H2O Driverless AI
Democratizing AI with Automated Machine Learning
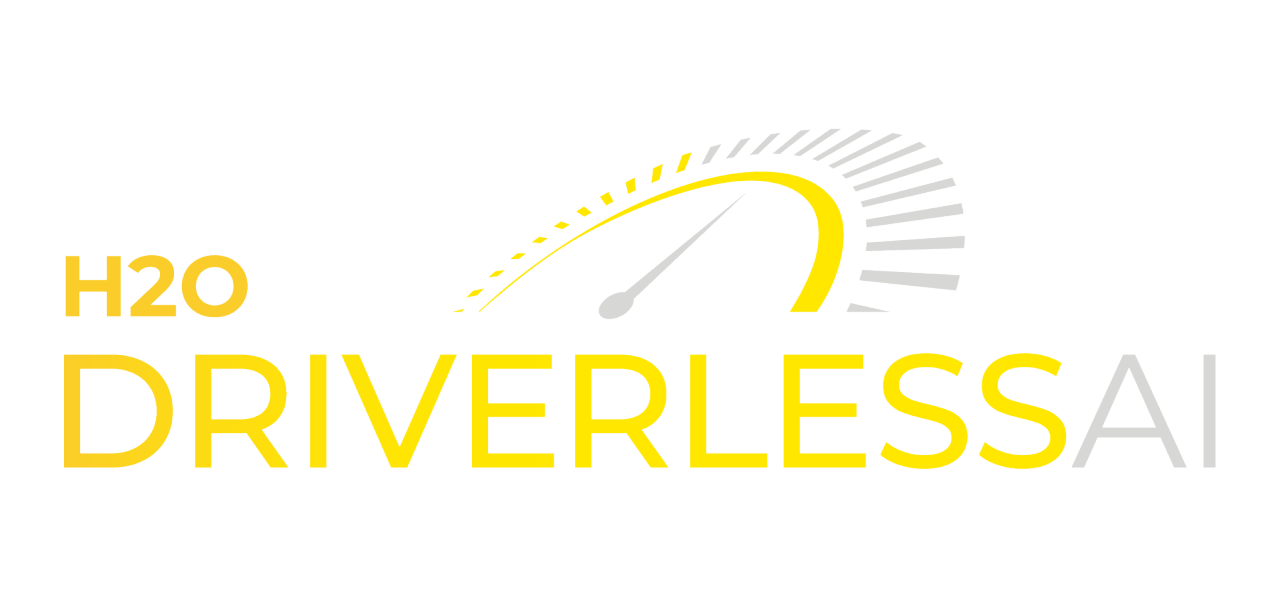
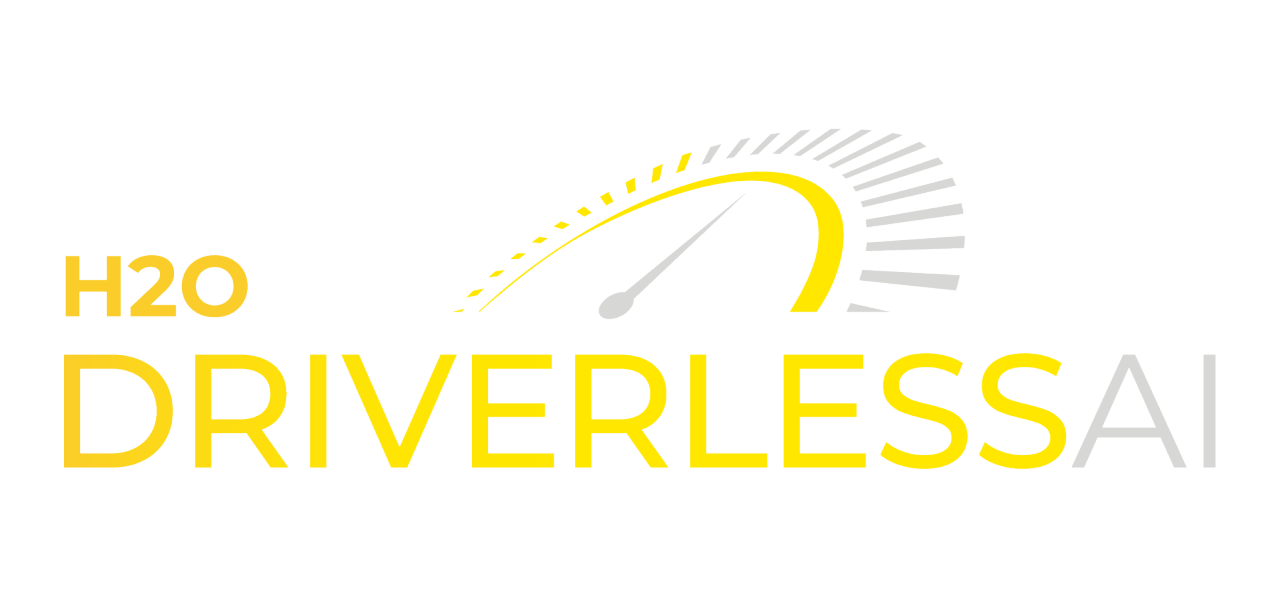
Hear directly from our customers.
