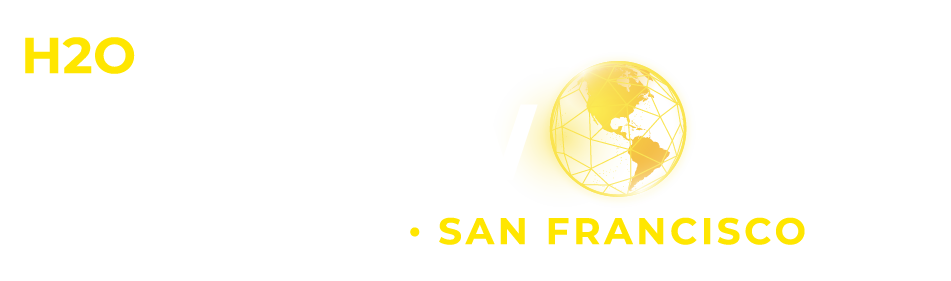
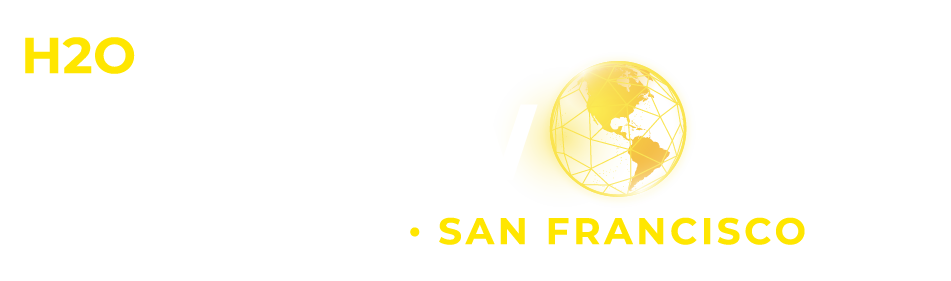
LLM Fireside Chat
Speaker Bio
Helena Fopiano, Director of Customer Strategy and Analytics at ADT, is a seasoned professional with over a decade of experience in the field. Based in Boca Raton, Florida, she currently leads the Customer Strategy & Analytics team, driving revenue and profitability while optimizing customer experience. With expertise in data science and analytics, Helena excels in mitigating customer churn, optimizing NPV, and implementing advanced analytics for strategic decision-making. Her leadership extends to recruitment, mentorship, and fostering innovation within her teams, showcasing her commitment to excellence in the analytics domain.
Read the Full Transcript
00:06
Hi, I'm Megan. I'm a data scientist at H2O and today I'm going to be talking with Helena about her work at ADT and we're going to be talking about large language models and her journey with AI and a couple of other topics today.
00:20
So we'll get started. Hi, Helena. So do you want to start by telling us a little bit about your AI journey so far? Sure. Can everybody hear me? Thank you for having me. Absolutely. At ADT, our machine learning journey really started pre -2018.
00:40
And just like the Coca -Cola folks talked about earlier, it was like me, one analyst and an intern. So how can you stretch that team further and do more and by not really having the expertise that maybe you would like to get started on a machine learning experience?
01:00
And that's how we crossroads with H2O. How can we utilize a tool and give us that extra power and lack of experience to allow us to start building models for use cases and improve business value, which is where we are today.
01:18
So we're on our own sort of journey. We have all at our own pace. We're like a thousand years from Auguste over there, but we have made our achievements so far that has given us to where we are today.
01:32
Thanks. And I think one of the things we have been talking about is with machine learning and data science, only a small portion is really the modeling piece. And a lot of it is really how do we get support and explaining a model and really make it more accepted by a larger audience, not just data scientists.
01:52
So do you want to talk a little bit about maybe that as well? Yeah, sure. It's something that's real and alive. And I live and breathe it every day. But being able or a little allowing the data scientists to build the models and then use that to sort of explain it or get the support from the business end users that ultimately are gonna change something in their behavior, typically has a pretty big wide gap.
02:25
You talk to a data scientist and they will tell you all about the metrics, all about the AOC and adding this one additional feature and it is a great accomplishment but for the business end user, it means absolutely nothing.
02:39
So for us it's always been about can I quantify business value? I'm gonna give you this model, I'm gonna tell you to do something different. What can you win by changing or listening to me and rely on the output of this model?
02:54
And there's certainly a high degree level of, should I call it skepticism? Like you gotta prove me right before I buy your product and I protect these products and as aaf to that, I promise you I will allow myself you A good amount of iteration.
