Supply Chain and Logistics Considerations During and After COVID-19
Background
The impact of the COVID-19 pandemic spans countries, companies and organizations that have had to adapt to circumstances they have never had to face before. With each passing week the economic data is becoming more dire, markets shift and jobless claims continue to grow. One of the first and most commonly discussed areas has been around fiscal and monetary response and the credit industry. However, supply chains everywhere have been absolutely shaken for a completely different reason. The inability to congregate in groups, along with factories being shut down, retail stores being closed, increased demands for certain items, and business modeling shifting and changing, are creating profound impacts on supply chains everywhere.
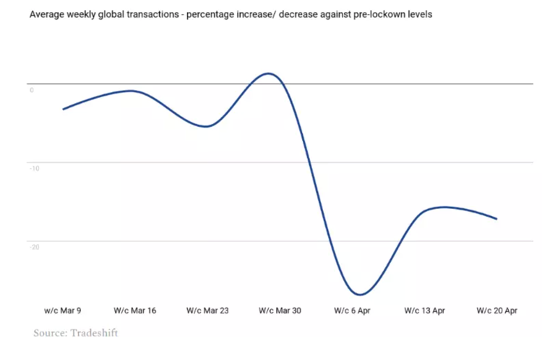
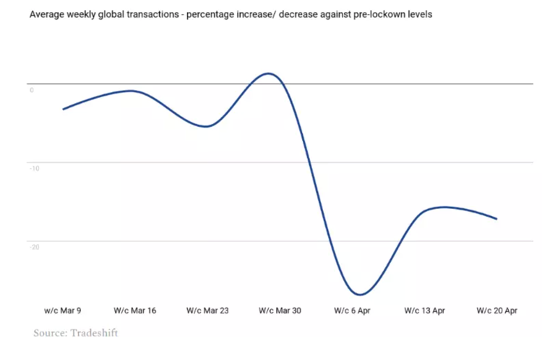
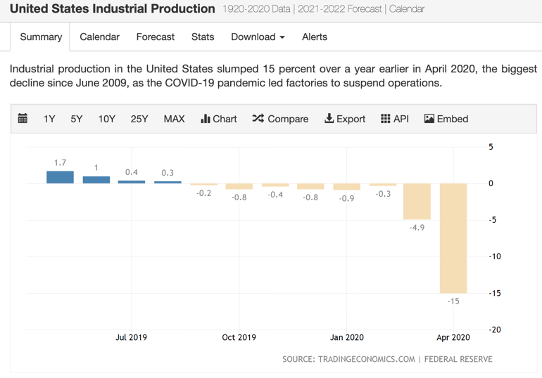
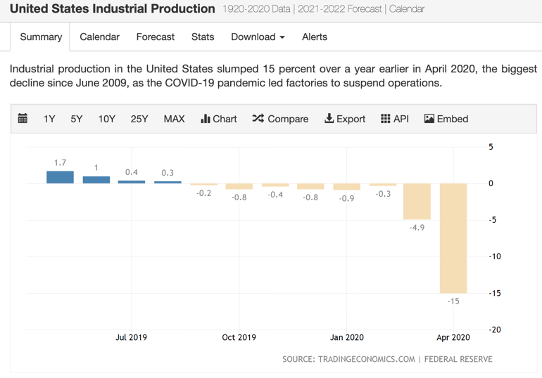
What started as a supply shock as consumers raced to purchase items and partake in activities before shelter in place advisory were to come into effect, quickly inverted into a demand shock as quarantines took place and consumers not only couldn’t spend money, but opted for liquidity in light of the economic uncertainty.
“The IHS Markit US Manufacturing PMI was revised lower to 36.1…, compared to March’s final 48.5. The latest reading was the lowest since early 2009, as output, new orders and exports all fell at record rates due to measures implemented to contain the COVID-19 outbreak. At the same time, employment declined the most since March 2009 amid greater spare capacity, as backlogs of work decreased at the most marked rate for 11 years.”
Put simply, given the uncertainty brought about by COVID-19, demand for imports and exports are down significantly and as a result those who would fulfill these orders are largely unable to work.
