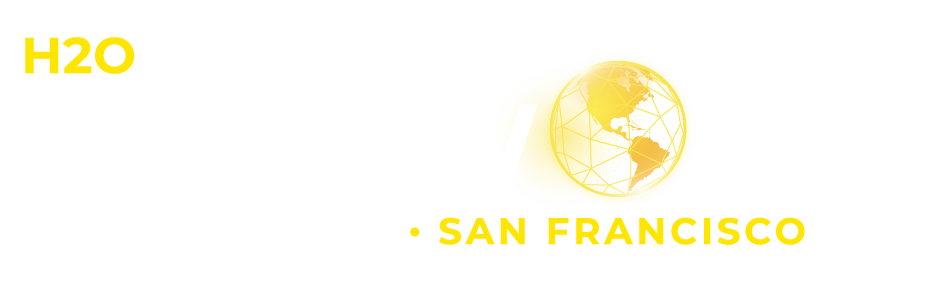
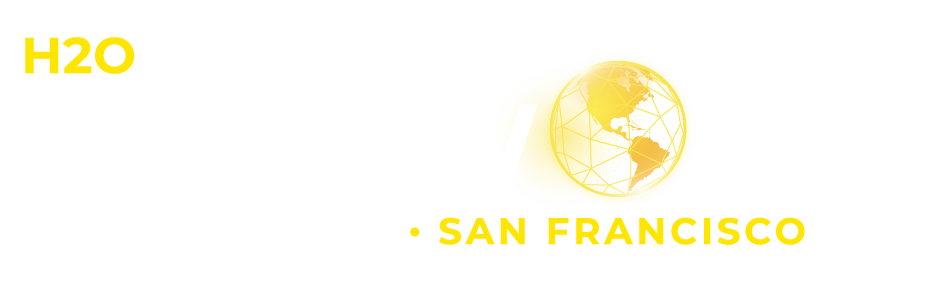
Kaggle Grandmaster Panel
Philipp Singer, Data Scientist, KGM, H2O.ai
Mark Landry, Data Science & Product. KGM, H2O.ai
Ryan Chesler, Principal Data Scientist, KGM, H2O.ai
Sanyam Bhutani, Senior Data Scientist, KGM, H2O.ai
Pascal Pfeiffer, Principal Data Scientist, KGM, H2O.ai
Dmitry Larko, Senior Principal Data Scientist, KGM, H2O.ai
Arno Candel, Chief Technology Officer, H2O.ai
Rob Mulla, Senior Principal Data Scientist, KGM, H2O.ai
Kim Montgomery, Senior Data Scientist, KGM, H2O.ai
Shaikat Galib, Principal Data Scientist, KGM, H2O.ai
AI-generated transcript
00:08
All right, this is a highlight. We have more than 10 Kaggle Grand Masters on the stage. And we have 30 in the company or so. I don't lose count sometimes, but it's roughly 30, and we have 10 here. And we have an honorary guest today, Mikkel, also known as Anokas.
00:26
He's from the UK, and he made it to San Francisco just in time for this event, so welcome. Welcome, everyone. Let's give them a hand. And I'll spare your introductions, but everybody has deserved tons of goodwill from the community, because they have not only shown that they're good at competitions, but also have shown that they are good at teaching others how to do Kaggle.
00:56
And I wanna know from each of them, why do you think Kaggle is relevant, as opposed to just being a toy, like a hobby or something? How does it actually help in the real world when you're doing work?
01:12
So, to me, Kaggle is a place you can actually learn about the domain you never worked in. For example, you wanna learn about the image classification, or like, I mean, image, working with image in general.
01:28
Kaggle can be a good place to start learning about that in somewhat close to real -life scenarios. Just because you have other people competing, and you have to really do a good job, is the modeling, data preparation.
01:41
You can learn a lot in the process, actually. So that would be my take on Kaggle, relevance to real world. I also think it's really good because it forces you to be disciplined. You don't get to decide how you're going to evaluate your models, you don't get to decide, oh, I'm doing, you know, I've set up this dataset, but I've accidentally trained on the test set, and I get 100%, so I'm happy.
02:05
You really have to explore all the possibilities. abilities and like you said, it's a great way to learn because of that. It's definitely a good way to just get exposure to lots of different problems and intuition about what you need to do in terms of feature engineering or what models work best.
02:34
Yeah, it's good as far as understanding things like leakage and how that can really be problematic. I think for me it's the knowledge of good validation. So in Kaggle you always have public leaderboard which is available to everyone throughout the competition and only in the very, very end when the competition already stops you see the private leaderboard with the actual team.