Rajesh Malla, Head of Data Engineering at Resolution Life insurance, takes the stage at H2O World Sydney 2022 to discuss AI transformation and how Resolution Life uses H2O Driverless AI to predict claim triage and other insurance AI use cases.
What is Automated Machine Learning?
Machine learning is the foundational element of artificial intelligence (AI) and uses algorithms to detect and extract patterns in data to predict certain outcomes based on that analysis.
Automated machine learning (autoML) systematically addresses multiple steps of the data science lifecycle with automation designed to reduce complexity across tasks and empower data scientists to implement AI projects with higher accuracy more efficiently. AutoML also improves accessibility to machine learning capabilities for those without expertise in data science by providing user-friendly interfaces that anyone with beginner technical knowledge can use, enabling business users and IT professionals to easily implement machine learning into their daily workflows.
H2O Driverless AI
Delivers industry leading autoML capabilities specifically designed to use AI to make AI, with automation encompassing data science best practices across key functional areas like data visualization, feature engineering, model development and validation, model documentation, machine learning interpretability and more.
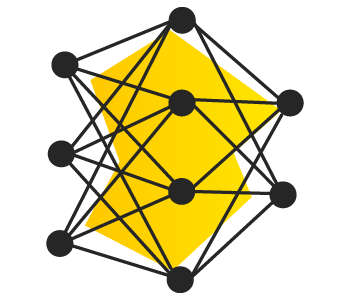
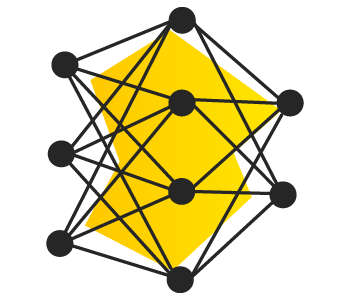
Intelligent feature transformation
H2O Driverless AI automates the entire feature engineering process to include detecting relevant features in a given dataset, finding the interactions within those features, handling missing values, deriving new features from data, comparing the existing and the newly generated features and showing the relative importance of each of these features. Features are transformed into meaningful values that machine learning algorithms can easily consume.
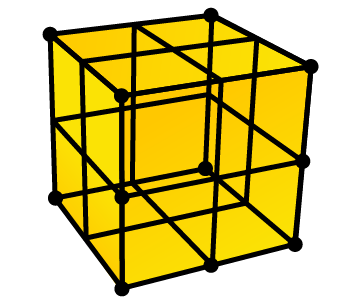
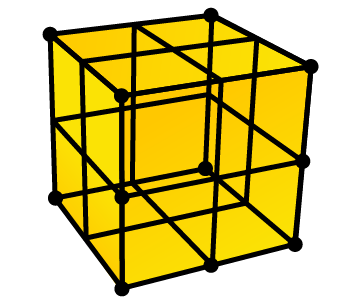
Automated model development
Reducing the time that it takes to develop accurate, production-ready models is critical to delivering AI at scale. H2O Driverless AI automates time-consuming data science tasks including, advanced feature engineering, model selection, hyperparameter tuning, model stacking, and creates an easy to deploy, low latency scoring pipeline. With high-performance computing using both CPUs and GPUs, H2O Driverless AI compares thousands of combinations and iterations to find the best model in just minutes or hours.