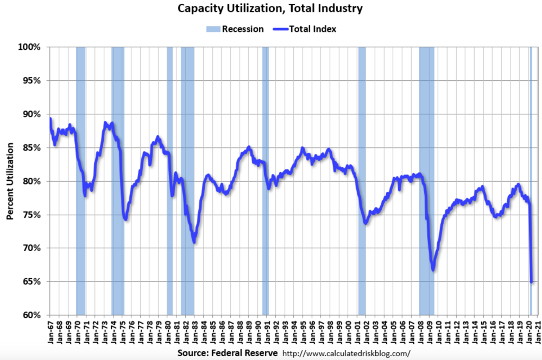
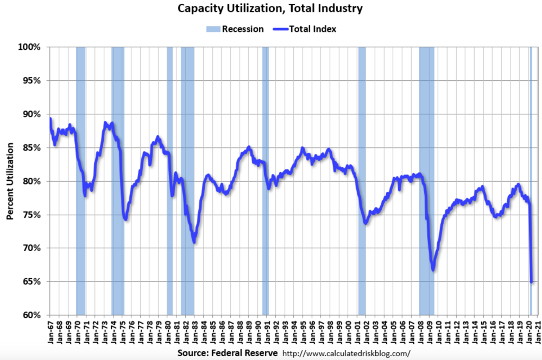
This gets especially concerning considering all the industries involved and the trickle down effect. Consider the food and agriculture industries wherein their products are perishable. These demand shocks can ultimately lead to having to write-off these products entirely, because there is no market for them. This especially ripples through the various brick and mortar grocery and food stores that have had to be closed due to quarantine protocol. Many of the largest grocery chains do not have an efficient delivery mechanism in place compared to the Amazon Fresh type options that have grown in popularity recently.
Historical Context
COVID-19 is an extremely unique challenge the world is facing for many reasons. First, all of our most recent crashes and crises have been economic or financial in nature. In the past, even with stocks reaching decade lows or unemployment beginning to spike, industries like retail, manufacturing, and industrial were still able to operate seamlessly. People knew that they could still go to the store and buy groceries or go to a car dealership and purchase a vehicle. As a result of this being an extremely contagious and dangerous pandemic, quarantine has limited the amount of businesses consumers can even interact with. Additionally, there is little to no data to know whether or not consumers will even truly feel comfortable returning to big open shopping places, should they reopen. As of May 2020, over 35 Million jobless claims had been filed in the United States alone, this has a dramatic impact on what the consumption demand will be and subsequently, supply chains.
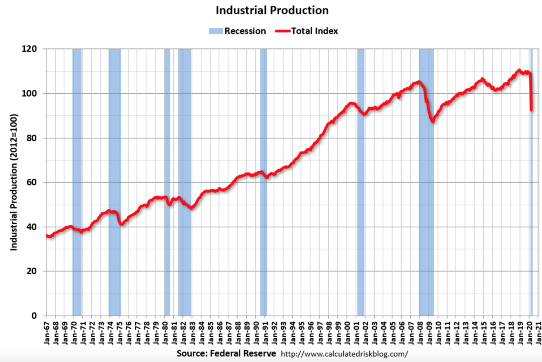
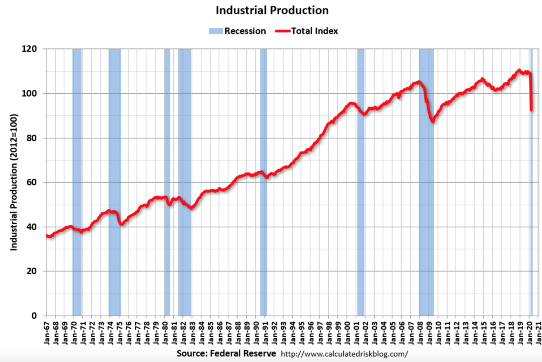
What Al Can Do?
H20.ai aims to solve our biggest challenges through Al, and COVID-19 supply chain and logistics related issues are no different. What many companies with deep supply chains are learning is that a black swan event like a global pandemic requiring offices and retail stores to be shut down was not built into their models, and it shouldn’t necessarily be. What we are learning however, is that the business environment may never be the same and companies need to prepare for the long haul and start to figure out innovative methods of adapting their businesses to a changing world.
Companies with deep supply chain dependencies or are supply chain centric, should look to apply Artificial Intelligence to improve the accuracy of predictions, increase the speed to responsiveness to changes in the business environment, and sense large trend shifts early.
