private documents, we really need to have some rules so that LLM does not reveal what it is not supposed to, whatever it reveals all the sensitive confidential information.
34:19
That's where every LLMs also can be guarded with some of the guard rails which could be fact checking, which could be hallucination check, which could be sensitive info check, or it could be a set of system prompts where there could be a user prompt, but you also always append it with system prompt where you mentioned some of the instructions that you are not supposed to reveal something like this, rather you should double check.
34:42
So we will again see some grounding guard rails in lab one. There is a JNEI section. I would leave it to gen for lab two, and rather I will skip it to the next part. which is the hands -on training kickoff.
34:59
And I would like to invite Chun Ming for the first lab. Hey, morning, everyone. My name is Chun Ming. We're gonna switch gears from theory. So appreciate that you guys have been patient. For the rest of the day, we will have a series of labs where you'll be able to do hands -on.
35:19
The first, which I'll be conducting, will be on RAG, where you'll be uploading your LinkedIn profile, or if you don't have LinkedIn by chance, you can upload your resume, and then we'll tie it to LLM, and you'll be able to ask questions.
35:34
But okay, that's from a logistical standpoint. Wi -Fi, make sure you are able to access it. And the second thing, and this is the website, the platform that you will be using, genaitraining .hdo .ai.
35:50
You should have been emailed username password, where the username is along the lines of genai, well, XXX. If any of you don't have the ability to log in to the website, could you raise your hands? The lab assistants will be helping.
36:04
There's one back there, and there's one there, a couple there as well. So make sure you're able to log in to the website, and you should be seeing a landing page like this. If not, also raise your hands, and we'll try to help you.
36:24
And the other thing that is not for this lab, but it would be good for you to get started on, is there's another platform that you'll be using for a later lab called Aquarium. Could you go to Aquarium .hdo .ai, separately, and there'll be an option at the bottom of the screen to create new account, where you just enter in your email details, and you'll be able to create an account.
36:47
So I'll give a couple minutes. Anyone have any issues? Not just now, but for the rest of the day, please raise your hands. We have lab assistants standing. by to help you. Just wait a minute. Yes, so for aquarium, there should be something along the lines of, I don't know if you can a couple minutes to do the logistical stuff and we should be good to go for the rest of the day.
37:40
And in the meantime for the rest of you that are ready, I would also encourage you to log in to your LinkedIn. If you don't have LinkedIn, but if you have a resume file ready, please get that ready as well.
38:03
Resume file can be in any format PDF for the common flat files. But if you have LinkedIn, go to your LinkedIn profile, and there's a very easy way to download it into sort of a mini -CV form. So your profile and then save to PDF.
38:20
Again, the purpose of Lab 1 over the next 30 minutes will be you'll be using RAG or Retrieval Augmented Generation to upload your CV to our platform. And then you'll be able to chat to it both via web UI as well as using a Python notebook where we will demonstrate to you the API.
38:39
One common mistake. Some people are typing H20 .ai. That's very common. Just a full type H20 .ai. Yeah, that's a good point. Any time that we have you type H20, it's a letter O, not the number zero. Good call out.
38:55
Thanks. And since we're on my profile, maybe I'll just talk a bit about myself. I'm Chun Ming, Singaporean data scientist with H20. I've been with the company for two years, and we're quite proud of the fact that we are very big on Kaggle.
39:07
I'm a Kaggle completion grandmaster myself. And typically, I think for the audiences of these sessions, I think it's a mix of professional data scientists, professionals as well as students. I would strongly encourage you to try out Kaggle if you haven't.
39:22
It's a good way to keep up with data science. Okay, I'm going to switch to the actual lab. Last call out. Anyone with any issues, please raise your hand. So you should be seeing this. And the URL is genai -training .h20 .ai.
39:41
I don't see any hands up, so I think we're good to go. So I'll orientate you to this. This is actually when you land in our app store. But the ones that I'll have you focus on would be, if you look at the pane above, there will be two...
39:56
tabs, one called Enterprise H20 GPT. If you can see my mouse, can you open a tab of it? As well as my notebook lab. So you should have two new windows open from the top two pane. So again, open a new window for Enterprise H20 GPT.
40:15
Colloquially, we call it H20 GPT or H20 GPT Enterprise. And also open my notebook lab. And if you are able to do so, this should be the landing page for the H20 GPT. And this should be the landing page for my notebook lab.
40:33
And for the more technical folks among you, the notebook lab is basically a Jupyter lab environment. So again, a big picture, we will be using the web UI to work with your CV, as well as later on using Python notebook to access the same API.
40:48
So again, hands up at any point if you have any issues. If not, I am going to assume everyone's good to go. So let's go to the enterprise GPT landing page. So everyone should be seeing this. Everyone looks quite focused, so I assume everyone's okay.
41:07
So to orientate you, this is the web interface for our RAG platform, H2GPTE, where you'll be able to upload documents of different formats, chat with them. Okay. As always, raise your hands, don't be shy.
41:28
Okay. So on the left, right, you see this navigation pane, maybe I'll orientate you. So we have the concept of collections, documents, chats, jobs, and settings, collections of collections of documents.
41:42
You can think of it as a database where you can have a chat with a database of documents. Document would be where you can see individual documents in each collection. Chats are basically histories of chats with particular collections.
41:57
Jobs are just basically job monitoring thing where you can see what's going on. Last but not least, settings. I'll come back to this later where this is the web UI, but you do have the ability to interact with this using a Python client.
42:14
So there'll be later on in the notebook, you'll be needing this API key. So could I ask you to go to the settings page and create a new API key? You can just type any name for it. Once the key is generated, copy it and get it ready for, maybe put it in a notebook or something.
42:42
I'll come back to this again, but if you're ready, do so. So collections would be the focal point where you upload your documents. So could I ask you to go to collections and then you should see an icon called New Collection.
42:58
So again, collection is a concept for document of, sorry, collection, database of documents. So again, click on New Collection. And this is very important. Please be very careful about this. Name it My Profile with a camel casing NoSpace because we'll be using the API to access this collection later.
43:20
So My Profile, Big M, Big P. Again, any issues raise your hands please. So you create it. One thing you'll notice is that the name is not necessarily unique. Underlying, there's a unique ID that's created that you access by API.
43:41
So this is one thing to be aware of. So when you create a collection, it's empty. But you can go to the collection. And then there'll be another icon called Add Documents. So in terms of document ingestion for the web UI, there are three options.
43:57
Upload documents, import from file system, and import web pages. We're going to be using the first one today where you're directly uploading from your laptop. Import from file system, this is more from the location of the instance, so in most cases you won't touch it.
44:12
Third, import from web page, you can technically point it to a website, and there's some pre -processing code tied to a web crawler. Again, in your case, go to upload documents, browse, and point it to your CV.
44:28
Okay, that's a candidate, let me make sure I add mine. Let me just create a new one. Yeah, and by default I believe the LinkedIn generates file called profile .pdf. So you add it, and you'll be immediately transported to the jobs pane.
45:04
So maybe I'll give you some technical background. So what's happening under the hood is that you actually have seven components running. Only one of the seven components is the actual LLM, but conceptually when you upload a document, there's a bunch of stuff going on, chunking.
45:21
So when we send information to the LLMs, we don't necessarily send document by document. There's a concept of chunking where we chop up documents into fixed context windows. We do embedding to get it to tie into a vector database.
45:38
Embeddings just for more technical guys, we're using something called the BGE embedding model. And then we basically dump it all into a vector database, which is part of the RAG system. as a question from the lady at the back, maybe we could get one of the lab assistants to.
45:58
So has everyone managed to upload a document? So you can go back to the collection at the bottom, you should be able to see your CV. And one other technical point I'll mention about our platforms specifically is that in terms of ingestion, we offer a variety of formats.
46:19
So we support all the flat text documents, PDF, Office Suite documents. And the cool thing, which I find personally quite cool is that we also are able to ingest multimedia. So if you have audio files, you can actually upload audio files.
46:35
I won't demo it today, we are very focused on this LinkedIn example. But if you upload an audio document or audio file, it will actually be transcribed using a speech -to -text model. And the text will be sort of the basis for the collection.