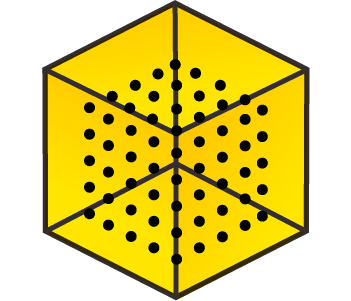
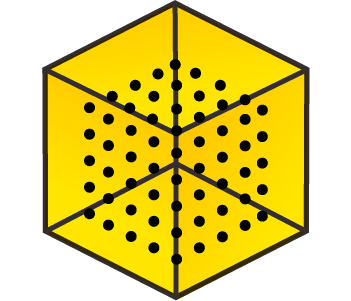
Comprehensive explainability toolkit
H2O Driverless AI provides robust interpretability of machine learning models to explain AI results. With industry-leading capabilities for understanding, debugging and sharing model results to include Machine Learning Interpretability (MLI) and fairness dashboards, automated model documentation and reason codes for each model prediction, H2O Driverless AI provides data teams with everything needed to provide transparency and establish trust across the entire machine learning lifecycle.
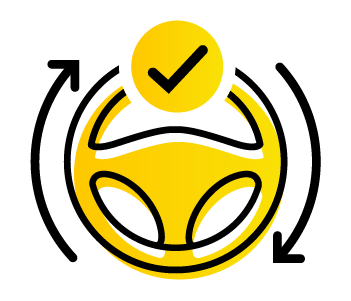
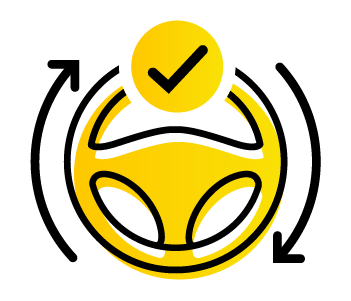
Expert recommender system
H2O Driverless AI uses an AI Wizard that investigates your data, provides recommendations based on your business requirements and gives instructions on the appropriate machine learning techniques to select based on your unique data and use case requirements. The AI Wizard’s built-in recommendations are based on data science best practices from a variety of disciplines to ensure the customized model being created leverages your data effectively and aligns with your business needs.