Demand Optimization (Supply/Demand Forecasting):
What might have been relatively standardized and straightforward in the past, has been turned on its head with the pandemic environment. Put simply, companies look to accurately predict what the market demand for a product will be, what their production capacity can be for this product, and what is the optimal mixture of the two.
Inventory Management (Turnover and Wastage):
How quickly does a company sell and turnover their inventory that they have developed? What is the liquidation process flow of their inventory look? Is their inventory perishable or does it become defective over time?
Network Optimization Models:
As global supply chains have become more robust & intertwined with massive networks of trading partners, trade routes, and transportation methods, solving “optimal route” has become significantly more complex. Companies had recently become focused on the most environmentally/ fastest/cost effective distribution methods, but now with COVID-19, companies are having to readapt to determine what options for shipping and transport even exist.
Fulfillment:
Companies heavily dependent on delivery and shipping products are increasingly focused on how effectively they can quantify and understand how well they are able to fulfill the orders for their products. Did they sell out? If they didn’t sell out, why did they fail to fulfill? Was it a technology error or a distribution error?
Demand Optimization:
An example that H2O pursued was demand optimization under COVID constraints. By applying real-time data, external data such as COVID statistics, and unstructured data such as mobility data, to build more robust demand sensing models in order for firms to adapt to rapidly changing business environments.
Catastrophe Modeling:
It has become very clear that most companies did not have an extremely contagious virus scenario model built, but it has also brought to light many companies were overly ambitious in how well they could predict various scenarios. More advanced machine learning models need to be applied to run more iterations and cross validations of predictions to try optimize future operating models to be more stable during black swan type events such as a pandemic.
Advanced Network Analysis:
Due to the constraints with manufacturing, travel, and other pandemic related operating constraints, it is more important now than ever for firms to apply the most leading edge methodologies to look for creative solutions to optimize network strategies.
Inventory Optimization:
Because we are currently witnessing global demand shocks and a supply shock in many different categories of products, it is more challenging than ever to figure out inventory management. Companies should look to deploy Artificial Intelligence to apply the most advanced methods for minimizing Inventory wastage, while rapidly adapting and learning to adapt to fast moving COVID conditions.
Augment and Enrich Data:
Platforms like H2O Q and Driverless Al enable seamless transformation and augmentation of data sets. It can be extremely valuable to be able to attach external mobility data, traffic data, travel data, 3rd party commodities futures data, or Google trends to predictive models.
How Al and Machine Learning Can Help
H20.ai has developed a new set of AI apps by combining the H2O Q and Driverless Al platforms together to bring automated insights and automated machine learning to sets of business problems. H20.ai created a new Q Al Demand Sensing App to address the challenges in the supply chain and logistics space. Global supply chains are notoriously intertwined and therefore an external shock sends a ripple effect through that market, therefore it is extremely important supply chain practitioners make the best predictions possible.
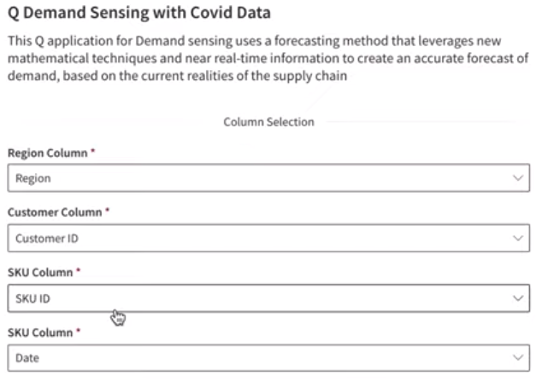
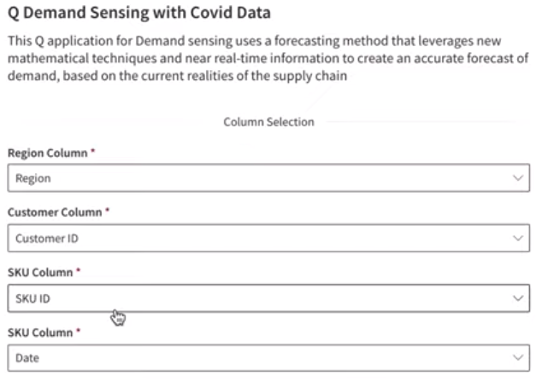
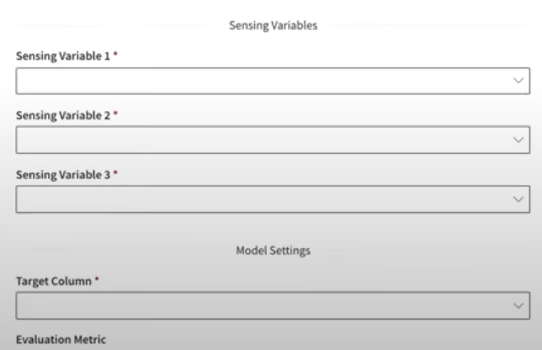
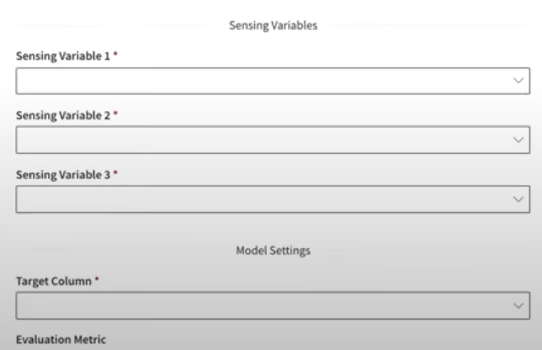
We can see an example of the external data such as COVID data or Twitter sentiment analysis that we can bring in and easily append to our data set to analyze correlation and potentially predictive signal.
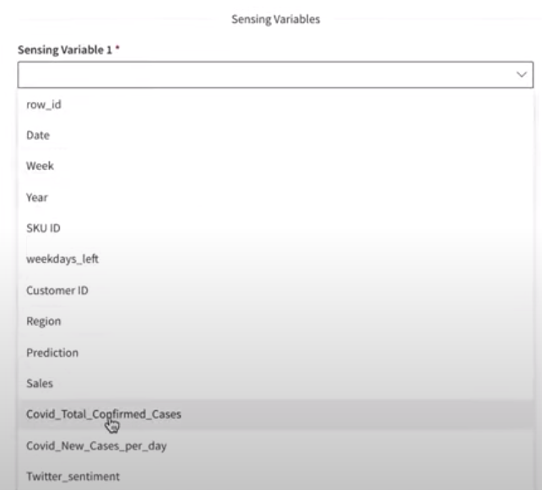
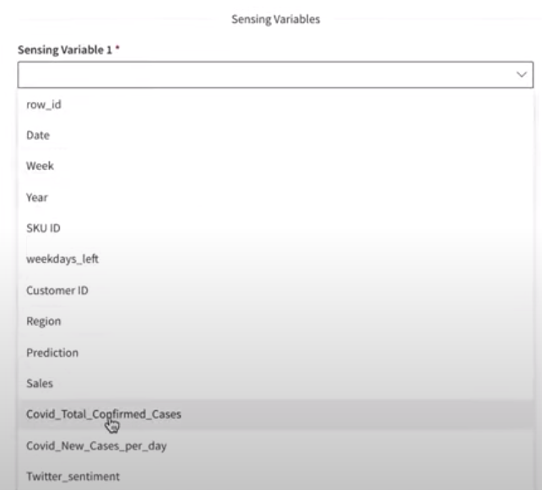
From there, it is very easy to select all of the various combinations of SKUs and regions to analyze and model.
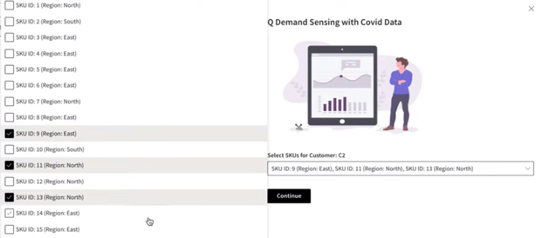
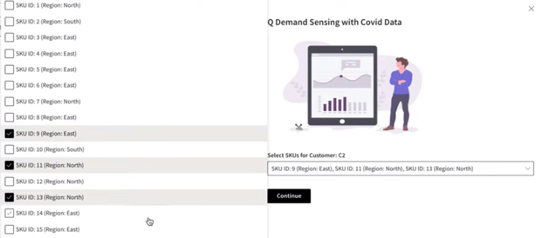
Q Demand Sensing highlights that this is a time-series model and how this will be constructed while it runs.