03:06
score that you are being ranked on and where the medals are being distributed on. So you can easily be fooled by having a good spot on that public leaderboard, but in the end your model is not generalizing enough.
03:21
You're basically overfitting on that public leaderboard. And you really need to think about a good local validation too, to support your good public leaderboard score for example, to be sure that you won't drop on private leaderboard.
03:38
And I think everyone on Kaggle had that one competition where they dropped like a thousand places down in a competition. And that's exactly the point where you question your own thoughts and what you did wrong in that competition.
03:51
And that's like the most important piece of information that I took from Kaggle. And it didn't take many competitions to learn that the hard way. For me Kaggle is like a learning playground. When I do Kaggle competitions it's like learning new techniques, new methods that people are talking about or which is disrupting technologies.
04:25
There is a good place to stay motivated and learn the technique or the method until to the deep domain. Kaggle keeps me motivated in the competitions to get updated and also make valid models so that it doesn't overfit in real world applications.
04:47
Also, we can learn where the limitations of these models if we want to implement it in real world software. Many solutions are complex enough that we cannot actually implement this in real world software.
05:06
but how can we make it simplify and still usable in real world applications? That's what Kaggle gives me, learning the methods, stay updated and keep validating what methods works would work in the real world applications.
05:25
Thank you. I think for me Kaggle has definitely helped me with the workflow, the process of getting the data, cleaning it, putting it through a model and stuff like that and then figuring out if things aren't going as well as maybe I see someone else doing the leaderboard where I need to go back and fix things.
05:50
I need to go back and clean the data more, do better feature engineering, find a different model, go read some papers about the topic and figure out where I can fix whatever has gone wrong. I would agree with everything they've all said, so I'll throw something different out there is that sometimes in the real world when you want to take shortcuts, you pay the price for that too, but it's harder because there's no leaderboard telling you.
06:16
So you might short circuit something, you might look at your training, you might burn too much of your training data and not set up a validation set just to see what's out there and then you start to see, oh, this is doing really well and you start to eventually check it out and you realize now you've got to go back to the same basics that you learn in Kaggle like Pascal said.
06:33
I mean, I'll try to give a different answer. You go to X or Twitter and you see like 10 ways to make a million dollars using some new tech and then you go to the leaderboard and the leaderboard doesn't lie, right?
06:46
I've had the privilege to interview everyone on the stage and it's like a real degree, right? Like there's a, the solution between the first place and 100 places ever so slightly different, but like in those fine details, you learn what's the best, you like learn what's the best technique.
07:01
So leaderboard doesn't lie. Yeah, I think everyone said such good things. One of the things that I like about Kaggle is just the competitive nature of it drives the innovation. So when you launch, when a Kaggle competition is launched and I've been on the other end of helping launch them, you don't know what the best model is and a lot of times that's the way it is working at companies.
07:26
You don't know what the best model is, but it's amazing to see the competition really drive the score up beyond what you even thought was possible when it was launched. And to me, what some of the most amazing competitions are the ones where the winning team is really a lot better than even the second best team just showing that they found something that was always there in the data, but the competition really drove it out.
07:56
I think for me, Kaggle is really interesting because it's just been exposure to many, many different problems. I looked at my profile the other day and I downloaded the data. from more than 100 competitions, and I've read all of the top 10 write -ups, and so I've seen the best of the best on every single different problem, and so it's been really valuable in terms of exposure and understanding how you format a problem and data and models and all of that stuff and how the best people do it.
08:23
It's been phenomenal for learning all of that stuff. Yeah, most has been said. What I want to add are two things. The first one is teaming, so I really enjoy teaming on Kaggle because it's not only about learning about techniques, but it is also about getting to know other people and also learning how they are working, which I find personally very interesting because everyone has a slightly different approach, and you learn something on Kaggle.
08:56
I have learned a lot about engineering because there is a lot of resource constraints. For example, on Kaggle, where you need to put... It's very similar to putting things into production. So yeah, that is definitely also for preparing your own training framework, pipelines and so on.
09:15
So there's a lot of engineering also that helped me. Thanks. Do you have any suggestions for someone who might not be a Kaggle master or grandmaster, someone who's just a regular practitioner? Any tips, any secrets or any insights?
09:34
Anything that stuck out in the last few years? Like, or something you wish you had done earlier? What would you tell yourself? Well, I would pay more attention to math class in school, that's for sure.