46:51
So that's for audio files. We do also support image formats. I think Shira was demoing a bit of that earlier. But for images, we use deep learning vision. OCR is too light for it. So fairly sophisticated OCR model, where we are able to extract text from images.
47:11
So a big picture is that we support a variety of multimedia. But at the end of the day, the collection will be a collection of text documents. So is everyone able to get to this point, where you are able to see your CV?
47:26
Great. So really, the centerpiece of the UI you can see is start your first chat. And you can start asking questions at the bottom. So I'll just type in a sample question, like, who is this candidate?
47:38
Is it a candidate? And I encourage you. So this is meant to be interactive. You don't need to type exactly what I'm typing. But it's fairly intuitive. You should be able to ask questions about your CV.
47:51
And again, more technical background for the technical folks. The underlying LLM that we're using is Lama 270B. So it's a $70 billion model. I think someone raising their hands up. So once you ask a question, so obviously the answer will be here.
48:11
And from a UI standpoint, you see this thumbs up, thumbs down. That's a way for a user of our platform to be able to say that they had a good interaction or bad interaction with the answer provider. And it's stored in a database.
48:24
And via API, you are able to extract it so that users or developers working on our platform are able to improve the model. The other important thing is that there's also a show references sort of tab where if you click it open, it points to exactly where in the document the answer was derived from.
48:43
I think for your LinkedIn or your Facebook, or CV, it's only a couple pages, so this is less important, but imagine if you have an annual report of hundreds of pages, this is gonna be very useful. So the cool thing about RAG is that it also reduces the risk of hallucination, cause you're very heavily conditioning the questions that you ask on the information that you're sending to LLM, so I can ask something totally irrelevant, like I'm feeling a bit hungry, I haven't had breakfast yet, so I'll say, what is Luxa?
49:14
So normal LLMs, they will try to answer for you, but because, so what's happening under the hood is we are sending the closest context to it, as well as the question, so you can see that, it's saying that I'm not able to answer your question as it doesn't make sense, blah, blah, blah, right?
49:30
So this is a very good way, RAG is a very good way of sort of reducing the risk of hallucinations, and it's also tied to the concept of guardrails, so you might have heard of the, concept guardrails where LLMs by themselves are very powerful, but you can ask a lot of sort of negative questions and get negative answers.
49:49
So one key takeaway for you today is that if you use a RAC -based platform like H2O GPTE, you are able to reduce both the, I think there's a lady that needs help there, the risk of hallucinations as well as sort of a default guardrail.
50:06
So these are very basic questions, but you can also ask other questions like summarize my background or summarize the candidates' background. So again, I heavily encourage you guys to try asking your own questions.
50:33
Yeah, and you have access to this platform for all of today. So if you have time, feel free to go beyond using your CV, right? Try it, try it with other documents. This is a training environment. So one thing I should also mention is if you go to settings, right, you can see that this is version 0 .9 .2.
50:52
We are post version one for production. So this is just treat this as a development instance. Any issues so far? Please try to ask questions because we're quite interested to hear if you have any good or bad experiences of it.
51:14
Okay, I'll give you a couple of minutes just to play around with the platform. And then after that, we'll switch to the API. So you can actually scroll down. This is one UI, but if you scroll down. scroll down.
51:40
There's another chat. Oh, sorry. I think you, under that start, your first chat, it's a bit more nicer UI. Yeah. So maybe I can talk a bit more about the theory behind this while you guys are trying out stuff.
51:58
So this is Lama and talking a bit more as a data scientist. So Lama was trained on in terms of its training data was about 90% English, 10% all other languages. So what I've encountered, what we've encountered is that it handles English very well, handles English very well.
52:17
So one of the curious things if you look at research is that among all the various code switching, so English is something called code switching where you're mixing languages, Chinese, you know, Malay, Hokkien dialect.
52:33
A lot of the LLMs, including Lama, perform very well for English for some reason. I don't know if that's because it's seen a lot of English. But the other thing is that in terms of understanding, it actually does a decent job of understanding other languages like Chinese, Japanese.
52:49
Of course, the accuracy will depend on language to language. So you are able to feed in documents in other languages and potentially get good results. But what I would say is that in terms of responding in another language, because we are asking questions and right now it's responding in English, it still performs much better in terms of responding in English than responding in other languages.
53:12
So maybe one pointer, at least my personal pointer is that platforms like this using LLMs, open source LLMs might be good for ingesting documents in other languages, but I would recommend that you work with it in terms of English responses.
53:31
There are tips and tricks to get responses in other languages. Obviously the easiest would be to use a large language model trained in foreign languages specifically. Okay, I'll give another minute and then we're going to switch to the API for the folks that are ready.
53:46
Again, could I ask you to go to the settings page and create a new API key? You can type in any name here. And then copy the secret key somewhere to have it ready. For those of you that are super fast, like I was saying from the main landing page in the app store, could you open up the My Notebook Lab?
54:10
And you should be seeing something like this where on the left you should see a number of Jupyter Notebooks Lab 1 to Lab 5. Make sure that you're looking at Lab 1. Again, any issues, please raise your hand.
54:23
Happy to help you. And also make sure that so if you can see at the bottom, you should say idle meaning that the kernel is ready. If not, click there and you should be able to select a Python kernel.
54:39
This is basically... starting the Python instance if you haven't. So again, raise hands, any issues. So takeaway here is that what we will be doing is interacting with that collection that you uploaded using the web UI via API.
54:55
So there are three ways to interact with our platform, web UI, which I just showed you, Python. There's a Python client. And actually, you are able to also invoke it via JavaScript. I'm a Python guy.
55:08
Happy to answer questions about Python. JavaScript, I'll get my colleagues that are JS folks to answer if you have any questions. Okay, I see a bunch of very serious faces, so I assume that you've gotten here.
55:20
So... What we'll be doing is asking a set of questions through the API, as well as doing something called prompt engineering. You might have heard of that term. So the very first thing is that we offer a Python client available on PyP.
55:34
It's already pre -installed on this environment, but you can always pip install it. And something to be very clear about is that make sure that the Python client is the same version. But again, it's installed.
55:45
You don't need to do anything. So click on the play button up here when you're highlighting this cell to run it. So you should see maybe a quick s -trick, and then it changes to one. This shows that the kernel is running.
56:01
Maybe some of you aren't as familiar with Python. Do let us know. Raise your hand. If chances are, if nothing happens, your kernel hasn't been instantiated. So I assume that raise your hand, please, if you have any issues.
56:19
So I have some screenshots of the exact steps that we did earlier, but we will be jumping to step five, or rather step four. Before I jump to step five, I'll mention that. So you just uploaded your CV using the web UI, but here I saw just show, and you're not uploading your CV.
56:40
So again, we've created a client in step one called H2o GPTE, and then you do something called create collection, name it my profile, and then you open your file as a binary and then upload it. We won't do it because you've already done it via the web UI, but this is step five is where you actually be starting your hands on.
57:03
So step five is, and you can see under the authenticate heading, this is actually where you connect to the instance. Now this is my, so there's a rag URL and a rag key. Rag URL is already set, so you don't need to change it.
57:18
That's pointing to the training environment. Rag key is where you need to substitute my, my API key with your own API key. Again, your API key should be from the web UI earlier. So this is very important because if you just use the default setting, you'll be looking at my CV.
57:35
And I'm very happy for you to look at my CV, but I think you also want to look at your own CV. So substitute your own API key and then run this. Again, you can press the play button to run individual cells.
57:49
You should see an asterisk and then convert to a number to show that it's run successfully. If you are more into shortcuts, control enter is also a quick way to run a cell. So this is very important.
58:01
If you run it successfully and you are connected, there should be no error message. It should be blank. If you see any error messages, please raise your hand. Tim, someone there needs help. Again, I'll wait a minute or two to make sure everyone's there.
58:40
But for the folks that have successfully completed the last step, you can go to the next cell. This is also important where we use the API to list recent collections. This basically shows the collections that you've uploaded.