03:08
Typically, we don't ever get something right the first time. But if I can improve, that I can improve, and I'm listening to you as a business end user, and I factor something in, and I make it better, and a little bit better, and a little bit better, at the end of the day we're partners, and now you will support me in the next engagement that I want to do.
03:26
So we do this all the time, every day. And I know we're going to get to LLMs, but we went back there, started talking about LLMs too. What is an LLM, and how is it different from maybe a model that you already have?
03:42
So lots and lots of education for us. Yeah, that's true. I know as a data scientist, I think I spend 5% on the modeling, and then 50% of the time on data wrangling, 45% of the time on explaining it. And I'm wondering now, we're going to talk a little bit about large language models, but I think one of the things about large language models is that it's so widely accepted as well.
04:07
It's a little bit different than machine learning, which is more focused on data science, data scientists, building models, but now everyone knows about large language models, and they're already using it in their day -to -day life.
04:17
So maybe we'll start to see some changes now that they're becoming more widely adapted. Maybe it could even be useful to help with machine learning. How do we explain our models with large language models?
04:29
I know we talked a little bit about your journey with machine learning so far, but what about LLMs? What have your experience with Gen AI? So I think like everybody else, we're learning. Everybody doesn't have all the right answers yet.
04:45
I think we're learning them together. For us, it came all the way from the top, all the way from our executive leadership that basically said, how are you using this new technology? How are we reaping the benefits?
04:58
That's great, but without context, it was very difficult for us to answer. So we kind of took this journey of two paths. We started with a... governance path that said, I mean we're a security firm so we tend to be very conservative and it's very important for us around data security.
05:16
So we started a governance path that said what are our policies, how can we make sure that we're doing this safely and securely, how are we not putting ourselves at risk so we created a cross -functional committee with an intake process just to expose sort of all the things that various parts of the organization wanted to do.
05:38
And the other half was starting to talk about or just having dialogue around what are some of the use cases that our business stakeholders would want to see, have interest in seeing, or do you even have an interest in utilizing this new technology or not.
05:55
And I think I heard it throughout many different talks today but where we landed was our initial use cases were definitely text -based. I know we talk a lot about the fun video picture type of models, but they were really text -based.
06:14
And the organization also felt most secure in starting with an internal end user. So no direct exposure to our customers, but how can I expose it to somebody internally that in their turn is treating or maybe talking to a customer?
06:32
So that's where we're at today. So basically still keeping that human in the loop, but trying to accelerate or speed up their workflow. And I think you said it really well before. It's almost like a warm start.
06:46
Yeah, yeah, very much. And I mean, again, it has to do with an organization's level of comfortability. And somebody who still does education every day, we're not just going to jump into a limb. It's just too big of a gap for us.
07:00
So allowing that human in the loop or the SME or whatever the use case is to serve as the safeguard was just something that resonated very well. Yeah, that makes a lot of sense. And I think just from being a user of large language models, that might be also an interesting place where how do we kind of fail fast or how do we understand very quickly that the large language model is right or wrong?
07:25
Because if we're especially if we're having an agent or an intermediary using it, they still need to be able to verify that the answer is correct. Otherwise, it's not really an accelerator. So that's something interesting to think about.
07:40
What do you see as the future with Gen AI at ADT? So for us, we're in the process of doing a couple of POCs. I know the previous speaker was not a fan of POCs, but for us, we always have to sort of prove value before we can get funding, if that makes sense.
08:05
Today, the pilots or the small POCs, it's like somebody's side project. And there's always so much you can move by having your slush done going towards LLM work. So around the organization today, there are a couple of POCs there being worked upon.
08:22
In a way to try to prove, here's the potential value we can realize by doing this so that we can get more funding to scale it a little bit bigger. In general terms, for my specific team, we are trying to utilize LLMs towards call recordings.
08:44
For example, unstructured data is not something that we've always had readily available to us. And it's something that we have a lot of. We interact with our customers a lot, whether it's through phone calls, chat transcripts, reviews, ratings, you name it.
09:01
And at speed, be able to. kind of wrangle or summarize this text data in a way so that we can infuse it potentially into already existing models. I know how a use case where I can say this is what my model did pre and this is what my model did post and I can quantify that and I can explain it quite easily to pretty much anybody.
09:25
So the focus right now are various POCs for proof of business value so we can get some more resources funded to them. Got it. So it sounds like the LLMs would really be feeding in additional data into the traditional machine learning approach potentially.
09:43
So very basic what's the sentiment of the customer on the phone but maybe what was their context or what's their, what are they looking for, how many answers do they get, how many questions do they get answered.
