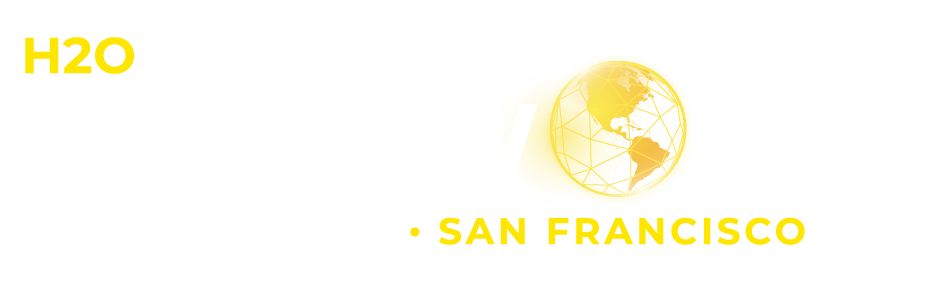
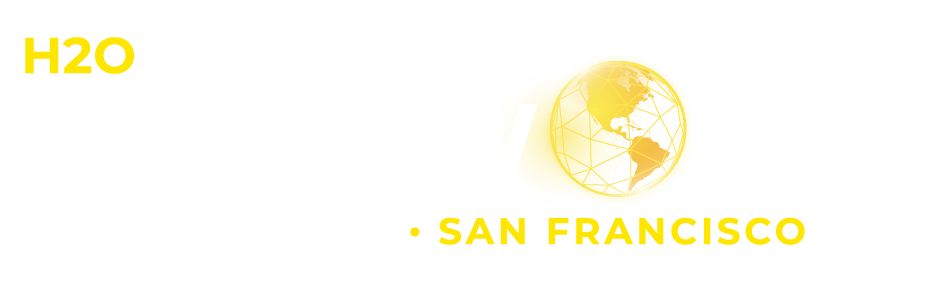
GenAI Fireside Chat
Speaker Bio
Murli Buluswar, Head of U.S. Consumer Analytics at Citi, is a strategic leader in financial services known for driving commercial success through data-driven decision-making. As the former Chief Science Officer for AIG, he played a crucial role in building a data-driven internal change management team. With a background at Progressive, Farmers Insurance, and Capital One, Murli combines analytical expertise with an MBA from the University of Chicago Booth School of Business. Beyond his professional achievements, Murli actively contributes to not-for-profit initiatives, serving on the Board of The Harmony Project and the NYC Tri-state Board of Pratham. Based in Tenafly, NJ, he is recognized for his leadership in analytics and commitment to community service.
Read the Full Transcript
00:06
I don't know if folks know that Rob is a quadruple grandmaster, right? When you have enough Kaggle grandmasters, you have one who's introducing others as well. It's Murali on the call. Hi everyone. Let me also introduce Keri.
00:31
Keri's been a partner in our journey over the last, better part of the last decade. She's a fellow and the day I met her, she already had a badge into city because she's been helping city modernize over a long part of her career.
00:50
So Murali, I was just introducing to the audience your iconic career across insurance, cards, and now city might be worth hearing your, probably a simple kind of how, a simple exposition on how you're bringing organization -wide transformation with AI at city and how you have pursued that over the course of the last, almost good part of your career over the last couple of decades.
01:27
Thank you. Thank you, Sri and Keri. First of all, good afternoon everybody. It's a pleasure to be there with you virtually and by audio, even though it's not on video. The way I sort of think about it is if I may give you a 30 -second perspective on how I thought about my career and then we can jump into the big question, the big theme that you've been exploring today around Genitive AI if that works for Keri and you Satish is that at the end of the day, if you think about large institutions, particularly mature firms, of artificial intelligence and machine learning and data -driven decision is still has not taken full grasp as one might hope for given all of the innovation that has happened in the broader universe.