58:56
In my case, I've uploaded my profile multiple times. But it doesn't matter if you upload it once or twice. Under Establish Collection Name, it should be pooling one of the my profiles. Again, this is very important earlier that I really emphasized where you needed to name your collection my profile.
59:21
If you haven't done so, could I ask you to go back to the UI and upload? That's basically, we're doing text matching to find the collection that we're interested in. Again, you should see a long string of ID.
59:35
This is the unique identifier for the collection. Make sure that you are able to see this. If not, please raise your hands. I think people generally look okay. What we're doing now is we're going to ask a set of questions.
59:55
Again, this is meant to be interactive. I've started with a couple sample queries. But feel free to substitute. Maybe just run it first. So run the queries cell. And then the next chunk of code. I think if you're a Python guy, this should be quite easy to understand.
01:00:14
You sort of connect to the collection ID. So we are saying that we want to start a chat with the collection. And then we have a lot of questions. of something called session query, where we can ask questions and get answers.
01:00:26
So you can also play this. And you should be seeing responses to the three queries that we asked earlier. So again, I'll give you a couple of minutes. Once you've seen the default answers or answers to the default questions, try changing it up just to make sure that it's working as expected.
01:00:48
Yeah, while you guys are working on this, maybe I can just also add some color in terms of Genia, in terms of LLM. So I think currently in terms of LLM's open source, where we are seeing good results for commercial use, in terms of parameter size and complexity, it would be in the 70 billion models.
01:01:06
So you might have heard of the number of parameters in the LLM ranging from 7 billion onwards to 70 billion. I think 70 billion is very good. even using an off the shelf open source LLM in terms of performance for RAG in terms of most commercial users.
01:01:23
You might need to do some prompt engineering but in most cases I think it works very well. So it might be a bit slow because we have a lot of people hitting up the instances but you should be seeing Q1, A1, Q2, A2, et cetera.
01:01:36
Again, raise your hand if you have any issues. Please, please try your own questions because I wasn't very creative. All right, I mean just think. So maybe to take a step back, so it's not academic. So when we ask you to look at your CV, right, it's not just academic for fun.
01:02:00
There are a lot of immediate business use cases. For example, you can just using this, have it as a start point for a CV screener. Have it start as a starting point for some kind of HR, Gen AI board, right?
01:02:14
So don't think of it as a theoretical academic exercise. Even something as basic as uploading a CV or uploading multiple CVs, there are really interesting business use cases for this. For those of you that are super fast, we will go to the next step where we can do prompt engineering.
01:02:32
So by default, when you ask a question, there's actually additional sort of text sent to the LLM. By default, we send something called text along the lines, according to only the information and the document sources provided within the context above.
01:02:53
And then your question will be appended to that and sent to the LLM. But here we will be doing our first example of prompt engineering where we modify that. So what I've done is that under this prompt query, you can see at the end, I've added a few more words saying, and answering in fewer than 30 words.
01:03:09
So one other interesting pointer or tip is that LLM by default is quite wordy. So if you want it to be more... more concise, you can do some prompt engineering where you modify the prompt query. And this variable is sort of sent along with your question.
01:03:23
You can see under session query, there's a flag called prompt query where you can send it. And if you run this, the answer should be a lot faster than it was earlier. Okay, in my case, my Python kernel restarted.
01:03:42
But no worries, if you are running yours, do so. Any questions, does not have to be technical. If you have any theoretical questions, do feel free to ask me or the lab assistant during or after the session as well.
01:04:03
because there's a lot of magic happening under the hood. It's not just upload document, and then it gets sent to the LLMs. Again, there are seven components. There's a lot of pre -processing in terms of chunking, indexing, embedding.
01:04:15
And even when we send the matching context, this is a bit more technical to the LLMs. We do quite a fair bit of magic. So you should be seeing much shorter answers. Again, my kernel sort of died, but that's fine.
01:04:34
What I would also like you to do, since you're already looking at this, is modify the prompt query, right? Maybe, and I have it in cell 8, is you can also ask it to respond informally in Singlish. This goes back to my earlier point where, for some reason, Lama does very well with Singlish.
01:04:52
So again, by default, what we send to the LLMs by default is, according to only the information the document sources provided within the context above. But for these two examples, I've added additional instructions to the LLMs.
01:05:08
So the first one was an answering in few and 30 words. And the second example was an responding informally in Singlish. And in this case, you can see I've already run it once. Yeah, I was quite surprised by it.
01:05:24
And there's been actual research papers where they were looking at multi -lingual performance and they called out the interesting quirk where LLMs and Lama perform very well with Singlish. Right, so I think if you take a step back and think in terms of when you're working with Jenei, when you're working with LLMs, so I think the first instinct for all of our people, especially if you're a bit older and you work the deep learning techniques, is you wanna train your own model.
01:05:49
But in many cases for RAC, when you're working with documents, you might not need to train. What you can do is, again, what's called prompt engineering, where you modify the prompt and you can do it all over again.
01:06:03
of interesting things in terms of telling it to respond in a certain way. The last example I have is, so there are multiple prompts that are being sent to the LLM. There's a prompt query, but there's also something called a system prompt.
01:06:24
And by default, it's sort of an instruction to tell the LLM to behave with a certain persona. I think by default, we say, oh, you're a smart H2O .AI assistant. But by changing the system prompt, you can also have it behave with a different persona.
01:06:41
So this example on this cell, we are modifying both the system prompt, or rather only the system prompt, to say that your goal is to extract hashtags. The prompt query is switched back to the default, but the query itself, and this is quite interesting because we are not asking for a colloquial answer.
01:06:59
We are asking the LLM to respond in a programmatic way which can be used as inputs to another program. So here the query I'm asking is based on my LinkedIn CV, return only three hashtags about the person this profile belongs to as a JSON file, which is a data structure, with a single field called hashtags.
01:07:20
Do not use the person's name as a hashtag. So again, you don't need to train LLMs in many cases, do stuff like returning programs, but you can by doing prompt engineering or structuring your queries an interesting way, you can get interesting results.
01:07:37
And if you run this, if you look at the answer, it's basically a JSON string. Right, so for the more technical folks, you can use LLMs and chain it where outputs from LLMs could potentially be inputs to programs.
01:07:53
So this is all I had, but I really want to, this is really the starting point. The second lab also depends on the fact that you've uploaded your LinkedIn CV and you've created my profile collection. But for the rest of the day, if you have time, do feel free to use both the UI and the Python API just to upload your own documents and ask questions.
01:08:20
And if you have any thoughts, questions, or even if you feel like it's not performing in an expected way, I think you can ask us and we can give you some suggestions on how you can improve performance.
01:08:29
So I will stop here in the interest of time, but... Yeah, so that's a good question. The name, the string name is not a unique identifier. Underlying, there's an ID that's created and stored, which you can extract using the API.
01:08:52
So good question. You can use the same name. Okay, I think everyone's good to go. I see some smiles, so hopefully that meant the session was at least interesting. I'm going to hand over to Jen, who will be taking the second lab.
01:09:09
Jen, please. Okay, I think many of you, like me, are having issues with the Python kernel. Again, this is a training environment, so I hope you understand that. So if you see this Python initializing, we are doing some stuff on our back end to try to get you going, but be patient with that.
01:09:36
Thanks. Hey, cool guys. We're going to kick off Lab 2 now. I'll quickly introduce myself. So my name's Jan. I'm a customer data scientist from H2O. I'm currently based in South Korea and I'm originally from Australia, so I'm really excited to be in Singapore with you guys today.
01:09:53
Today for Lab 2, our focus is going to be on we have this rag, we have the back end, but how can we kind of make this consumable for users in the front end? So we're going to use the same My Profile Collection that we did for our Lab 1.
01:10:08
So hopefully everyone was able to at least create the LinkedIn Profile Collection. Now I want to call out two things before we get started. First of all, please, if you haven't already generated, please generate your H2O GPTE API key.
01:10:23
If you don't know what that is or you don't know how to do that, please put your hand up and someone will come around and help you find it and make sure you're ready for the when we do the hands -on coding component.