09:49
But in general, I think it's, I would say, my key advantage is the persistence. So you just, you know, you just do a competition over and over again. You don't give up, you continue trying different ideas on the same data set, let's say, right, for entire competition lengths.
10:07
And that usually helps a lot. Yeah, I think my main advice would be, don't be afraid to just start with other people's code. Look at the previous solution write -ups. Look at the kernels, because people upload really good code all the time.
10:23
And especially if you're just starting out, it's like a really good starting point. You learn what they do, and then you can tweak it, and you will slowly build up skills from that. Because it's actually quite hard to just come into random competition on the site and do it from the start.
10:39
It can be quite daunting a lot of the time. Yeah, but I'd say also don't just use other people's code. It's good to try other ideas. I always thought it's fun to learn something while doing it, because I'm pretty bad, I would say, by following the code.
11:03
following some tutorials or some books. So I was super happy about Kaggle when I found it, when doing data science, because it actually put me into that spot where you can just do something. You might fail, you probably fail at first, but then after finishing that competition, I don't know, somewhere in the lower ranks, and reading up on these top solutions, you can actually learn exactly which points you didn't get during your solution, and where you can improve for the next challenge.
11:37
And you always take a little piece from each competition that you're competing in, and can adapt that to every other subsequent competition. So that is very, very important. Okay, so I started Kaggle competition like six years back.
11:59
That time it was far less competitive, far less... contribution was posted in Kaggle forums or in the code section. So most of the time I need to write the code and find relevant materials, read through articles.
12:18
That is still relevant now but a lot of people are contributing to the community these days. So I think if someone wants to practice data science and want to be better, they have multiple options to start looking at.
12:34
There are multiple good code contributors and there are discussions people discuss about their findings and they're really good. So yeah just spending some time in Kaggle definitely should make some aspiring data scientists better in their work or job or just simply can make their knowledge better.
12:59
Yeah so I guess like advice to my younger self starting in Kaggle would be to stay organized more because I go back and look at some of my old code sometimes and it's just a mess compared to what it is today or at least my organization and the directories and whatnot.
13:20
Even it's not as good, it's still bad today but it's way bad back then. So yeah that's one thing I would tell myself it's way easier to go back and find out or remember what I did like two months ago in the competition and stuff like that so I don't go back and repeat it and whatnot.
13:35
I would say for getting started for me it's like you kind of it's a little bit of a function of time for most of us and you'll hear that in other times like we put a lot of time into these competitions and most of the time the winners do so but you don't have to go for a win you don't have to go for a silver or bronze medal you know go for whatever you want like sometimes if I'm going in there and I think there's something fun but it's just too late I know when I'm not time you're just gonna work yourself from 300 to see if you can move up 50 spots in a few days or something like that, because you're going to learn something about that piece.
14:06
And then you want to see how that holds with other things. And so maybe it draws you in and you pick up something different each time. But some advice would be it's not easy to win these things. It's not easy to do well in Kaggle.
14:18
So don't be dismayed by that. But find a little pocket where you can keep learning. And as everyone said, the code has just gotten better and better and better. So my suggestion would be read the people that are on the stage's solutions.
14:36
And Team Hydrogen usually always opens sources their inference code or sometimes even their training code. I would start there. And that's always literally a goldmine of knowledge. Yeah, I think similar to that, there's a lot of information in the winning solutions on the discussion boards.
14:54
But use LLMs. Now that they exist out there, they can help you answer some questions that you might be stuck with, or they may hallucinate and you'd learn something by figuring out what it tells you is wrong.
15:09
But I've found it really helpful to use LLM's to even take other people's code and have it explain it to me and add comments. It's used what's out there. There's no better time to get started because they're out there.
15:25
I think one thing that I've seen from a lot of the people that are successful in Kaggle and just generally data sciences, they figure out how to iterate quickly and they figure out what's important very fast.
15:38
So if you look at the winners of Kaggle competitions, very often it's the person who trained the most models or tried the most things. That's really a lot of the successes figuring out how you explore very quickly a lot of different options and figure out what the key factor is.
15:56
Yeah, like Rob said with the LLM, if you paid attention, I'm not sure if you could read it, but that was my standard example on my slide. And also when LLM started to get out because this is a question I get asked a lot how to start and it's a good question to ask LLM's.
16:13
They give nowadays, they get better in this, they give a really comprehensive guide to this first, to that. I mean every person is different in the end. Maybe on a different level just don't be afraid.