02:21
And why is that? I think it's because essentially you've got operators who might not necessarily have the consistent curiosity and imagination and you've got highly competent technical talent that understands the tools and the technology and the opportunity for us particularly in a world of generative AI that I see is this ability to connect the two by bringing that intersection of curiosity meets data meets insight decision and large using AI.
03:03
day and what are your thoughts on generative AI in the bank? Good question. So the beauty of being in banking despite the obvious sort of challenges of being a highly regulated industry is that we're a very high interaction, high transaction industry.
03:23
So we collect quite a bit of data and we have many avenues in which we can engage and understand customer behavior. And the way we thought about generative AI in particular is literally if you sort of think about it, most obviously it is a code and content generation.
03:44
It's delivering contextual intelligence to our call center reps and people that sort of need it in a timely fashion and it is extracting and summarizing and classifying information that is typically manually processed by people.
04:03
Now that's a big task when you've got an institution with a couple of hundred thousand employees. So how do you sort of put some framework around that? I think the way to put a framework, the way we thought about it is first of all start by identifying a few material opportunities.
04:21
Frankly, Sri, I'm a little bit allergic to the phrase use case or proof of concept or pilot. The reason I'm slightly allergic to that is this capability is here to stay. Now it's a question of how do we make it work and how do we make it work on scale, i .e.
04:39
let's take on the big gnarly problems that have big economic value at stake. Let's actually work through all of the issues that are normal when you have a new capability that you'll have to muddle through and then at the end of it you come out with a set of outcomes within a nine months time, six to nine months time horizon that give you the confidence you to then be able to create that next wave.
05:06
And the way we thought about that wave, first wave of problem three is, is there data exhaust? For example, voice from call centers. Is there a customer impact or a regulatory issue at risk? Is there a significant out -of -pocket cost?
05:26
Has that process remained highly human intensive for the last decade and such? And do we think that we can actually drive material innovation within a reasonable timeframe with very well -defined metrics of success?
05:44
Whether those metrics are financial metrics, or whether those metrics are operating metrics, but having a clear definition of what the success looks like, six, nine, 12 months from now for each of those endeavors, through the lens of a CEO, a CFO, and head of audit?
06:02
The CEO notion is important obviously because of materiality. CFO 3 is when and how will it hit the financials and head of audit is traceability slash attribution. So we've actually come up with a first round of problem statements that hit our threshold for materiality that also then allow us to build foundational capabilities that can be paralleled into multiple other problems slash opportunity statements.
06:33
So one of them is around thinking about how do we enable our call centers agents more effectively. Another one is around content extraction and a third one is around summarization and pulling together information that currently is being done by humans in an inefficient way and in a partial way quite frankly.
06:56
So that's how we thought about it for each of those endeavors. We have very well defined metrics. And so what I enjoy about this conversation with you, hopefully from the audience perspective is that it's giving a little bit of a complimentary view relative to the deep brilliant technical intelligence that is on full display from many of the speakers in the room.
07:19
I'm not nearly anywhere as qualified as they are technically, but hopefully my commercial perspective is of some value to the team. That's great, Meryl, thank you. When you think about particularly being in a regulated industry as you are and you mapped out executive stakeholders and then how you're approaching each of the problems, how do you think about value creation and getting whatever the stated goal is from those problems?
07:45
How do you think about balancing that versus risk and regulatory oversight? Excellent question. So this notion of risk and regulatory oversight has always been there. It's been there with machine learning.
07:58
It's been there with traditional AI and it'll be there all the time. also with Genitive AI. The way we've thought about it is this is an extension of the operating discipline that we've had historically with the one exception that we in our first wave are not focused on, if I'm being blunt, we're not focused on necessarily revenue generating opportunities as much as cost mitigation opportunities.
08:25
And we're focused on avenues of value that are more internal process focused as opposed to customer focused. So the big need for engaging with legal, with model risk management, with privacy, with compliance and ensuring that we are doing every bit and then more to mitigate, if not largely eliminate unintended consequences and bias in our algorithms, those issues have always existed.