01:10:35
Second thing, sorry, I'll zoom in. Second thing is when you created your collection, it's currently set so that the collection needs to be named something that contains My Profile in it. So if your collection is labeled, actually labeled something different, it's not an issue.
01:10:55
If we go into H2O GPTE and we click the collection that we loaded our LinkedIn Profile to, so mine's called My Profile, but we can actually edit that name live now. So if I go edit details, I can update this and label it correctly.
01:11:10
And that's just going to help to ensure that when you do the hands -on component to build the app, it's all ready to go and things will flow as smoothly as possible. So just give one minute and then we'll go.
01:11:32
Okay. Now we're actually not going to be using the notebook. for this lab, we're going to be using one of our open source applications that help us build our wave applications side by side. I'll explain what wave is in a second but just so we're all launching at the same time, in the lab two there'll be a link where it will take you to a screen that demonstrates and will have the name Wave Studio at the top.
01:11:59
So wait a second, so it should look like this. Has everyone been able to get that screen up? Yeah? Silence is golden, we'll move on from that. All right, the next step we're going to do just to ensure that you don't accidentally use someone else's Wave Studio instance, we're going to click on the down arrow next to visit and we're going to click run in private.
01:12:22
And like I said, the reason we're doing that is just to ensure that when you're coding and doing the live code updates, someone else isn't accidentally in your instance and making changes which might cause some issues as well.
01:12:32
So just a reminder, we click the downward arrow next to visit and then we're going to go to run in private and wait for this to launch and get started. Cool. I'll give you two minutes. I see we have 58 people creating instances at the moment which is good, so I'll wait for a few of those to get started.
01:12:58
Yeah. Cool. What you need to do is when you come into the Wave Studio link, there'll be a visit button here and next to the visit button there's a downward arrow similar to this one. If you click that, there'll be run and then run in private.
01:13:16
Select run in private and what that's doing is it's launching your own individual Wave Studio instance so that it's only your own code and we can customize it just for yourself. Cool. All right. When it's loading, what you should get is it might automatically pop up if it's allowed, if it hasn't and you have a, it should say, visit.
01:14:01
If you have a visit button appearing next to your instance, click visit and you should be taken to a screen that looks like this. All right. Are we following so far? Yeah. Awesome. I'll just wait one minute and then I'll move forward.
01:14:18
But if you want to, you can press the start coding button and what's going to happen is happen is it's going to load a screen where we have our code side by side with what the AI application that we're actually going to be building or at least updating today in this tutorial.
01:14:51
Cool. So when everyone's got this screen, we'll keep moving. What I'll do while we're waiting for that is I'll just show you what we're planning to build today is a UI or a front -end for our LinkedIn profile or collection that we created together.
01:15:07
Now what we're going to do is we're going to actually apply some personalization to this so that it's based on your profile and things update automatically. For those that have their environment ready, The first thing we need to do is actually pass in our API key from H2O GPTE.
01:15:26
And this is at line 17 here. So line 17, yeah. Yeah? We're just going to replace this. Yeah. Oops, Daisy. If you do what I accidentally did, you just need to make sure to append studio to the URL and that will take you back to the side by side screen as well.
01:15:53
Okay. Has everyone been able to update the API key so far? All right. I'm going to chat through Wave for a little bit while we get started and show you how to update some UI components pretty easily.
01:16:08
But if we take a look at this code, we can see here, H2O Wave is an open source Python SDK that makes building AI applications really, really easy. It ultimately is built up with the skeleton code that we have here.
01:16:27
So we have our initial code that actually sets up our Wave environment with the Wave server in the backend automatically running. If we scroll down here, these next components, so from line 43 down to 66, that's just setting up the initial UI themes and sizing of our different, three different sections of our AI application.
01:16:47
Now if you want to, we can actually live update this to whatever theme we personally appeal to. So I think I'm going to choose neon. So I can copy and paste that into this theme location here. And we should see this application live updating on the right -hand side.
01:17:05
So where I updated was line 47. Could be 46, 46, 47. And you can update it. Below that, on line 48, we have a range of different options that you can choose from for your personal application. Okay, cool.
01:17:24
All right, the next component we have from line 68 to 77 is actually this header card. So what I'll do, don't do this, but what I'll do is if I comment this out, you'll notice that my header card is completely disappeared.
01:17:37
So these lines here are really all about, whoops, are really all controlling this personal header card that we have here. The one thing to draw attention to is actually this bui .personacard and this we're going to have live update based on our LinkedIn profile in a second.
01:17:59
Finally, we have a photo card at the bottom that's automatically generated. You can update this later if you want to miss a made with whatever makes you happy. All right, the next component we have is once we launch this app, it calls this home function.
01:18:13
Now this home function actually just sets up this home page that we're looking at. It has two components. The image here is static. If you click on it, do anything to it, nothing happens. You could update this image to whatever you wanted to for whatever AI application you're looking to build.
01:18:26
The next component we have here is this launch app button. And what will happen when we click this launch app button is actually it's going to set up and start to launch our H2O GPTE or our RAG connection to the backend here.
01:18:42
Now, if you click launch, what you should see is this top profile button should actually update with your name and your current job title. Now, how we've done that is... Let me just see if I can make this small.
01:18:56
Okay. What this code should look familiar to you based on Lab 1. So we've just connected to the GPTE client and then we found the collection that we're interested in. Now, if people aren't seeing a chat box appear or seeing their name change in the profile, there are two things that could be wrong.
01:19:12
One, you haven't updated your API key. Or two, your collection isn't called my profile. So please feel free to go check that if you aren't seeing this chat box appear in the bottom part of the screen as well.
01:19:26
All right. Keep going down. If we take a look at this, and what I've done so that it just runs a little bit faster is we have this H2O GPTE call here. Now, what we've asked it to do is actually provide one JSON, which has two string fields, name and title, and we've asked it to automatically extract that from our LinkedIn profile for us and we can then integrate that into our AI applications, which goes to show that we can get outputs from Generative AI and utilize them as direct inputs into any application through integrations.
01:19:59
As long as you specify the correct format, you should be able to get that repeatability as well. Now, we're gonna scroll down and we're actually gonna add one more component to our profile tab at the top.
01:20:12
Now, I know we ran out of time before, but what I've done, what we're gonna do is actually change this H2O GPTE call. We're going to update it so it extracts three hashtags that are personalized based on our LinkedIn profile.
01:20:24
Now, I've got two examples here. You can use them yourself or if you want to have a stab at writing them and seeing if they work, you can do that as well. But all I'm going to do is just uncomment here.
01:20:36
So I have system prompt which controls the system -wide behavior of the So I'm going to update this here so that my system prompts hashtag is being passed in with my query. And then I'm also just going to pass in my query as well.
01:20:56
All right. Then all I do is I pass those two components into the same Python client that we used before. And it's going to extract those hashtags for me as soon as I click that launch button. So let's go and click that again and we should see those hashtags appear in the top right hand corner.
01:21:18
as well. Awesome. Okay. Did that work for everyone? Is it working? Everything going okay? Any questions at all? Please raise your hand and someone can come around. No? Okay. Cool. Now the last component that we have and that we're going to touch on is this chatbot functionality which allows us to chat just like we did in our H2O GPTE allows us to chat with our LinkedIn profile.
01:21:49
Now when you're creating chatbots like this, you might want to apply some control. You might want to apply the style that the chatbot responds in and things like that. So we can do that in this app, sorry, in the code here so that when someone else is coming to our application, all of those things are set for the application wide.
01:22:10
Now the chatbot functionality is really controlled in this chat answer function. So if we look at LAN215, you'll see here that we have these two boxes. We have a system prompt that we're just going to leave as default but you can update that for your application or whatever you want to do to apply some control.
01:22:29
So our system prompt says it's an AI bot with access to your LinkedIn profile asking how it can assist today. Whether it's updating your professional summary, connecting with new contacts, job advice or anything related to your career, they're here to help.
01:22:43
So just let me know what you need and I'll provide personalized assistance based on your LinkedIn information. So we've set some contacts for the ALLAM when it's generating those responses, the types of queries and responses it should be giving to the user in this chatbot section here.