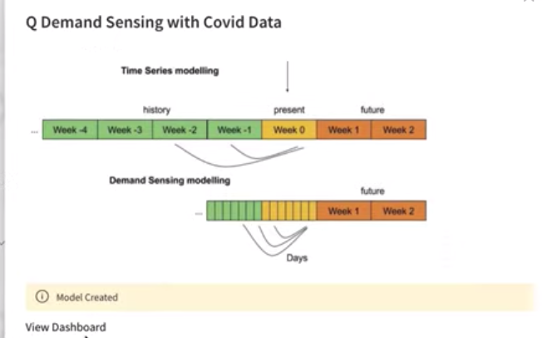
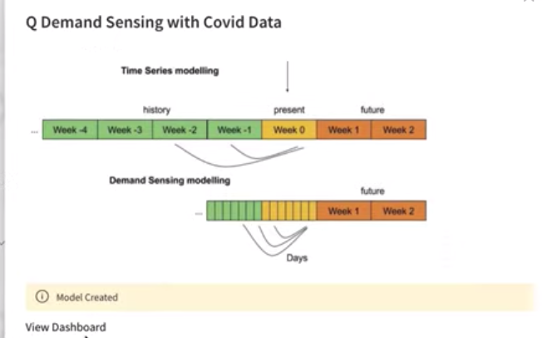
Once the experiment is completed, we can see upfront how much lift and insight we were able to drive in our model with our external data and advanced model methodologies.
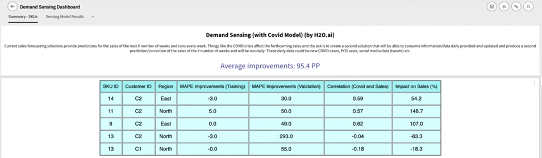
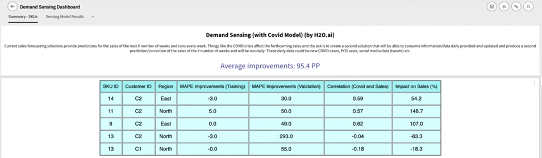
We can click through and see a time series chart of predicted versus actual overlaid over our external variables and factors of interest.
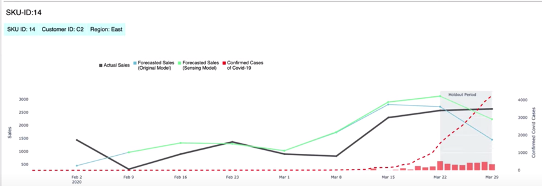
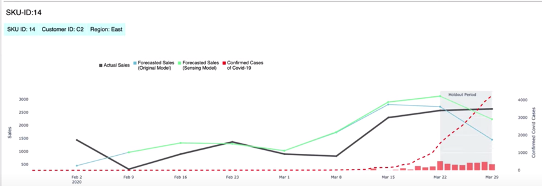
We can also see additional information by product below in the holdout versus training sets, so we can get a better understanding of how we believe the demand sensing predictive model will perform out of sample.
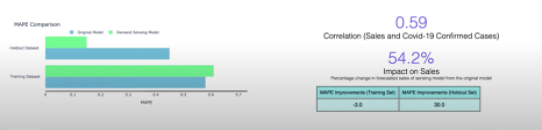
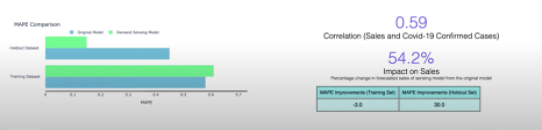
Conclusion
Supply chain dependent businesses face an additional burden when faced with COVID-19 challenges. They deal in physical products that typically require large volumes of people from the manufacturing phase all the way to the delivery phase and even deal with the issue of expiration of products. In order for these companies to survive, it is imperative that they adapt through adoption of technological innovations such as Al at their disposal in order to build faster, more efficient, agile, and resilient businesses. It is precisely this reason that H20.ai developed the Demand Sensing application alongside our supply chain & retail partners to become more responsive to events like COVID-19.