09:57
So some things that maybe weren't previously used before maybe just basic metrics are now getting potentially could be used in models for customer lifetime. Yeah very much they would add context. So for example if we have a propensity to turn model suite where we have a lot of numeric data, this is I spoke to this customer so many times in the last three months and so on and so forth by being able to add what the phone call was about.
10:25
It was the first problem that was raised within that phone call on top of very basic metrics such as call sentiment. I feel would have a lot of value but I guess it's still to be proven by us. Yeah that's a great point and I think especially when we use large language models it's almost for an individual task like I want to draft an email or I want to write my resume.
10:55
But if we think about it at a larger scale that's maybe where machine learning comes back into play because you're reviewing this large, large amount of data. of data, these call transcripts, all of these customer interactions.
11:08
And now you want to understand what about them is driving churn or driving customer satisfaction and what kind of customers are going to churn because of the same reasons. So almost that kind of higher level analytics is still the traditional approach per se, but you're using large language models to just generate that additional information.
11:32
Yeah, and for us too, like I said, unstructured data is not something that we've had around readily available for us to use. So we can also use the LLMs to simply try to learn and summarize and classify what is in, whether it's our WAIFI is transferred to transcripts or chat transcripts.
11:54
So even though that isn't necessarily weaving into another machine learning approach, it's a means right to an end for us to start learning. what is in our unstructured data and then be able to fit in.
12:08
How does that now fit into my churn model suite? But contextualize without spending an enormous amount of time is definitely a use case as well. Yeah, definitely. Have you also considered using the large language models to provide kind of a quick overview of the customer for the agents?
12:34
So part of our use case, bigger dialogue, it was something like that very quickly came about. We have a lot of customer -facing agents, and a large majority have less than 180 days on the job. Right?
12:49
And we have this huge data knowledge or corpus of information that we expect somebody to just be able to find, comprehend, summarize, and then dictate out to the customer on the fly. It's very difficult, especially if you're new.
13:06
But back to having an assistant to the agent, could we leverage an LLM in that way, maybe even in a form of a chat, where the agent could find the right information, summarized quickly, and then be able to relay it to the customer.
13:24
That was one of the very top uses that came out. That is also a ginormous use case. So me talking about it is one thing, but actually putting it into production somewhere, having it exposed to thousands of agents, feeling comfortable that I'm overcoming hallucination, right, that's like zero to a gazillion.
13:45
And hence, that is not something that we have begun yet, even though it's more on the, should I call it, the wish list. Yeah, definitely. And I guess that's where we've been talking a lot today about RAG, or retrieval augmented generation.
14:00
So that's where it would come in, because in this case, I'm sure you have a lot of, internal documentation about the customer and about your products and maybe external documentation as well. But you really want that large language model to answer not just generally about ADT but specifically with this you know very specific data on the customer essentially and that's where the ability for the large language model to find the correct pieces of data will really come into play to make sure it's answered correctly.
14:32
Yeah and for us and maybe for many organizations right but whatever idea you have in your mind that you really want to try anything is going to be valuable for you the organization also needs to be ready for it so a lot of things may sound fantastic in a sandbox but do I have the means and the ability to support it at scale do I have the resources to maintain it because if I don't then it's going to fail really quickly and I'll have a really hard time getting its second chance to do that.
15:09
So I'm also being very realistic around my first use case cannot be a massive failure and not going for maybe the biggest, most valuable use case first may not be my best bet. And for us, I mean each organization is at their own maturity level, right?
15:33
And I think for a native team perspective, we have a pretty good idea of what we have resources to support. So the best use case for us will be of fits within that confinement. Yeah, and definitely this needs to have the end users confident in it and believing in it and understanding it and wanting to use it as such an important piece.
15:54
So I definitely understand this idea of trying in a small section, see how it does and kind of evaluate the impact of it. Yeah, that's really interesting. Well, I know we're out at a time. Do you have anything else you'd just like to add for the group?
16:10
No, I would just say if somebody has done a successful pilot, I would love to talk to you. I think we're learning alongside with many others and some of our findings I've heard in previous talks today.
16:24
So it's quite an interesting time how everybody's sort of learning collectively. And you and I spoke about it earlier as well. This topic has a very fine line between what is super cool. In my case, it's what's super cool for me to provide the customer versus when am I stepping over the boundary of being super creepy, right?
16:41
I think we also have to learn into where is our level of tolerance and acceptance between personalization and feeling more stockerish. Yeah, it'll be a Black Mirror episode. Yeah. Well, thank you so much, Helene.
16:57
It was great chatting with you. Thank you for having me.