01:22:58
The other thing we can do is just append a personality prompt. So when we ask a question here now, say, put this down for a sec. Okay, I'm just going to quickly go back and touch on the system prompt in a second but while you guys have launched the app, you should see it thinking and generating that response for you in the chat over here on the left hand side.
01:23:45
Now, I'm just going to go back and chat through the system prompt and personality prompt again, just so we're all on the same page and we have a good understanding of what's going on. Now, I've specified my system prompt here at line 217, and this would generally be changed based on the type of AI application that you're building.
01:24:02
So for example, if you wanted to build an AI application that provides HR advice based on your HR policies, this system prompt would be customized based on that application, because you want to ensure that the responses it's providing is one, restricted to the types of questions you want it to be answered, so we can start to apply some guardrail type suggestions here, telling it not to answer questions, telling it types of things it doesn't want it to talk about.
01:24:28
We can apply these restrictions in the system prompt. Then we have the personality prompt, and this is really all about controlling the style of the responses as well. Currently, I've instructed it to always respond with formal language, but I can change that, and we will in a second, but I do want you to generate your first question response.
01:24:45
So I don't have a master's, so I've asked it if I should do a master's and if it would recommend, and it's generated a very professional response here for me about not only which master's programs, but different options I could look at based on my LinkedIn profile.
01:24:57
Now, what we do is using both system prompt and personality prompt. We're going to pass that in, so every time someone asks a question, this system prompt and personality prompt is actually pre -appended.
01:25:09
So that with our question, so when the question is sent to the H3GPTE, it's sending not just your question, but also the guidance around how it can respond. And that's how we can start to, I guess, gain some control over how our chatbot responds from an organizational perspective or an AI application perspective.
01:25:30
I've just passed that in here at line 233, where I have the session query. We asked the question, I've also put in a timeout here just so we have quick, so that these responses come back quickly, and if it doesn't, it'll error quicker.
01:25:45
so you can try again. And then I've passed that in. Now, what I'm going to do is actually going to change this. I'm going to do a little fun one, but obviously, this is professional and can be used to control professional tone and things like that.
01:26:00
But just this one, sorry, don't need that. This one, really, you can see a really big difference into the response. So I'm going to update my personality prompt now to say, always respond in the form of a wrap.
01:26:12
So if I follow that same process, if I launch my application, it's just taking a second. The reason is loading so long is every time I click this launch app button, it's connecting to my H2O GPTE client that we're all connecting to at the moment.
01:27:09
And then as well, I've made it so that it's generating those three hashtags live on launch as well. So it's doing the connection and that query before it sets up the UI. And then obviously we have quite a few people hitting H2O GPTE at the moment, so it might just take a little bit of time.
01:27:24
What I might do just for speed, and if you want to as well, I might just comment out. All right. I'll touch on this and then I'll just touch on a few closing notes before we go out. You'll see here that now my system and the personality has changed.
01:27:41
It's written me a wrap about how I should definitely consider doing a masters and all of the different things I can possibly do. So the idea behind it is, although this is a fun example, the idea is that when you are implementing these AI applications, you do have this control and these restraints that you can apply when you are setting up these internal chatbots and things within your organization.
01:28:03
I just want to touch on, I've put these notes in the lab, so if you want to come back later and do the Wave Studio again, that's totally fine. And you can follow these to change everything as well. The cool thing about AI Wave is that it's really, really easy to deploy these applications as well.
01:28:20
All you need to do is specify your app code that we've done. We provide an app .toml file, which if you go into example notebooks in the lab, app template, I placed it an example in here as well. So the app Tom all just contains some information as well as your requirements .text file, which just contains the requirements for this AI application, relatively low level here.
01:28:42
And then we can just bundle those together either as a wave bundle or a zip and we can easily deploy those into our AI platform as well. You'll see here I have some apps deployed, actually I have a few versions and they're all ready to go and you can set the permissions for other people to then use that application through AI Cloud.
01:29:01
You can also use AWS and other platforms as well, but this is just one example of when you've built your application. Can I make a reference in future? Can you possibly make a sample PDF file for our app?
01:29:15
A sample PDF? Yeah, sample PDF, but you'll see our PDF and you'll find a CD or whatever. Yeah, no, that's great feedback. It's good for people to do a playlist or a template. Yeah, yeah, no. Awesome, thanks.
01:29:29
Cool. Like I mentioned before, the system prompts and things like that is one avenue for guard rails, but there's other guard rails you could implement when building these AI applications. You can have them as part of that system prompts, but also you can have prechecks and postchecks for what the GPT is outputting, as well as the types of questions going in and have an automated response generated for those types of systems as well.
01:29:51
Awesome. Okay. There, we do have a public AI app store that has public examples of different AI applications that have been built, and everyone has access to this, so you can take a look. We also have the cool thing about this public AI app store is actually the GitHub code is fully open source as well.
01:30:09
You can also use these other AI applications as a template to build out your own gen AI applications as well, and these links are all available in that lab there ready for you to go. All right. I'm going to stop here and pass over to Vishal to go through fine tuning as well.
01:30:28
Yes, of course. Yeah. Hello, everyone. So my name is Vishal. I'm a senior data scientist working at H2O. Yeah. So I am a data scientist based in Singapore, and we are going to now look at last language models fine tuning.
01:31:04
But before that, so we'll be using two different hands -on activities. First we'll be doing a Python based fine tuning of language model, a simple small language model. So make sure that you are back to these notebook labs, and you are clicking on lab three, which is around fine tuning.
01:31:25
Thank you. make sure you are able to access that. And in the last lab around RAG, you lost, most of us lost the kernels. So make sure that you again, see if you are able to launch the kernel again. Just click on Python and select it here.
01:31:54
And also for this lab, we'll need a aquarium. So I hope everyone has created an account at Aquarium. And if you haven't, you just need to go to aquarium .h2o .ai. Then you need to just log in here. Make sure you click you are not a robot and click on login.
01:32:17
Then after that, you need to click on browse labs. You click on browse labs. Yeah. And after that, make sure you go to lab six, which is LLM studio. And click on this one. Okay. I'll go back to that.
01:32:45
And then after this, click on start lab. So once you click on start lab, you should be able to see a URL here. So it is launching a AWS instance, uh, containing the LLM studio. So you click on this and you should be able to see a screen for LLM studio, which is a no code UI platform for last language model fine tuning.
01:33:13
Okay. So we'll be using LLM studio, uh, but we'll be also using a Python code, uh, for LLM studio. And so make sure that you are able to know this is wrong. Make sure you are able to launch a kernel.
01:33:40
So in the notebook at the top right, uh, click on the kernel here and select Python and select here. Okay. Then, uh, after a while, you should be able to fetch the kernel. So I'll just give you a very quick, uh, intro to fine tuning.
01:34:07
Uh, I think, uh, many of you are data scientist and you may be working with a natural language processing models, uh, especially Bert, uh, Bert models over the last five years. So fine tuning, uh, what do you mean by fine tuning off a large language models, all fine tuning off language models?
01:34:23
Since the act of taking a pre -trained foundation model, uh, so now foundation models are generally these GPT models. But before the advent of chat GPTs and all, the most popular language models were the BERT models, et cetera.
01:34:37
So you are just taking a pre -trained model, and you are just further training it on a new data for a specific task. So for example, and this task can be a task like text summarization, text classification.
01:34:52
So earlier for NLP tasks, you may be fine tuning these models for text classification kind of tasks. But now we have this chat model such as H2O GPT models, which are already instruction tuned, so they are able to answer your questions.
01:35:05
But many times you want to fine tune them further on some of the specific tasks. For example, you want to fine tune this model and create your own GPT to change its behavior. For example, you want the output response of this GPT model to always be in bullet points, or you want the output response to be always as a Python code.
01:35:26
So code Lama is one example where it has been fine tuned on code data and it's always generated a code output for you. Or you may want to assume some personality. So you want to, the UGPD model to always respond as a financial analyst.
01:35:41
If you're in healthcare sector, then you want it to respond as a doctor. Or you may want to assume a famous personality such as a leek one you or someone else as well. Or you may want to personalize even further.