Everyone can benefit from the power of artificial intelligence, but only those with certain skill sets have the capacity to build and develop solutions with the machine learning tools available in today’s technology market. However, it is critical for people in a variety of disciplines to create and use machine learning applications as AI becomes more pervasive across all organizational functions. Automated machine learning has removed barriers to AI adoption by wrapping the expertise needed to build models into a guided approach to data science that allows users to access the power of machine learning without the need to write any code.
How It Works
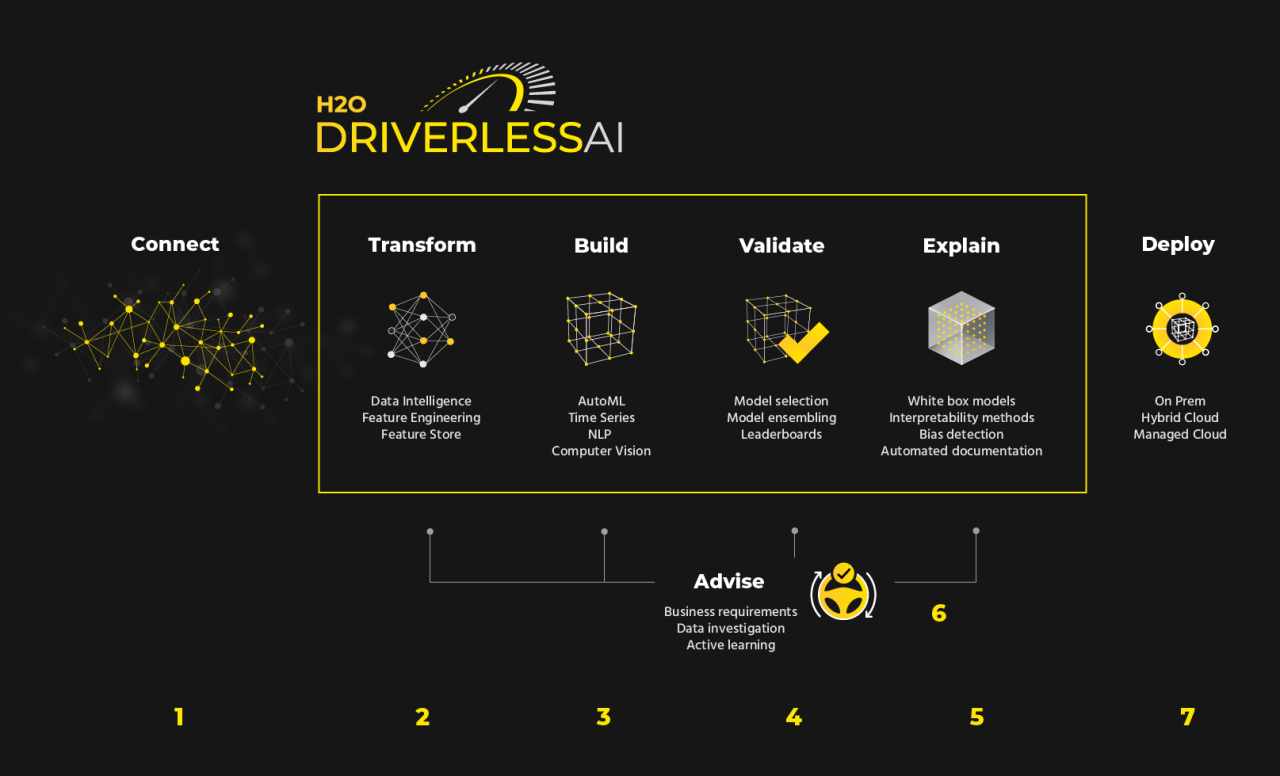
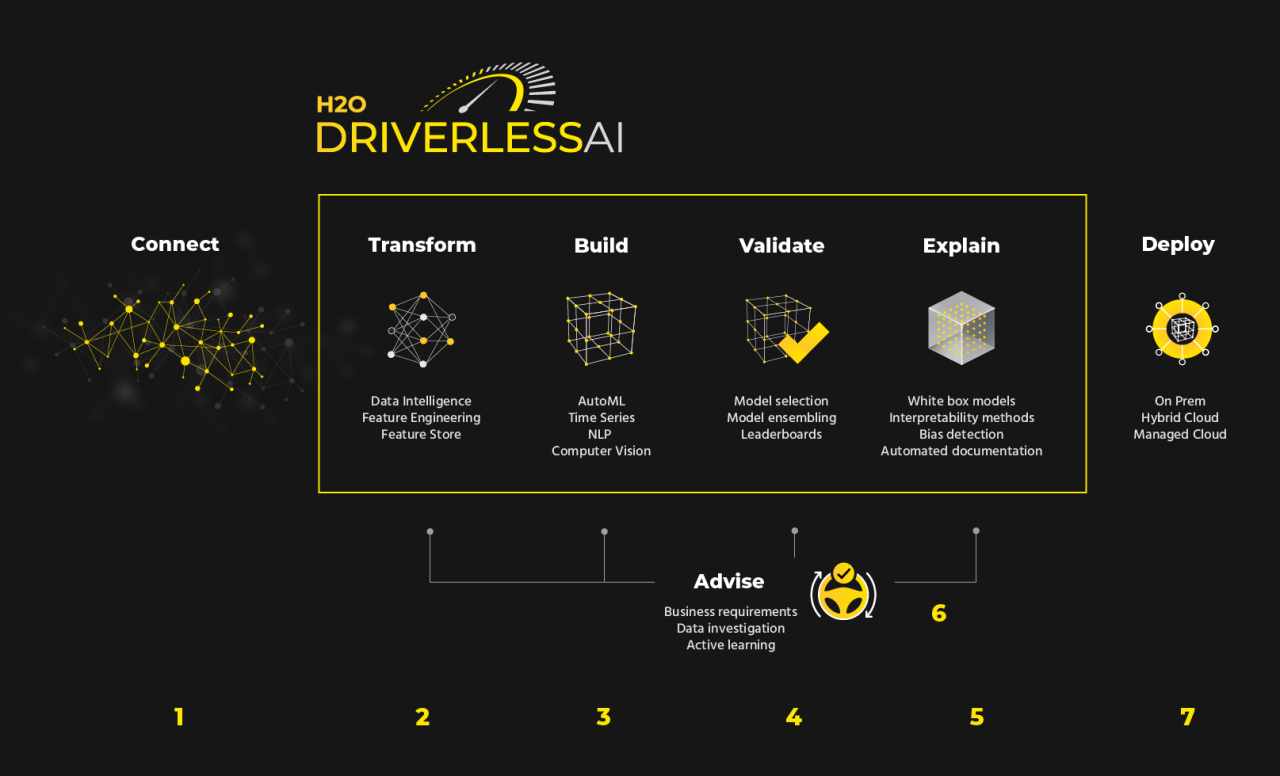
Automatically visualize and address data quality issues with advanced feature engineering that transforms your data into an optimal modeling dataset.