08:57
They just take on a new level of scrutiny with the world of Genitive AI. The other big thing that I would say that is important for us as we have started embarking on this journey is to have a monitoring discipline and to keep a human in the loop so that there is some human oversight as we make that evolution from human to at the end of the day components that are fully automated but ensuring that that transition with a human in the loop is allowing us to bring the best of AI and HI.
09:31
Excellent. Thank you for that. I think I've had the conversation maybe five times today so I want to put the question to you. When you think about how you roll this out, you know, an organization of your size, you know, do you put this technology in the hands of everyone or do you keep it to the experts and deliver applications?
09:48
Is it a mix? How are you creating a strategy around the implementation process? And, you know, we have a lot of different disciplines in the room and listening in on the live stream. So some are regulated, some are not.
10:00
What are your thoughts in general on this space on how to actually get this technology into the hands of your teams? Outstanding question because the thing that we need to juggle is one is the stoking of culture of curiosity and learning where ideas and understanding of what this capability could do does not reside in any particular function or group of individuals rather that needs to be democratized.
10:27
So having points of view and input from a wide range of employees across different functions that are A, feeling more inspired and enthusiastic about understanding the power of generative AI and B, connecting that to their universe through a lens that we might not yet otherwise is supremely valuable.
10:49
On the other hand, you cannot allow a thousand flowers to bloom at the onset. The reason for that is there are limitations around ensuring that your function is not limited to the use of the technology.
11:02
finding that right balance between a public LLM and your own internal data. There are limitations around access to GPUs and such. And really the way to sort of build this muscle is to sort of think about materiality and say look what are the 5, 10, 15, 20 problems we can solve in the next 6, 9, 12 months that will move the needle, that will build the muscle and that can dovetail into a whole new level of thinking around additional opportunities that you might only vaguely conceptualize.
11:36
So taking a little bit of a measured approach that bifurcates between the need to create a culture where people seeks to understand and learn and contribute to a broader basket of opportunity identification but yet balancing that with the recognition that you have to go after your big ticket problems and opportunities and in order to do that You really need to be able to orchestrate whether it is technology or whether it's data science or whether it is a product function or whether it's finance and operations and such.
12:10
That is a tall order because at the end of the day, you're reinventing what decisions are made and how decisions are made. And that reinvention means that people's roles are being redefined tomorrow relative to what they were yesterday.
12:25
And so understanding that human element and thinking about the scale of a particular problem that you're solving and having the humility to recognize that that's not just a technology solution. That's a human solution that needs to be able to bring in a very measured view of how do you sort of face success in a way that ensures that you're not undemently disrupting things in a way that creates unintended adverse consequences.
12:57
I don't know if that answers your question, Barry. Yes, absolutely. This question is actually for both Kerry and for Murli. And this actually goes back to Murli's comment. He is a huge fan of radical customer empathy.
13:17
And Kerry here also is nearly 100% renewal rate through our career at H2O. So I think maybe, Kerry, you can start off. What does radical customer empathy mean for you? And of course, then, Murli would be nice if you can add a couple of sentences on that.
13:39
Yeah, thank you, Shree. I learned that from Murli, radical customer empathy. And it really does touch, I think, the heart of a lot of us at H2O. Our culture as a maker of culture, it really hits home.
13:50
We believe that our customers are children of the company, right? And Shree says that all the time. I steal that from him. But we do really mean it. And I think what it comes down to is really listening because What we're learning, the innovation that our team brings forward, and I'm certainly not on the innovation team, but the folks who are bringing forward the innovation, it's happening so quickly.
14:08
So being able to listen to our customers and understand not just where they are today, but in fact, where they're going. We've built a great partnership at Citi with Merley and his team. Being able to be on the front lines of some of these conversations that helps influence how the product gets to enterprise grade.
14:24
So we have great ideas, great solutions, amazing open source tools, but how do we make that open source? And I think that's leaning in and listening to our customers. And this is a very interesting time, things are moving very quickly, people need to deliver results, but there's also a lot of caution.