01:35:55
You may want to create your own GPT assistant who learns from your own data and able to respond according to your personality. So we will be doing two labs here. One using Python notebook where we will be using like a relatively old language model called BERT and we will be fine tuning it on textual entailment task where the objective is that you are given two sentences and you need to figure out whether this sentence two is entailed in sentence one.
01:36:27
So that will be first task and second task would be where we will be actually fine tuning instruction tuned model, last language model and we will again train it on, train it to respond as a LinkedIn influencer.
01:36:41
So we'll tune it on a data where you have, you'll ask it to generate a LinkedIn post as a famous LinkedIn influencer and its response should be the LinkedIn post in the format of that particular LinkedIn influencer.
01:36:55
So we'll look at these two tasks. So first let's go to a notebook where we'll fine tune. Yep, so where we'll fine tune a BERT model using WNLI data set. So for that we have to. Go back to genai -training .h2 .ai, go back to your notebooks, my notebook lab.
01:37:57
Yeah, and then you go to lab 3. So most of the codes are already preloaded here. So what we are doing here actually, we are using the Hugging Face Transformer library and we are using a BERT model, BERT based model, which is around 100 million parameter model.
01:38:20
relatively small compared to the GPT models that we work nowadays. And we will find WNLI dataset, which is a natural language inference dataset here. So go ahead. So if you have received, you have got the kernel, acquired the kernel here.
01:38:38
Yeah, I have. So you just need to click on these, just need to run these codes. Seems this is running a bit slow. Okay, so what if you scroll down here? So when you are actually working with this language models, first thing you need to do is to tokenize the dataset that you are working with.
01:39:15
So after you, the dataset is downloaded, which is the glue benchmark data of WNLI dataset. After that, you have to also load a tokenizer. So you have to load the tokenizer using these commands. And then after you have tokenized the dataset, then you need to load the pre -trained model weights.
01:39:40
So in this case, the pre -trained model weights will be from the bird based model. And after that, you have created a trainer configuration where you are defining which model you are using, which dataset you are using.
01:39:54
And this dataset will be the tokenized dataset. And after that, you will train the model. And this training is actually fine tuning the model. So bird based model is a encoder based model, which has been trained on like mass language modeling.
01:40:07
So it's not a generative model. It's just an encoder model, which is actually able to understand your data, your English data very well. But you need to fine tune it to be able to respond according to your training.
01:40:20
task. In this case, our task is actually natural language inference, which is around textual entailment task where objective is that the sentence two, if you are giving two sentences, then sentence two is actually entailed in sentence one or not.
01:40:34
So it looks like this notebook, this kernel may take some time for us to work. So you can go ahead and run these all these cells in the notebook later on. But for now, maybe what we'll do is we'll use, we'll fine tune a large language model using this tool called LLM studio.
01:41:00
This is an open source tool that H2O launched, which you can use for your fine tuning task. So if you are working with some large language models, you can actually use this for doing fine tuning of those language models in a no code manner.
01:41:15
So for that, again, you go to browse labs. Click on lab six lm studio and click on the URL And you should be able to see you should be able to see the screen like this which is s2 lm studio So I'll just wait for two minutes here to make sure everyone is here Is anyone having issues acquiring the lab Change other Mary .
01:42:22
If anyone is not able to instance of LLM studio, please So LLM studio is a platform which you can use for fine tuning models. So in the landing page, you can see how much resources that you can use to make a large amount of Then second step is around data sets.
01:42:54
So here what we will do is we will import a data set which is our LinkedIn influencer data set. So here instead of uploading from our laptop, what we will do is we will bring the data set from S3 bucket.
01:43:07
So just drop down here and click on AWS S3. Then you need to specify the bucket name. that you have in front of you, there is bucket name there, but I'll also acquire... In the lab three also you will see on the top the link to the URI of the bucket name.
01:43:31
So this is the bucket name from which we'll acquire the data. So make sure you copy it from the lab three or you can also see the URI from the sheets that you have in front of you or in your emails. So what I'll do is I just click on the URI of bucket name and then you don't...
01:43:56
It's a public bucket so you don't need access key and a secret key and then you drop down for the file names and let's select this one influencer data cleaned sample. So I have created a small subset of the data set because we have a limited time and so we are going to be using a small data set here.
01:44:20
Yeah. So once you have selected the file name, then click on continue. Make sure you are using this file. Yeah. Influencer data clean sample. So it's a small, very small subset of data set on which we can, we are able to run experiment in this limited time because last language modeling fine tunings, a task can take days on number of GPUs.
01:44:45
So, but here we have just minutes only. So we'll be fine tuning a small model on a small data set here. Okay. So I hope everyone is, uh, has is acquiring this data set. Then click on continue. So once yeah, after that, you need to configure the dataset.
01:45:08
You need to specify the train data frame. If you have validation data set, you can upload that, select that as well. But in this case, we do not have will do the validation from within the train data set.
01:45:19
Then you need to specify the prompt column and the answer column. So because we are fine tuning this language model on the responses that you want to generate a get from this language model based on our prompts.
01:45:32
So the input to the language model with the prompt and the output will be the expected response that we want to get. So our input is actually the instruction that we give to the model and the answer column would be the content because we want this language model to generate the content.
01:45:49
So make sure your prompt column is instruction and answer column is content. Uh, you, so this parent ID column is optional. So if you have a chat data set where you have interconnected prompt response, uh, data set, then in that case, uh, you can specify the parent ID so that, uh, the model understand that it's a continuation of conversation over a chat.
01:46:13
Okay. So prompt column is instruction, answer column is content. And after that, click on continue. So once you click continue, you will see that it has uploaded a reset where the prompt is the query that we are giving to the model that write a LinkedIn post in the style of an influencer.
01:46:37
And the answer is that particular LinkedIn post. And then click on continue here. So then our process of acquiring the status is ingesting this, this data set is collect is complete. Then click on this data set here.
01:46:58
And what you will see is some of the some of the EDA on this data set. So you can visualize the data set, how it looks like. And you can also look at the train data statistics. So here you can see what is the what is the length of the prompt and what is the length of the response.
01:47:19
So this is an important statistics to know because you will use this for defining the context length and the response length during the fine -tuning stage of language model. So here we can see that the text distribution, I mean the largest length of the prompt is around 450 and the largest length of the response is around 280 or something here.
01:47:47
So after you have done this initial EDA on dataset, then you go ahead and click on create experiment. You go down here and click create experiment. So this will bring you to the experiment page of LM Studio and where we have uploaded this dataset here, influencer data clean sample.
01:48:10
Is everyone able to reach this experiment page? I'll just give a pause here. If anyone is not able to do it, let us know. Make sure you're using this dataset, otherwise the experiment is going to run for a much longer than what we expect.
01:48:35
Okay. So LM Studio actually makes your task of LM fine -tuning much easier because it's a relatively complex task and there are many, many hyper parameters which are involved here. There are many settings around like the experiment setting, dataset settings, around the tokenizer settings, around model settings, et cetera, that you need to change.
01:48:58
So LM Studio make this task of acquiring the settings, changing the settings much faster. So to be able to fine -tune your model on a certain task, you have to experiment with different settings. So it is the size of the data, the size of the model, the quantization that you apply for fine -tuning, et cetera.
01:49:18
Or you want to apply it to the model, apply a Lora or not these kind of techniques or not. So you need to experiment with these settings to be able to get the best performing last language model on your task.
01:49:27
Yeah. In this general setting, there have been so many options. Yeah. We'll go through. It takes time to find things for this. Yes. Is there any sun that works for that to automatically adjust by itself?
01:49:42
Yeah. So this tool has been built by Kaggle Grandmasters, who are actually experts in last language models and finding. So there are some of the settings are already pre -loaded here. And yeah. And maybe Chowmein can help me.
01:49:57
No, because I helped work on this platform as well. So your answer, this is the open source platform. For most cases, the default settings are good. Maybe you might just want to change the model that you're training.
01:50:10
We do have a proprietary enterprise platform that's similar to this web. You do exactly what you're asking, which is. We have a drop down which basically sets the one basic settings all the way to expert settings.