Quickly create and test highly accurate and robust models with state-of-the-art automated machine learning that spans the entire data science lifecycle and can process a variety of data types within a single dataset.
Assess model robustness and mitigate risks in production by obtaining a holistic view of the models and preventing failures on new data.
Easily understand the ‘why’ behind model predictions to build better models and provide explanations of model output at a global level (across a set of predictions) or at a local level (for an individual prediction).
Create and customize machine learning models with a built-in guidance system designed to implement data science best practices based on your unique dataset and use case requirements.
With H2O Driverless AI, models can be deployed automatically across a number of environment choices including creating a REST endpoint for any web applications to invoke the model, automatically run as a service in the cloud, or simply as a highly optimized Java code for edge devices.
Democratizing AI Adoption with AutoML
As organizations look to streamline decision making and improve customer experiences with AI, they are running into three core challenges of talent, time, and trust. There is not enough data science talent to build every use case by hand, and even with the right people, hand-coding is time intensive, difficult to replicate and subject to many errors. Each model developed must then be explained and validated by the business so users can trust the decisions provided by the model. H2O Driverless AI automates many core data science tasks, while automatically documenting the model development process and providing machine learning interpretability for all models in production. With the addition of an AI Wizard that provides proactive data science guidance, more people across all business functions can now build machine learning models, removing barriers to machine learning literacy and understanding and, ultimately, increasing accessibility to AI technologies.
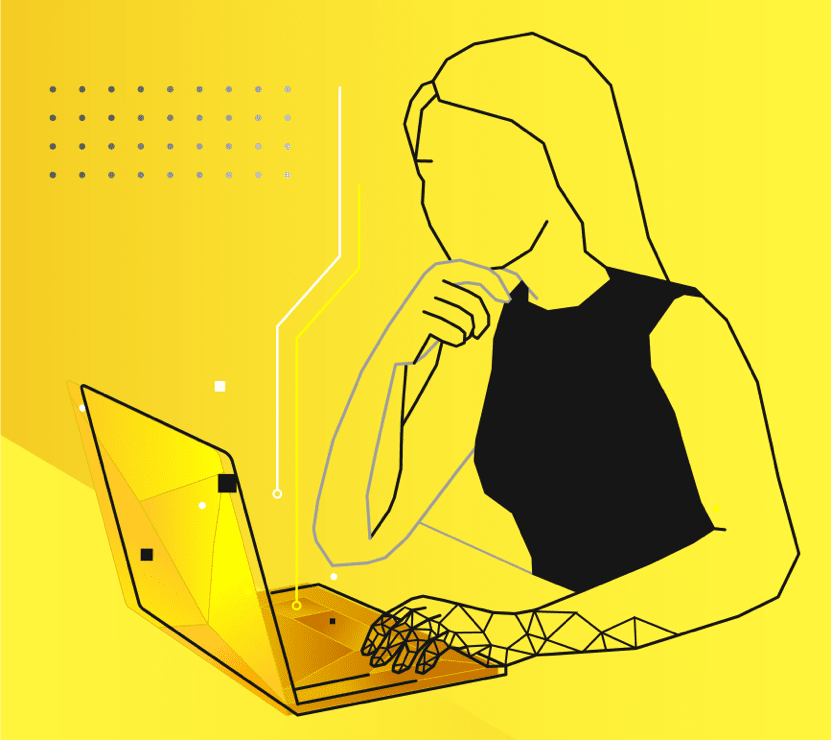
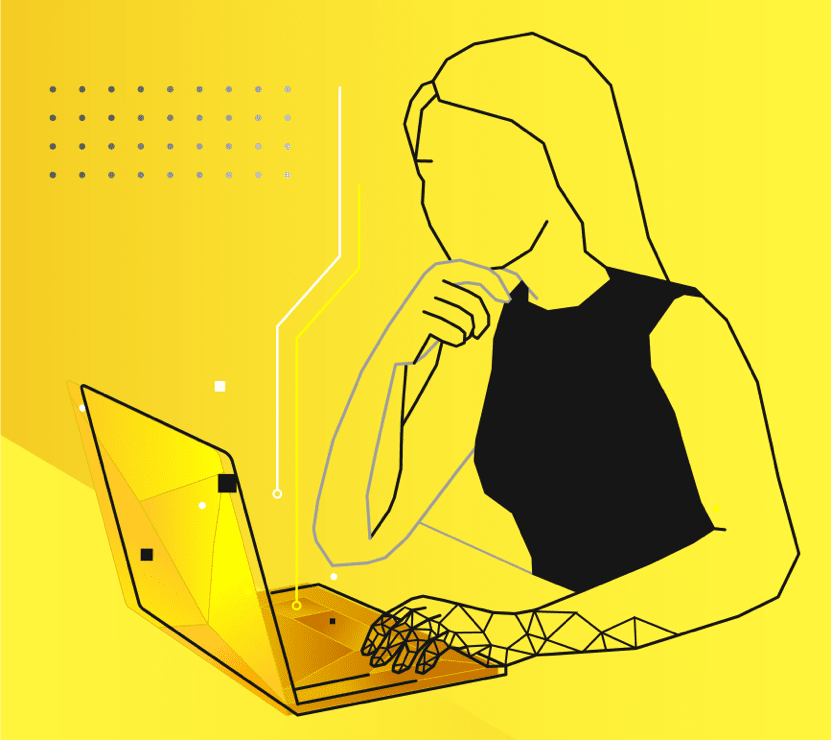
Data Scientists
Make highly accurate machine learning models with speed and transparency to accelerate the implementation of analytic initiatives.
H2O Driverless AI empowers data scientists to work on projects faster and more efficiently by using automation to accomplish key machine learning tasks in just minutes or hours, not months. By delivering automatic feature engineering, model validation, model tuning, model selection and deployment, machine learning interpretability, bring your own recipe, time-series and automatic pipeline generation for model scoring, H2O Driverless AI provides companies with an extensible customizable data science platform that addresses the needs of a variety of use cases for every enterprise in every industry.
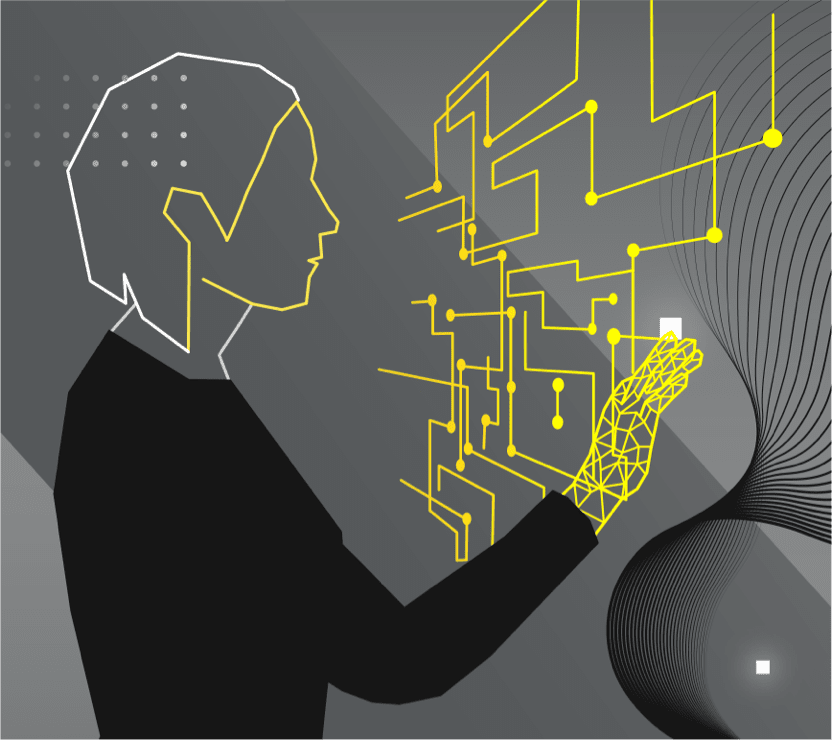
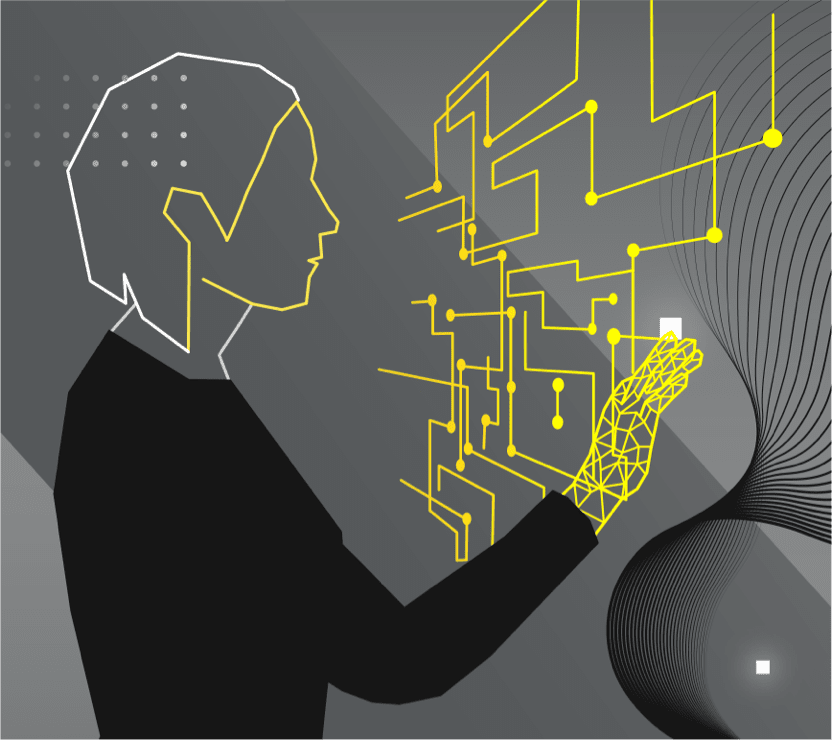
DevOps and IT Professionals
Operationalize models with minimal changes to existing workflows.
With H2O Driverless AI, machine learning models can be deployed automatically across a number of environment choices including creating a REST endpoint for any web applications to invoke the model, automatically run as a service in the cloud, or simply as a highly optimized Java code for edge devices. It is optimized to work with the latest Nvidia GPUs, IBM Power 9 and Intel x86 CPUs and to take advantage of GPU acceleration to achieve up to 30X speedups for automated machine learning. H2O Driverless AI includes support for GPU accelerated algorithms like XGBoost, TensorFlow, LightGBM GLM, and more.