14:40
So finding the right balance with our customers is a journey. It doesn't happen in one conversation, and hope it happens over the course of the relationship. And as we develop new tools, I think we can really make that grounded in solutions for our customers and make the value for H2O bigger than just a software contract.
14:58
And Merley, I love your opinion on that. Thank you, first of all, Kerry, you're too kind and generous in attributing that to me. I will not take credit, but it's very kind of you. And the other thing I want to say in the spirit of radical empathy is I'm looking very, very good right now, so I feel terrible that the audience cannot see me, but some of you might think it's a gift to you.
15:22
I don't know about that, but be that as it may. For me, it is, if I step back and I think about your questions three more broadly, and I'm going to think about it in the context of B2C, but the same principle obviously applies exactly to what Kerry was saying a moment ago as well, is what is the fundamental mission of any enterprise?
15:46
The fundamental mission is to build a sustainable, mutually economically viable relationship with a set of customers that are fulfilling both in terms of economics, but also fulfilling on other dimensions as a bank to a consumer or HTO in a B2B relationship.
16:08
That's the overall mission, because if you can stick to that mission and that purpose, good things actually come out of that in a way that allows you to sort of think medium and long term in the investments that you make and the choices that you make in terms of how you engage and relate to your customers.
16:27
Bringing that to the world of data science, my philosophy specifically is that the beauty of AI is that you ought to be able to infer customer needs without necessarily having them pound the table and overtly always articulate that to you.
16:47
And so every interaction and transaction that you might have with a customer, whether you're in healthcare, whether you're in banking, whether you are in consumer goods and such, you have an opportunity to say what is the latent significance that the customer is sharing in terms of their needs, their interests, and how do I draw that intelligence through data science to be able to engage with them in a way that mirrors back that radical empathy, even though they're not overly asking for it.
17:18
That world is magical because that world is a world where we are solving for the unmet and unasked from advanced analytics. The more we can actually do that, the more we can build an enterprise that is going to last for decades or even centuries.
17:45
Fabulous. I mean, towards that beautiful world of, using your word magical world of customer, deep customer relationships and building trust. You're all in the business of building trust as opposed to building trust.
18:00
only making our quarters, Richard. So I think it's a very fantastic kind of team that we picked up from you, Morley, over the years. And thank you for, despite, I don't know, power outage. I'm glad you were able to make it.
18:16
Hopefully next time we can see you in person and you'd inspire our audience even more. But thanks for taking the time and thank you, Kerry. Look forward to building a fabulous relationship with you and over the years with the folks who are ardent followers of you across the industry.
18:35
Thank you. Thanks, Morley. Thank you, Kerry. It is such a delight. I am grateful to have you in my ether, both as business partners, but also at a more human level. The one thought that I might share as a parting thought to the audience is it is important to spend an inordinate amount of time understanding the technicalities of how we trade off hugging face with Lama.
19:04
To what extent do we rely on public LLMs versus frankly building our own internal, call it SLMs when you're trying to solve for particular problems. So understanding the nuances of that, understanding the risks and the implications of how you find that needle in the haystack.
19:23
If you've got a LLM with 70 billion parameters and not allow that noise to creep in is very, very important. At the same time, if we can all build that muscle of being equally enamored with solving material problems that are either deeply commercial in nature or have wonderful public policy implications, that to me is sort of the true north.
19:49
And the more we can invest in in parallel, using both sides of our brains to think about the technology and the data and such on the level of the LLMs. left brings, and at the same time, reflect upon what are the big problems we could solve and how could we solve them and how do we develop that curiosity through this and be able to reinvent business models and institutions.
20:15
That to me is the ultimate promise and the beauty of Gen AI is that if I may put it blithely, there's a bunch of people that I have known in my life that I thought were atheists now are deeply religious.
20:27
That's the promise of Gen AI and we are not even scratching the surface. So very excited to see how H2O plays a pivotal role in that and look forward to benefiting and supporting and engaging with you on that.
20:42
Thank you. Thanks Irwin. Thanks Murli.