16:25
It's easy to say, but don't be afraid of like placing badly and don't let that be the reason to not do it because it doesn't really matter. Just have fun and learn things. Awesome, maybe a bonus question.
16:43
What advice would you give customers when you deal with customers, you often see them doing something not ideal according to your standards, let's say. How can they avoid making those mistakes? What would you like them to change?
16:57
Do you have any such advice? suggestions? I would say really focus on the evaluation and the metric and stuff. When you're working against a real -world problem you have to make sure that the thing that you're optimizing is actually the thing that you want it to do in real life because otherwise you'll deploy it and it will behave very weirdly.
17:20
Yeah, I'd say on that note too that if you're gonna spend the time on the metric as a company you can hold out a test set similar to Kaggle and have your data scientists work on their solution but then validate on a holdout set.
17:40
Yeah, similar. Just be careful about validation and make sure your validation sets similar to what you're trying to predict. And maybe one fun thing because leaderboards are always something that drive data scientists crazy.
17:56
So if you have something like a metric in your company, just put that metric up, give that validation set to your data scientist and they will go crazy just beating that. So that's really something that is driving Kaggle and should probably also drive companies.
18:19
All right, do we have a question from the audience? Okay, so if you have a synthetic data set that you would like to make for Q &A, for fine -tuning, let's say, how can you make such a data set for LLM fine -tuning?
18:56
Oh, there are some other questions. So let's start with that one first. Any suggestions? I can try. Yeah, it's a very tricky task. Evaluation as we have heard throughout the day in LLM space is explicitly difficult and which also drives us Kaggle a little bit crazy, right?
19:17
Because we love to optimize fixed metrics and this is a little bit difficult in the LLM space. But like I said before, try to figure out what are your actual KPIs for this use case in the company. Try to come up with a certain type of evaluation that kind of matches like metric -based, matches this KPI and then generate a data that is closest to your expected production data and then try to optimize that.
19:48
However, that looks like, right? And LLMs can be really useful for generating these synthetic samples. You just need to steer it into certain... direction and usually that works well. But there is no gold answer to that question.
20:03
It's usually a process that involves several different steps. Yeah, and I'll focus on something he said, closest to production. That's a really important thing for everyone. So what mistakes I've seen customers make is go get something from the internet, train a model, and then realize that your production data has some very big differences, not even small.
20:22
And there's all sorts of ways that can play out. But really pay attention as early in the project as you can about figuring out how you're going to deploy it and what that looks like rather than testing in a little experiment.
20:33
Maybe a good idea, but don't go too far with that. How do you see the world of Kaggle evolve in the next few years? Like is everything going to be agents and LLMs, or will we stay with video and image?
20:52
Well, yeah, I believe in agents and I actually would like to see some competitions on Kaggle, which agent -centric competition. Because I do think that's the next big area of research, that's going to be agent -based solutions.
21:10
Well, I hope we still get competitions that aren't dominated by LLMs. Yeah, I definitely hope to see some tabular and time series and a little variety at least. As I always like to get new challenges, new types of problems, I wouldn't mind agents to be one of the topics.
21:36
Yeah, it'll be fun. My question would be even if you have agents, then what's differentiating the top solutions then? If everyone has a super smart Philippe or Pascal agent, how's like the winning solution coming on top after that?
21:58
And I think they'll figure out a way Kaggle is always do. Yeah, I guess one of the ways I see it changing that doesn't necessarily involve LLMs is there's been a lot of focus in some recent competitions on how fast your model can run and limitations on the size of the model.
22:22
And those sort of things that Philip was saying he's learned a lot about engineering doing competitions, I think that's going to become more of a part of it. Not only having a good model, but having a good model that can run quickly or can run on edge device.
22:38
Those sort of things are easily just added into the competition design. And I think you're going to see more of that, especially with kernel competitions. Yeah, I mean, as I've shown in my talk, LLMs are already there in problems that we have solved without LLMs just half a year ago.
23:00
Also, we are using now LLMs in our daily work for coding. We are using LLMs to generate data. This is a big thing also for competitions that you can generate extra data with LLMs. In the computer vision space, we haven't seen it that much yet on Kaggle, but this might be also the next step, and then also multimodal things.
23:22
So yeah, there is a natural evolution. And maybe in half a year, everything is different. Who knows? Awesome, thank you very much. At least we'll have some jobs left to do in the future, and it might be Kaggle.
23:36
Thank you all. Thank you.