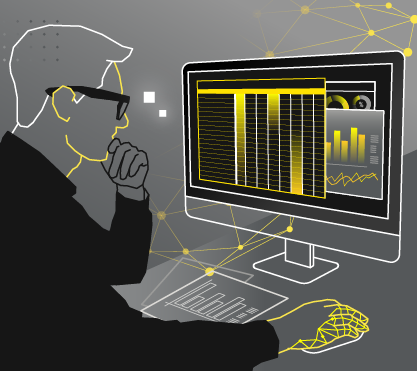
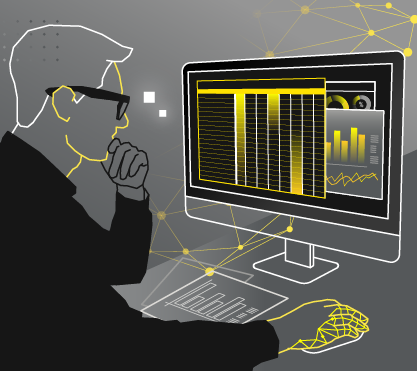
Business Analysts
Driverless AI users can take advantage of the flexibility it offers in the feature engineering process via built-in recipes, an open catalog of recipes and by using BYO functionality.
H2O Driverless AI allows almost everyone within a business to create their own predictive models. The quality of models generally depends on the skills and experience of data scientists involved. Once the user defines a target column, Driverless AI will automatically identify the appropriate features, select and parameterize the optimal algorithm, present the results in chart format and enable the user to automatically deploy the new model to production.
Driverless AI in Action
I am very excited to use the H2O Driverless AI because prior to it, we used to spend weeks hyperparameter tuning etc., but with Driverless AI, one experiment takes just a few hours.”
Wei Shao, Data Scientist, Hortifrut
AI to do AI is absolutely a watershed moment in our industry.”
Martin Stein, Chief Product Officer, G5
The automation of the data science process reduced time and costs. And time is money. So, you can do more with the same amount of time. It's possible to deliver more value to the business, develop more use cases and focus the data science effort in the use case instead of development tasks.”
Ruben Diaz, Data Scientist, Vision Banco, Vision Banco
Request a demo to learn how H2O Driverless AI can help democratize AI across your organization.