01:50:25
So open source, we throw the kitchen sink at you. But for most cases, the default settings are good enough. Thank you, Chowmein. So these default settings are also capably crafted by KGM Slack, Chowmein, and it should be able to work quite well for your tasks.
01:50:42
So here, so there are different type of problem types. So one is causal language modeling, which is our task. But you may also want to be doing reinforcement learning using human feedback. So if you're familiar with that term, that kind of task also you can be applied here.
01:50:57
You may be doing sequence to sequence modeling where you use encoder, decoder architecture, or you may be using this GPT model for a classification task. So that also you can do where it actually gives a state of the art performance.
01:51:08
So there are different type of problem types that you can do. But in this case, select causal language modeling. Then in the experiment name, I will suggest you to give your own experiment name. Maybe call it like my GPT because actually you are creating your own GPT model here.
01:51:27
And in backbone, if we train, you can see that the default model is Lama 2, which will actually give you good results, even though it's 7 billion. But we want to quickly finish the experiment. So let's select a very small model, which is a 125 million parameter model, which is Facebook, OPT, 125 million.
01:51:50
So this model is very, it's like a toy model. It's a thousand times smaller than the state of the art right now, which is Lama 70B. Yeah, but we will be using this for having a quick experimentation using LM Studio.
01:52:06
Then there are some other settings as well, which are the data set settings. You can create your own personalization as well. You can give this chatbot your personalization. your own names such as my GPT or my LinkedIn GPT, something like that.
01:52:22
Then you can specify the validation size, but let's leave it as it is. The prompt column is pre -specified. Let's leave it as it is. One thing you may want to change here is the maximum length of the prompt and maximum length of the answer.
01:52:39
So because we earlier we saw that the maximum length of the prompt in our dataset was around 450. So we want to increase it a bit here. Otherwise, it's going to cut the input out. And the response also, we want to increase a bit here.
01:52:58
And the maximum length is actually the maximum length of prompt plus the answer because we are doing autoregressive modeling and LM will use the combination of prompt and answer to do this. Fine to name.
01:53:10
So we increase it further as well. Maybe make it around 1000. Then you have some settings around quantization. So you have int4, int8, et cetera. But for this experiment, let's keep using int4 so that it runs faster, loss functions, optimizers.
01:53:32
In the epoch settings, you can run it for more number of epochs, but let's keep it at one epoch only. Some settings around Lora, if you are familiar with fine tuning, then you may know about this technique called Lora, which allows us to use very large language models in a limited GPU memory as well.
01:53:53
So here you can define the effectorization, the dimension of the effectorization matrix, which is the R dimension here. You can reduce it to three or just leave it as four. Right, so similarly, there are many metrics here.
01:54:11
One, another metric here is that for evaluation of these models, you want to use metrics such as blue or perplexity or roge kind of metrics, or you may want to use a GPT model, another AI model to evaluate this performance of this fine tuning.
01:54:27
So you can select another GPT model as well. So for example, you can use GPT 3 .5 or GPT4 models too, also evaluate the performance. Right, I think so. We have played around with some settings. You can do it.
01:54:43
So this platform is open source. You can also install it in your system, and you can use it for fine tuning and play around with these settings as well. But for now, let's click on an experiment. So this will take a bit of time here.
01:55:02
Yeah. Yeah. Okay, so this will take just a few minutes here. But after this model is, after the experiment is completed, then we can able, we'll be able to push it to hugging phase. As a checkpoint, you can download the particular model weights also for your own deployment.
01:55:22
One thing I want to highlight is that after you have finished the experiment, then one thing you need to care about is the last language model ops, which is the ML ops of last language models. So this also deals with the deployment and operations of last language models.
01:55:44
So it's similar to a typical machine learning model deployment and operations, but some other things that you need to care about is the customization, the optimization of inference. So for example, in customization, do you want to apply some prompt templates, some quantization, etc.
01:56:00
during the inference? For optimization inference, we want to use some techniques for faster inference such as VLLM or TGI techniques. Then you want to apply some resource controls for inferencing and scoring.
01:56:14
Then you also want to take care of some complaints and regulations where you want to put some guardrails when you are doing the deployment using this large -naked models. So all this is actually handled in H2ML ops here, but these are the different aspects of LLM ops that you need to be careful about here.
01:56:33
So let's go and look back to our experiment here. Let's see if it's finished. Okay. For me, it is around 99%. How about others? Is anyone able to finish the experiment? Yeah. Okay. So one gentleman is able to finish it here.
01:56:54
Okay. So for me, also the experiment is finished. So after the experiment is done, you click on the experiment, you can see how the quality of training was. But one thing you want to do here, one thing you want to do here is to push the model to hugging face so that it's ready for consumption or you can download the model weights for your local consumption as well.
01:57:20
So what I'll do is I'll push this model to hugging face where I can give my hugging face account and hugging face API key, which was an optional step during this training. So using this, use your hugging face username and API key, you can export these models to hugging face.
01:57:51
So once you do that, you'll be able to see in your own hugging face account, you'll be able to see the models that you pushed earlier. So around four days back, I pushed this model around LinkedIn posting and yeah, now it's available for me to consume for my own LLM ops.
01:58:06
So yeah, thanks for sticking with me during this fine tuning steps. Now I'll pass to my colleague. Timothy for the next step where you want to prepare data for fine tuning and how do we do that? Hungry Everyone is hungry Let's finish this fast.
01:58:39
My session will be cutting short a bit So we have some notebook, but we will not go through today Later on if you have time just like there's left four and left five in the notebook feel free to run It's just more explaining some of the data preparation tasks for LLM Some of you might actually coming from tabular data preparation background You might doing a lot of joining missing value handling, but for the LLM specific sometimes you would test We will do something like profanity check and test like readability test so those kind of Details will be shown in the notebook But today we don't have time to go through that.
01:59:14
Feel free to explore later on. So for us, in the last session, what we're going to do is to try out the product, which is the Data Studio, developed by our Kaggle Grandmaster here, Shivam, Jen, Nishan, and Tariq.
01:59:31
So here, just feel free to go back into the aquarium platform, click on the Lab9, which is this Lab Data Studio 0 .4. Everything is pre -warm. Just follow what we do, simply by clicking Start Lab. Immediately, you should see that there's a URL already.
01:59:50
Click that. Then what we are going to do is to, just now, what Vishal was showing is about how to fine -tune the model. Imagine that, for example, if you have some video, or you want to do some, like, CNA newspaper trying to fine -tune your model, answer like a CNA, answer like a tech guy.
02:00:19
How do we prepare those data from video perspective to newspaper perspective to prepare those data into a Q &A pair? What we are trying to do is exactly doing this. Feel free to later on explore the features one by one.
02:00:31
But today, mainly, what we're going to go through is to this tree step. Click on the step one, which is the Q -rate. You can see that we are supporting multiple formatting. Just now, what we have been doing so far is using your LinkedIn poll file, so PDF straightforward.
02:00:48
If you have any kind of PowerPoint, MP3 file, or dog file, feel free to just import. How to do that? Go into the right -hand side. Click on the New button. And now, we are basically opening something called a project.
02:01:04
Here, you can maybe leave the project name as random, but simply just pay attention on the task type on the right -hand side. So we can generate Q and A pair for fine -tuning the model, but we also can generate other types of content, for example, summarization for your sequence -to -sequence model fine -tuning as well.
02:01:23
In this case, we can stick to question and answer and then click on browse. Upload your document here. For example, you have a profile linking document and click open. Everything okay. Click upload. The document is quite fast, but again, if you have like a hundred or thousand of document, we do have something called smart chunking.
02:01:47
Basically, we'll do some K -Means analysis, not chunking everything. In this case, simple, just click run pipeline at the bottom. In this case, very similar to the REC demo that's shown case by Chen Ming.
02:02:01
So we are doing the test chunking first, and now we are processing the file. If you see that there's a 100% complete, simply by clicking refresh. You will be able to now starting to generate the Q &A pair one by one.
02:02:16
While we are waiting for that, see if you feel free to raise up your hand if you have any question. I also generate, for example, from let's say the TED video. So they would basically generate 22 question.
02:02:30
This video is about what is decision fatike. Basically, you make a lot of decision a lot. Now you feel a bit numb. How do we actually trying to handle these processes? Similarly, the Q &A paper is also you can feel free to pick up some of them and upload updating that as well.
02:02:49
Anyone have finished this part? OK, has been complete. So I just need to wait for this blue box go away and then click cross button. And now you should be able to see something like this. This is your linking profile.
02:03:09
Upload that and generate the question accordingly. If no question, then what we're going to do is to very straightforward to show you the last part is to sometimes if you have some document that is the Q &A pair that is irrelevant, for example, native language, maybe it is not related to my fine tuning model, we can click on that and then market us.
02:03:35
It will be relevant. Maybe you go through some of them. You want to modify some of it. For example, I click on this guy. I want to modify some of the content. You can also modify the question and the answer straightforward.
02:03:46
Once it done, we can click on the right hand side. This publish us preparation project. I know I'm a bit fast here. Allow me. Anyone have finished the Q &A pair generation? Can you raise your... Okay, great.
02:04:06
Great, great, great, great. Anyone can... is able to click on part. publish as a data project and landed on this page? Perfect. So now what we do is to click on the prompt column and response column.
02:04:20
This by default already picked up instruction. If you forget about the data, you can click on this preview. So this is just the data. And then now we can go into save and next. Exactly. So it's all our platform is to provide you guys a more about the template to design.
02:04:41
Later on, you can just customize just like what we shall showcase the type line. If you are later on getting more focused, you can fine tune those parameters. But based on the Q and a typical pairs fine tuning, we will prepare all this analysis one by one for the data preparation from augment the data to clean the data to check whether it is a forward sexual or a harassing word, length, quality, sensitivity data, so on, we can simply by clicking configure.
02:05:11
But in the future, if you want to modify some of the pipeline very straightforward, you can, for example, just like, let's say, chunk it, right click, delete. And then I want to maybe check on the language.
02:05:21
Just move the language here from the left -hand side of the tool panel and then connect it to that. Once it's done, click Configure. Then here, what we're trying to do is to basically design the pipeline one by one.
02:05:35
For example, if you want to filter a certain column, we want to add more depth test cleansing job, so on and so forth. Poffernity check, the threshold, length, so on and so forth. I'm not going to go through all the things, but feel free to explore.
02:05:53
You can even add your own code, meaning that, for example, in your industry, maybe you are from banking, you are from government, there is some code that you want to modify to apply on your test data to clean it.
02:06:05
You can also put your Python code here as well. Last but not least, you can output the data set itself. For example, the output type can be JSON, CSV, or packet file, and then we can click on Reveal.
02:06:18
This whole platform itself is not only supported by UI, you can also import the library connecting to that. These other parameters are already generated, so you can put it into your Jupyter Noble, RStudio, or your NEID.
02:06:30
Once it's done, click Run Pipeline. Then the process right now on the top right is going through step by step. Okay, so in order to just make sure that we understanding the processes, how many roles are being dropped out based on certain functions, so we also output this particular visualization for you, from input to output, how many roles are dropped, also a percentage as well.
02:07:11
you One important showcase here is to click on this show intermediate features. So if you talk with from to on, you will also see that the dataset now generate a list of different new columns. Each of the content, what is the profanity?
02:07:30
What is the flash gray? How easy to read as a nine grader, six grader, the instruction name, response name, so on and so forth. Some of, sometimes this is important for your data analysis or your fine tuning part.
02:07:43
If you're okay, then you can click on download the CSV file and voila, you have the dataset. You can basically take that into part to your fine tuning studio in the LM studio. Any questions so far for the data studio?
02:07:58
Any like stuck? LM. We will talk about that in the details in the lunch, the backend design. Okay. Then last but not least is the, because we also have the evaluation, Shivam already very fast to show that.
02:08:20
Just pay attention later on. You fine tune the model, you want to compare the model. In your notebook, you will see that you are using model A, model B doing like a AI as a judge to look at the content.
02:08:33
I just want to show one more thing is these evaluations, GPT is like when you have multiple prom, sometimes to evaluate a model, a model might involve different dimension. Sometimes you are more like looking at the coding perspective.
02:08:48
Sometimes you're looking off the math perspective. What we provided is to create a list of prom to test out the model that you built. So if you click on the response column, this is publicly accessible.
02:09:00
Simply just click, just type eval GPT .ai and you will have something like this. What it does is like, for example, if I click on select a new prom or I click on next prom. So now you can see that, for example, this is a very simple question, a math question.
02:09:20
And now you can compare. This life, for example, I want to compare GPT 3 .5 turbo and Vecuna 33 billion and then show the GPT evaluation. Then what we do is like providing the model score. Again, AI model as a judge also will give you the reasonings from step one to step four.
02:09:44
And then further thing, you can remember Jen talk about the application building. You can also build more application plug into this and then starting to finish the whole flow and then to customize the UI, how this model is better and so on and so forth.
02:10:04
So this is basically the whole picture, not the last part. We will finishing with the quiz. I will basically pass into Sifam on top of this, but just want to show one more part is to Of this whole four labs In the future when you have some document or whether in your database or your HDFS You can bring it into our data studio to convert into QA pairs to clean the data to prep the data well And you can put into the vector DB which already included and then put into our enterprise GPT to do the rack You can also fine tune it with our LM studio with your very confidential data And you can then put into our end user to do some query question and answer Evaluate based on our Eval studio and finally using our app studio to build different your own company applications I will pass it to Sifam for the quiz and This is the last part and then we can go have lunch Thank you Timothy Thank you everyone for attending all the labs and sticking to us till this end.
02:11:16
It's almost three hours of intensive hands -on activity. Well, this is still not the end. There is still one more thing left, which is the quiz and the certification. So all of you have gone through various concepts, various topics.
02:11:29
What we have done, we have also designed a quiz based on the today's topic and all of you will be able to try the quiz and people who will succeed, they will get a set of questions. Certificate delivered to their emails.
02:11:42
So this is the QR code. I request everyone to take their phones and open the quiz and start answering. So we'll have about 10 minutes for this. After this, we'll do closing. Lab assistants, if you can take your phones and give the QR code easily directly to the people that will work.
02:12:41
Let's give us a little bit of this guys. While they are fixing that issue, any questions on any of the trainings that happen so far, you need any explanation? I can ask any of my team members to help you.
02:13:32
You can raise your hand. I'll give you the mic. So, if you have a question to ask. Any questions for the trainers? I know quizzes are always fun, right? If you can use H2OGBT to answer the quiz. I actually, this morning I was actually, I uploaded my own presentation, right, to see what the presentation is about.
02:14:38
And it was not a pretty, not bad answer. So it's actually reasonable, like for example I asked the question, what is the, what is the team of the presentation? It's actually was not too shabby. And then I asked the question, how do I do evaluation of models?
02:15:04
And it gave a decent presentation, decent response of my own keynote, right? So, which as you know, that only one liner prompts. So I managed to somehow manage to get good answers. I asked what are the top customers of H2O?
02:15:21
And it's not hallucinating, it's reasonably accurate. So, hopefully go home and use from our app store, the platform and deploy it for good use of the world. Which brings me to kind of the closing section.
02:15:43
We're all patiently been working for the last four hours, right, five hours. There's lunch downstairs. When we've been trying to find correlation, right, in the world of noise, correlation is powerful.
02:15:58
But causality is actually really what drives decisions. So ability to kind of piece why something is happening. Going from correlation to causality is actually going to be. the driving force. That which cannot be expressed in words is feeling.
02:16:17
Feeling is actually right below silence, we talked about silence in the morning, feeling is just right one bit below the silence. And usually music is the only way to capture feeling. Words, already you lost a lot of the meaning.
02:16:35
And if you think about LLMs, they mostly focus on words, right? And then of course, how do we go from finite to infinite? Because data science is really search for truth. And with that, I want to kind of play two deeply powerful ones and closing in closing.
02:16:59
Talk about play Mangalam, which is the final closing session. Garai Purwa Thank you.